Schedule your
AI-native MDM demo.
Make your data better, so your business can work smarter
For more information, please view our Privacy Policy.
What can you expect?
Consult with an Expert: Engage with a specialist to discuss your unique data challenges and requirements.
Tailored Demo: Experience a personalized demonstration of Tamr's data solutions, focusing on your specific needs.
Expert Feedback: Gain insights and recommendations on achieving clean, connected, and enriched data records.
No Commitment: Explore Tamr's capabilities without any obligation.
Trusted by data teams to deliver accurate, reliable data at scale to your business
"Everything revolves around data, so not having a solid data infrastructure is a non-starter. Without it, you can’t compete, you can’t understand your customers and how they use your products."
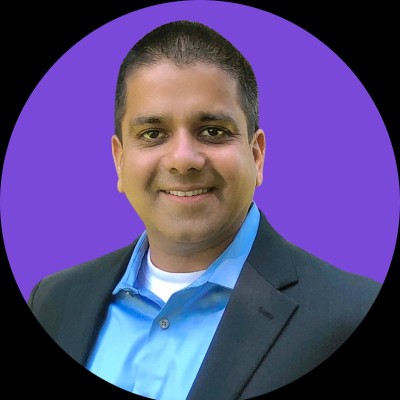
Anupam Nandwana
Chief Executive Officer
“By achieving a unified view of 7.5M providers, we are able to improve staffing strategies and reduce provider burnout by better balancing physicians’ patient loads.”
“With a holistic and accurate view of each customer, we can offer more personalized, efficient, and responsive services that enhance customer satisfaction and loyalty.”
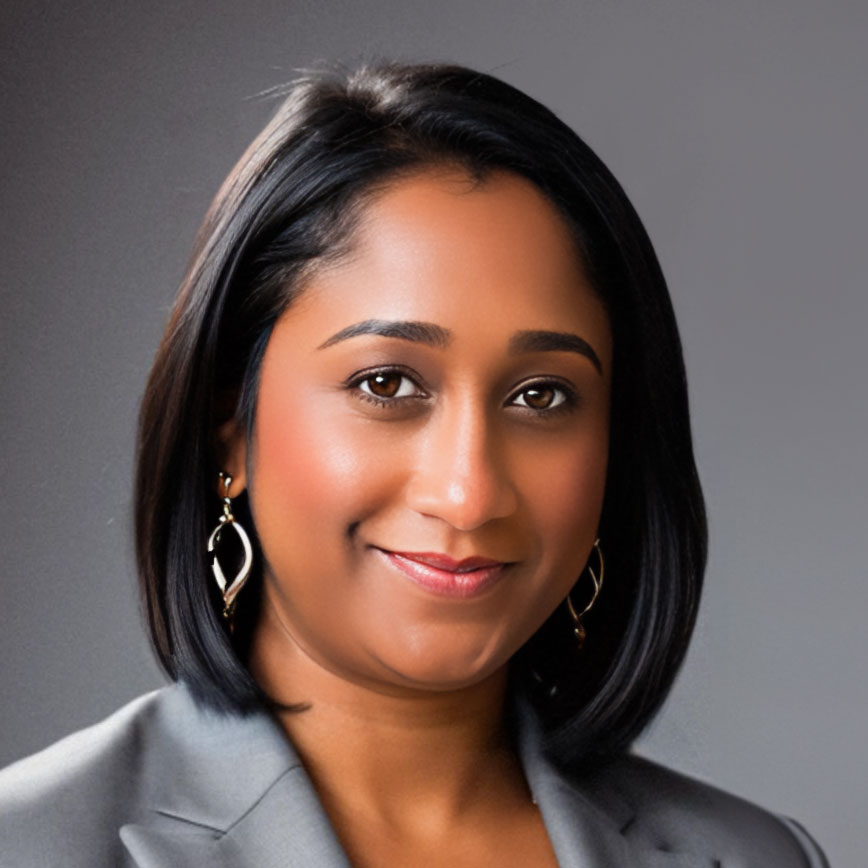
May Govender
Chief Information Officer
“I’ve been impressed by not only the caliber of the human-guided machine learning that rapidly scaled our analytical operations, but also by the professionalism and expertise of the field engineering teams of Tamr."
