What is Data Unification?
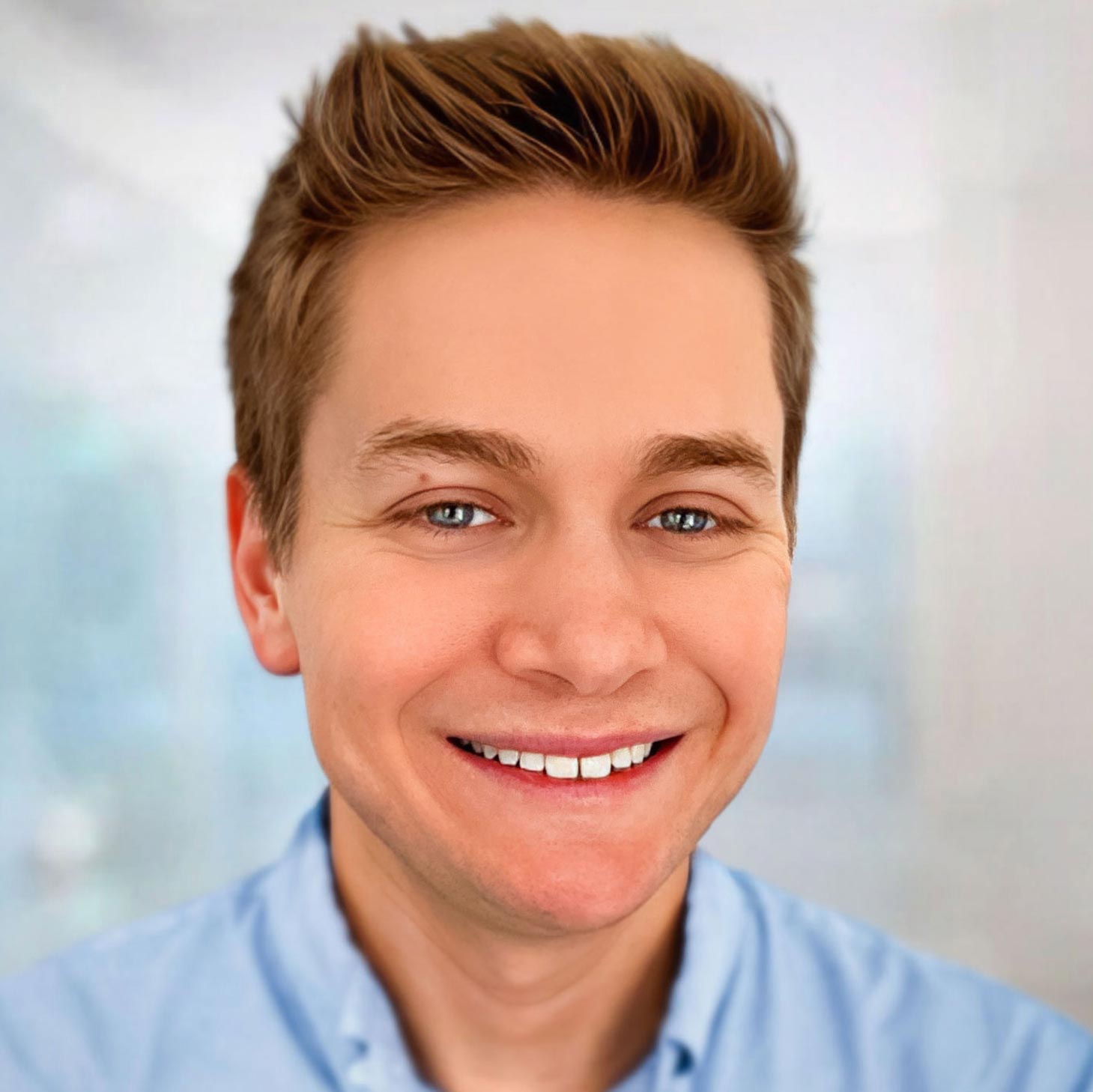
To those unfamiliar, data unification may seem insignificant. After all, it can’t be that hard to unify data, right? Unfortunately, this is a huge misconception. Data unification is an incredibly complex process and one of the biggest challenges many large organizations face today.
Before we dig into how it works, let’s take a look at the definition of data unification:
Data Unification (n): The process of ingesting data from various operational systems and combining them into a single source by performing transformations, schema integrations, deduplications, and general cleaning of all the records.
The Data Unification Challenge
To understand the major challenges with data unification, think about all the different systems and programs used at your organization. Each one captures data differently. Now imagine trying to combine all of the data across those systems and programs into one master source. This process is incredibly difficult to achieve at scale, especially when it involves hundreds of thousands of datasets.
To give you a better idea of what this process entails, here’s a high-level breakdown of the data unification process from the viewpoint of Michael Stonebraker:
- Ingesting data, typically from operational data systems in the enterprise.
