Manage Data Quality with Data Mastering
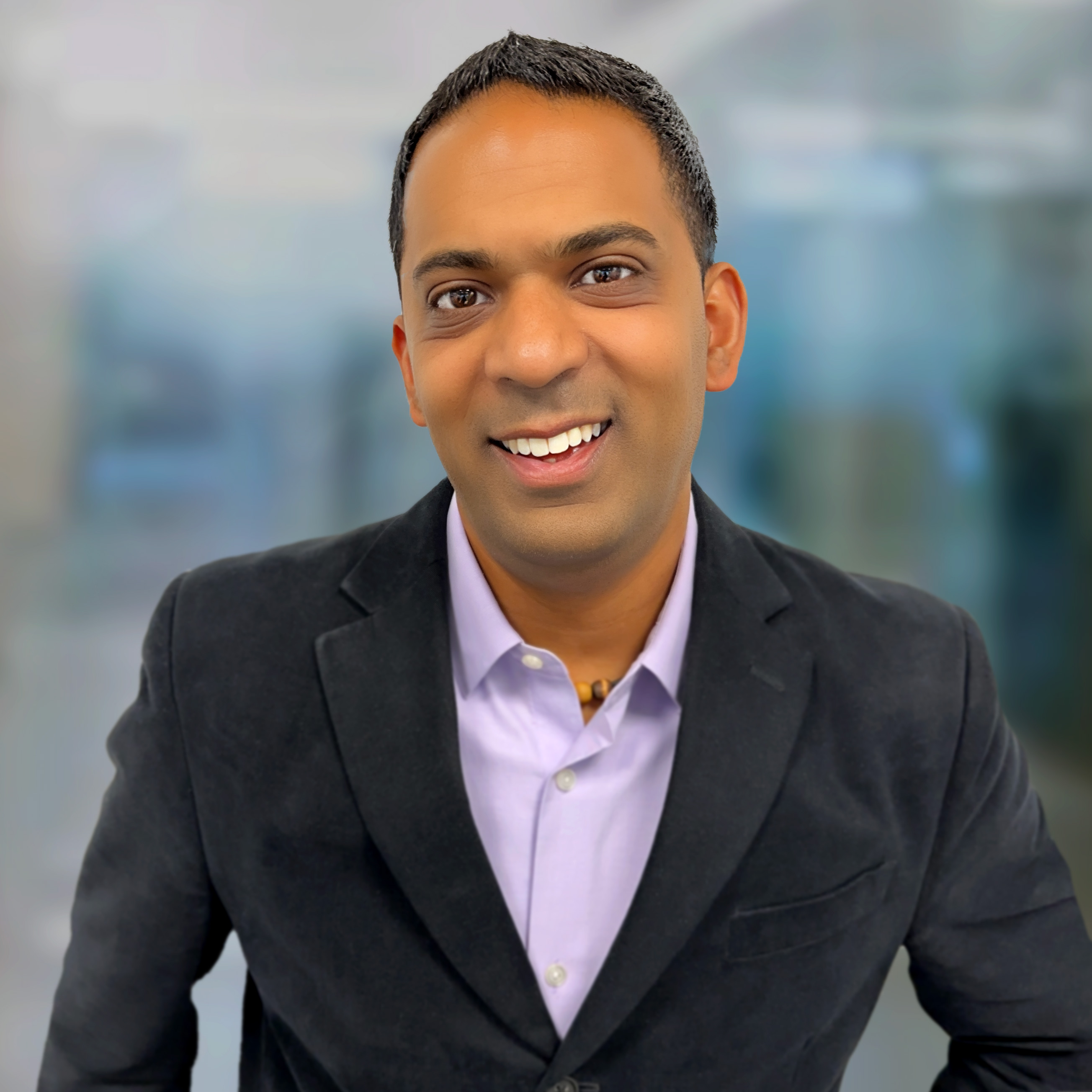
Becoming data-driven is no longer an option if companies want to grow and succeed. It’s an imperative. But despite years, even decades, spent aiming to achieve this goal, many organizations continue to struggle.
With investments in digital transformation set to top $7.4 trillion by 2023, it’s clear that organizations are committed to achieving this goal. So then why are so many falling short?
