How Hierarchies Enable Proper Customer Data Management

Not only do hierarchies represent data in a structured and understandable way, but they also serve practical business purposes, serving both operational and analytic value.
For B2B businesses, understanding the hierarchical nature of customer relationships is essential. While a typical B2C company has more customers than a B2B company, the customer-to-account relationship is one-to-one, making managing customer data fairly simple. Conversely, a ‘single’ customer in a B2B scenario can be incredibly complex; customers are composed of many locations, divisions, and roll-up to parent companies and subsidiaries.
For instance, the legal entity you contract with may differ from the regional office you deliver to, or the parent group representing the overall corporate relationship. Consider the following case: when Microsoft acquired LinkedIn, Microsoft Corporation became the parent company to both LinkedIn and its subsidiaries. LinkedIn suddenly had connections to Microsoft’s other subsidiaries, such as Github and Skype. Add to the changes brought on by the acquisition, LinkedIn has many locations: LinkedIn Ireland and LinkedIn Singapore are both a part of LinkedIn Corporation. When a business has multiple entities as customers, it can be confusing to determine what services and what contracts refer to which customer.
Customer relationships fail when customers are only defined as individual entities. This limits both the operational and analytical decision-making available through data. However, by utilizing hierarchies for customer data, B2B companies can more clearly define their customers and better inform decisions related to sales coverage, account expansion, contract management, and risk assessment. Understanding the different types of hierarchies, the challenges in managing them, and the value propositions will explain their potential role in your business.
Types of Hierarchies
B2B customer data can be expressed or shown through many different forms of hierarchies. The type of hierarchy is chosen based on the business’ needs, and often multiple types are required to fully support the business – how sales classifies customers for regional territory management might not be the same as how finance defines customer hierarchies for legal contract management.
Two different types of hierarchies in the context of B2B customer data are given below:
Corporate hierarchy: classification based on the relationship between entities within a corporation, showing parent/subsidiary relationships.
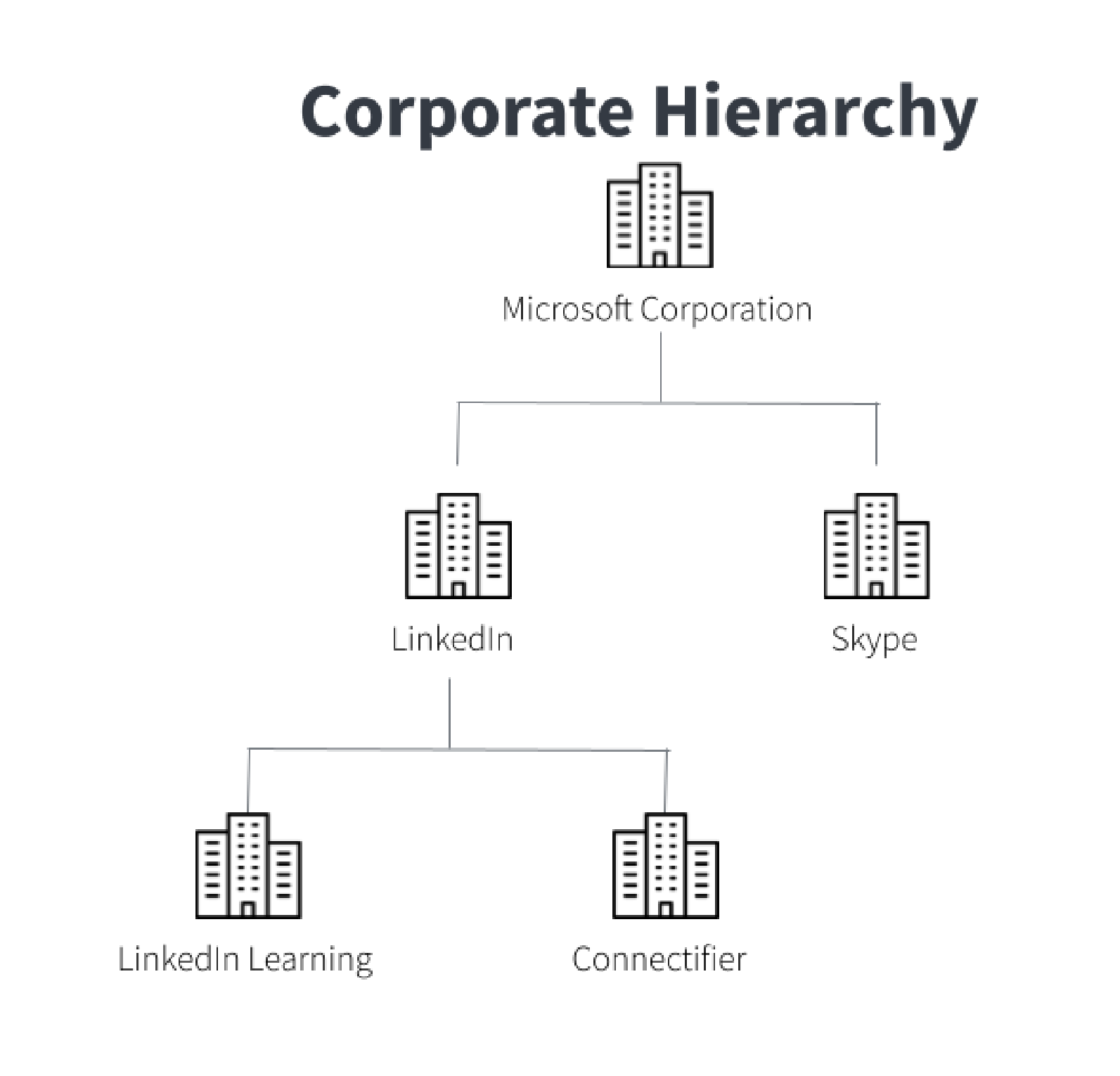
Sales hierarchy: classification based on site or region of branches under one corporation, possibly showing geographic relationships.