- Performing data cleaning, e.g., -99 is often a code for “null,” and/or some data sources might have obsolete addresses for customers.
- Performing transformations, e.g., euros to dollars or airport code to city_name.
- Performing schema integration, e.g., “salary” in one system is “wages” in another.
- Performing deduplication (entity consolidation) e.g., I am “Mike Stonebraker” in one data source and “M.R. Stonebraker” in another.
- Performing classification or other complex analytics, e.g., classifying spend transactions to discover where an enterprise is spending money. This requires data unification for spend data, followed by a complex analysis on the result.
- Exporting unified data to one or more downstream systems.
As you can see, unifying data is complex, which is why the vast majority of today’s organizations are facing a data unification crisis.
The Data Preparation Ecosystem
Because of this data unification crisis, there is an immediate need for organizations to have internal and external datasets that are agile and curated. And organizations that provide these types of datasets are part of the rapidly-expanding data preparation industry that is expected to grow to $12.89 billion by 2028.
That explosive growth is largely because of the fact that data scientists spend 60% - 80% of their time cleaning and preparing data alone. New tools aim to greatly reduce this time and are quickly becoming the industry standard, with many new projects incorporating the use of data preparation tools.
Data unification is an integral part of this new data preparation ecosystem and is an essential input to tools used by analysts and consumers, such as self-serve data prep tools and data catalogs. These users can’t be expected to be productive and generate meaningful business insights without a foundation of trustworthy data, which data unification provides. Further, the emergence of DataOps and the strategic need to increase analytic velocity in the enterprise has accelerated the move towards this modern architecture.
Out With The Old: The Traditional Process to Unify Data Isn’t Effective
Legacy approaches to data unification typically revolve around ETL and MDM.
ETL, or Extract, Transform, and Load, involves writing an upfront global schema and then relying on a programmer to understand the schema and write conversion, cleaning, and transformation routines as well as all necessary record updates.
MDM or Master Data Management involves creating a master record where all entities across the organization are defined, and then merging all records to match the master.
Both ETL and MDM are incredibly labor-intensive, requiring complex rules systems to be developed to unify data. These systems have a high upfront cost to develop and are costly to maintain. As a result, data unification efforts are often limited to a select few high-value data sources.
In With The New: A New Approach To Data Unification Is Working Wonders
Data products are emerging as a new, more effective way to unify data. Data products use a powerful blend of AI and human expertise to conquer the data. The result is unified, consistent, enriched data, something that was near impossible to achieve with the old methods.
Data products accelerate time-to-value by providing the clean, trustworthy data organizations need to work smarter. They enable you to access and connect your data, organize and enrich it, create consistency across disparate systems and sources, and curate and update it. Simply put, data products deliver the best version of your data.
Unifying Provider Data at P360
Leading technology solutions provider, P360, needed to create a solid foundation of accurate customer records for its pharmaceutical customer so they could achieve their goal: drive better marketing to the healthcare providers they count as customers, and increase engagement with them. Unfortunately, the customer lacked a solid foundation of trusted data, so for P360, fixing that foundation was priority number one.
But P360’s customer faced two big challenges. Like many B2B companies, P360’s customer had diverse data from a wide variety of sources. With over 150 sources of internal data (physician names and addresses) and external data (prescription histories and claims information), gaining a complete and holistic view of their customers was no small task.
In addition to the challenges that come from unifying multiple data sources, the customer also had a time constraint: the project needed to be completed in six months.
To meet the customer’s needs, P360 knew they needed to abandon their in-house, rules-based tool in favor of a cloud-based, AI-driven solution. Using Tamr’s Healthcare Providers Data Product, P360 delivered high-quality provider records to their customer in just six weeks.
Tamr’s Healthcare Providers Data Product allows organizations to gain a complete, unified, accurate view of provider data so they can target messaging, measure their addressable market, manage complex relationships, and increase revenue. With Tamr, P360 helped their customer master millions of provider records in weeks and create golden records containing unique customer IDs as a consistent identifier and single source of truth. As a result, P360’s customer gained the accurate, high-quality data needed to feed their CRM system and drive effective sales and marketing campaigns.
Final Thoughts
According to the latest estimates, 3.2877 million terabytes of data are created each day. And this number is only growing. Businesses need to unify data using data products to make sense of this endless data stream to make smart, data-driven decisions and compete in a global economy. You’ve heard the expression knowledge is power. For modern-day businesses, that knowledge comes from having complete access to reliable, up-to-speed data, and avoiding these pitfalls along the way.
To learn more about how Tamr can help you address your data unification challenges, please schedule a demo.
Get a free, no-obligation 30-minute demo of Tamr.
Discover how our AI-native MDM solution can help you master your data with ease!