The heavy reality is that modern, enterprise data is highly complex. The average G2000 company has over 1,000 data sources across more than 600,000 tables. Data flows from a myriad of sources, including multiple ERPs, CRMs, and departmental SaaS solutions. Couple that data with reams of even more data from end-user applications and third-party sources, and you can easily see why it’s so difficult to get clean, curated data.
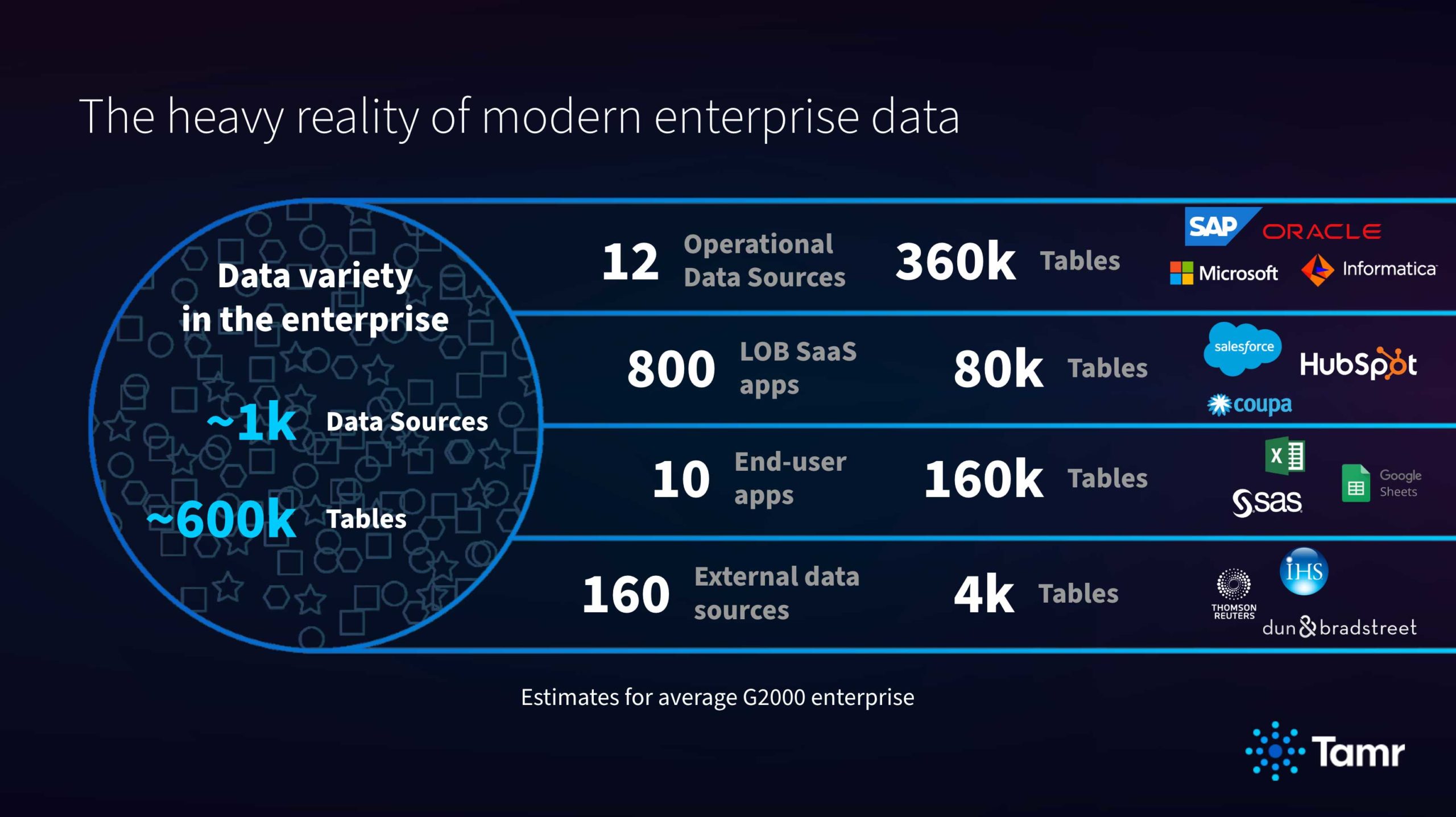
A Decades-Long Struggle Continues
Since the introduction of business process automation in the 1970s, organizations have struggled to ensure their data is clean. In the 1990s, big data infrastructure emerged, along with data warehouses. Solutions from companies such as Informatica and Cloudera rose in popularity, but the challenges with data quality persisted.