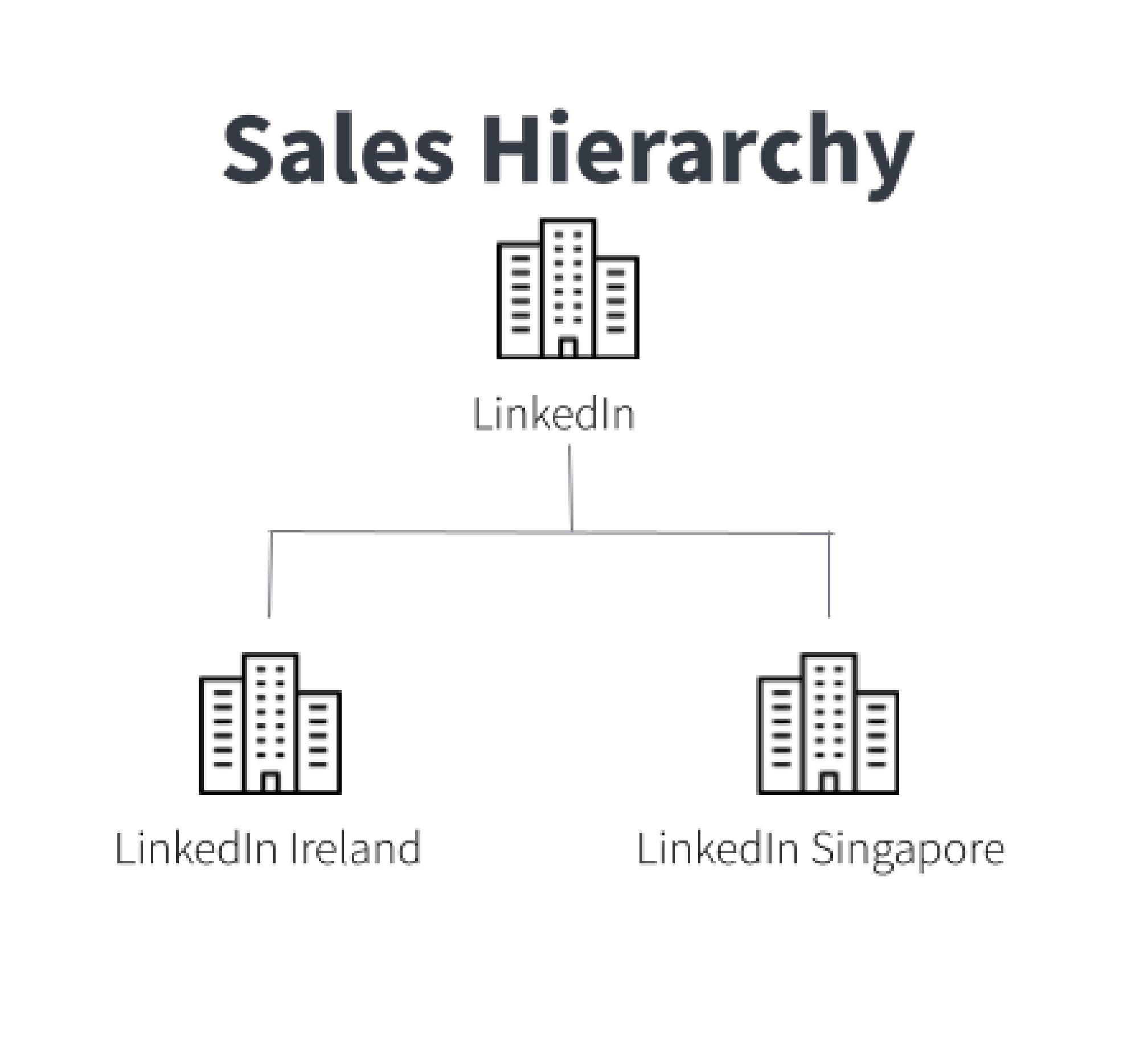
Challenges of Hierarchy Management
While hierarchies may seem like a simple way to organize B2B customer information, maintaining and overseeing hierarchies are difficult for a number several
1. Duplicate, siloed, or misentered data: Duplicate, siloed, and misentered customer data limits the effectiveness of hierarchies. The problems of this type of bad data snowball- hierarchies based on this data will also contain duplicate and incorrect information, which limits the ability to structure the data. It is important that hierarchies contain mastered data, which will improve accuracy and completeness to use and manage these hierarchies effectively.