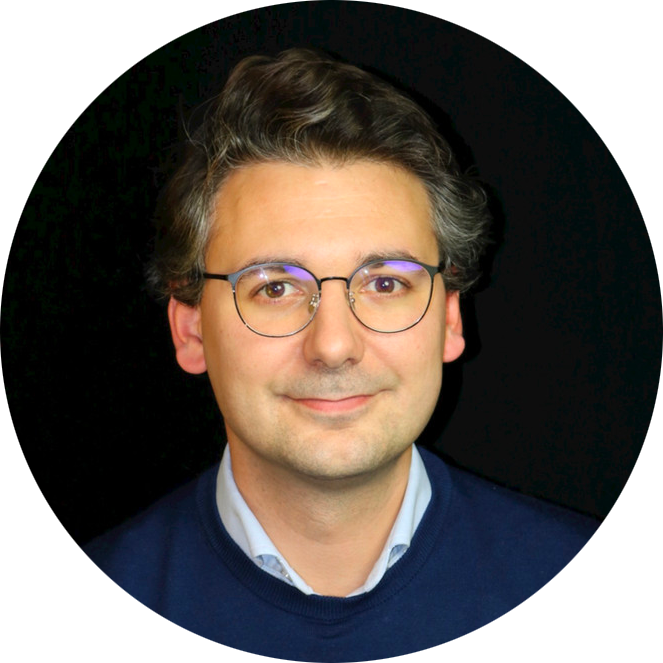
Jean-Baptiste Anne
Head of Sourcing Methods & Information Systems
“Data mastered by Tamr underpins the entire digital journey. If we didn't have the single customer view, we wouldn't be able to feed information downstream into decision and risk engines.”
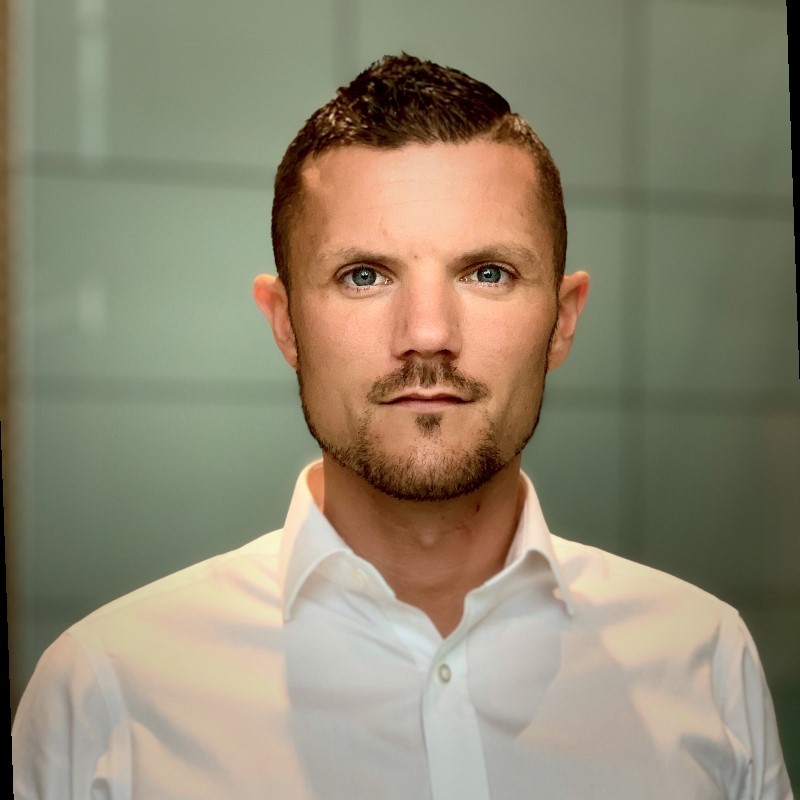
Jonathan Holman
Head of Digital Transformation
"To master 375 million records using the traditional way of writing rules, we'd be doing it for 10 years and probably still wouldn't be able to do it."
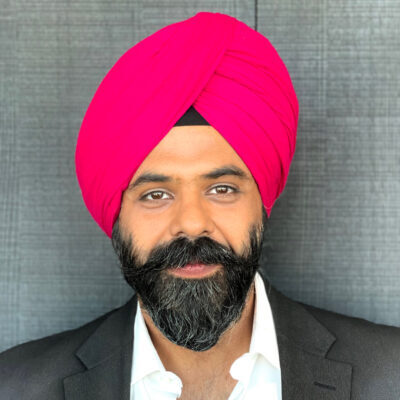
Harveer Singh
Chief Data Architect