In the 2000s, companies began to expand their focus to democratizing data, or giving end-users the ability to access and use data to make decisions. They implemented self-service analytics tools such as Qlik and Tableau, but soon realized that these tools merely fixed the facade. And while users could now access the data, they didn’t trust it. Why? Because the tools didn’t address the underlying challenges of data quality.
Fast-forward to 2015 and the introduction of the data cloud. Companies, desperate for a solution to provide the quality data that the organization needed for analytics and decision making, embraced the data lake. They loaded all of their data into the data lake, but because the data they entered wasn’t clean, they soon realized that what they created instead was a data swamp.
To truly overcome data quality challenges, companies must manage their data as an asset. And while data lakes, analytics, and data governance are important elements of this effort, many companies fail to include what I believe is the most important part: data mastering.
3 Data Quality Issues that Data Mastering Can Solve
With data mastering, companies can address three persistent data quality issues:
- Enrichment
Let’s start by looking at enrichment. Companies receive source data with varying levels of quality. It may be incomplete, inaccurate, or ill-formatted. Enrichment services usually focus on a specific domain, providing information such as commodity information like addresses all the way to firmographic information or information on corporate hierarchies.
The output of enrichment services is cleansed data. They also provide valuable metadata that includes data quality scoring and validation status. Companies can query this metadata using simple business logic to compare the input and output records. A high-quality enrichment service will reduce the time spent manually identifying or fixing data records.
- Categorization
Next, let’s explore the categorization of data. Here, you classify data into a tree or taxonomy. And the definition of the taxonomy could use categories related to the data itself or its use. Data assigned to certain nodes are then flagged as requiring further data quality investigation or remediation. Companies commonly use this approach to categorize metadata such as datatypes, common values, etc.
- Mastering/Golden Records
Finally, there’s the overall mastering of data and production of golden records. For this process, you read source records, identify duplicates, taking into account messy data, and create clusters linking duplicate records. From there, you can create golden records which represent the best possible version of the data based on the information available. By analyzing these clusters, you can identify data quality issues such as multiple records from the same source or outlying records.
You can also analyze golden records to flag data that doesn’t meet a specific set of defined criteria, and you can use them to correct data quality issues such as incomplete data. Using golden records for this analysis also helps you identify records with true quality issues, versus those with issues that you can solve by simply matching the data better.
Deploying a Mastering Pipeline Across the Enterprise
To improve data quality, organizations need to deploy a mastering pipeline across the enterprise. Start by identifying the sources of data. Focus on capturing the most critical sources, as you can always go back and add more later. Then, identify which domains of data you need to link together to deliver impactful output. Think about what provides high business value. As an example, suppliers and products often pull from the same data feeds.
Next, you’ll employ the data quality methods discussed above: enrichment, categorization, and overall mastering/golden records. That way, you can automatically correct any underlying data quality issues. You’ll also need a persistent ID that you can use across the organization. A persistent ID extends beyond IDs tied to specific systems (like a supplier ID, which may only exist in your ERP), and helps you take advantage of broader mastering capabilities such as the prevention of duplicate entries at the point of capture.
Finally, you’ll need to collect feedback from your data consumers. This feedback loop is critical to correcting data issues.
Cloud-native, machine learning-based data mastering makes all of this possible. Unlike traditional, master data management (MDM) solutions which rely solely on rules, modern data mastering scales as demand changes. It’s designed with machine learning at the core, and uses it appropriately to scale your efforts up or down as needed as the volume and variety of your data changes.
With mastered data, your organization can feel confident that you’re providing a clean, curated, single version of the truth for use across analytics and decision making.
To learn more about how to improve data quality with data mastering , and to hear how customers like you use data mastering to improve data quality, please watch this webinar.
Get a free, no-obligation 30-minute demo of Tamr.
Discover how our AI-native MDM solution can help you master your data with ease!