2. Missing, incomplete, or outdated data: When customer information is missing, incomplete, or outdated, it also limits the effectiveness of hierarchies. If fields such as legal entity or address are obsolete or missing, this type of bad data might lead to two entities or branches being treated as separate when they are really the same. Additionally, missing contact information such as phone numbers or addresses prevents identified sales opportunities from being explored. To use customer data in hierarchies and maximize their effectiveness, the customer data needs to be enriched with updated and complete information, standardized and verified using trustworthy external sources.
3. Changing corporate relationships: For various business reasons, most commonly when mergers and acquisitions take place, company names may change, in addition to the corporate relationships. It can be difficult to keep up with this constantly shifting corporate information and make-up. Hierarchies can quickly become inaccurate or obsolete, as the name of a customer, and its subsidiaries, parent, or locations change. In order to fully utilize hierarchies, they need to be updated with the current corporate structures.
Mastering Customer Data for Hierarchies
Master data management can help ensure a robust approach to defining, implementing, and governing hierarchies. Key features of a modern MDM approach for hierarchies include:
1. Unique ID’s: Data clustering: [deduplication, ability to cluster at various levels, and creation of a single identifier] Knowing the fact that different types of hierarchies may address different business needs, clustering at different levels or based on different criteria is essential for generating functional and effective hierarchies. Additionally, unifying duplicate records and giving each unique record a single identifier allows for a single and complete source of data. Together, complete and flexible hierarchies are formed.
2. Machine learning with humans in the loop: [ability for SMEs from the business to decide what is and isn’t a match/ fits the hierarchy to ensure the data is in a usable format] Traditional rules-based matching is not efficient at scale and doesn’t always account for misentered, incorrect, or missing information. Modern MDM approaches include human-guided machine learning. Subject matter experts, or SME’s, that know and understand the data on a business-level, can give their input on what is and isn’t a match, or if something fits or doesn’t fit the hierarchy. This ensures the data is in a usable format. Then, machine learning can expand on this SME input and suggest matches, with high accuracy, to quickly and effectively master at scale. Thus, efficiently creating usable and trustworthy hierarchies.
3. Enrichment with external data sources: [ability to include data sources for accurate, up-to-date info and external guidance on hierarchy structure e.g. D&B] Using verified data sources while enriching records will provide complete and accurate information. For example, addresses and contact information can be updated, and hierarchy structures can be completed based on comparing against external sources such as D&B.
Value Proposition:
To maximize hierarchies’ value, it needs to contain mastered data, be flexible in their organization format, and include updated and enriched information. Assuming that all of these conditions are fulfilled, hierarchies can be extremely useful and advantageous for B2B businesses.
Hierarchies can help your business increase revenue and growth while minimizing risk. By putting your B2B customer data into a type of hierarchy and comparing certain fields, you can identify certain accounts to prioritize and contact to develop leads. Specifically, with the help of these hierarchies, you can discover more points of contact as well as which type of business you have with which entities. So, with this outreach and organization, your business can find cross-selling and up-selling opportunities. Corporate hierarchies can also help your company make these business decisions with reduced risk. Defining corporate relationships, as well as knowing complete account information, will mitigate any risky or poor business decisions.
Additionally, hierarchies can make the sales process, as a whole, more efficient. Read more about this in our ebook, “How Bad Customer Data is Killing B2B Sales.” Putting your B2B customer data into hierarchies allows you to understand corporate relationships based on entity, division, or site. Knowing this information can improve and standardize sales management within your own business. Specifically, hierarchies can enhance lead routing and assignment. This will limit confusion and allow for proper mapping. Also, hierarchies can improve reporting. New accounts are identified as a subsidiary or independent of other accounts. Together, these all allow your sales team to efficiently work with the proper information, reducing the length of sales cycles.
Conclusion:
As you can see, using customer hierarchies are critical to strong customer relationships. Hierarchies based on B2B customer data can help identify business opportunities and drive growth. At the very least, hierarchies can be used to visualize and organize account information. But, due to their allowance of flexible classifications and with mastered, updated, and enriched information, hierarchies can improve account management, facilitate sales cycles, and reveal specific selling opportunities.
Get a free, no-obligation 30-minute demo of Tamr.
Discover how our AI-native MDM solution can help you master your data with ease!