All’s FAIR in Life Sciences
The FAIR data principles are finally emerging from the halls of academia to find their place in the commercial world. And not a moment too soon for life sciences businesses, as they begin to treat their R&D data as first-class assets after decades of proliferating and languishing data silos.
“FAIR” stands for Findable, Accessible, Interoperable and Reusable. It’s the mandate behind an emerging common-sense but critical standard for scientific data. As I wrote in a previous blog post, the problem of siloed data has persisted in the science community despite efforts over the last 15 years to identify and address the root causes (technical and organizational). However, FAIR–an initiative that began at an industry meeting in 2014 and in the publication of the FAIR data principles in 2016–has since been making real progress.
For instance, Bayer presented on the subject of “A Framework for Making Data FAIR at Bayer” in June 2018 and Janssen Pharmaceuticals is a partner in FAIRplus, a project that “aims to develop tools and guidelines for making life science data FAIR.” There are definitely some difficult challenges to FAIRification of data assets–not the least of which is the culture change required in organizations and in individuals to see data not as something a person or department owns but as an enterprise asset. But with the right leadership and starting with the less-challenging principle of making it possible to just find the data, progress will continue.
Why FAIRification is Essential to Life Sciences
Common threads in my conversations with biopharma companies are: “Our data is all over the place. We can’t find it.” and “The cost of developing drugs is horrendously expensive. It’s unsustainable at this point.” Which is why, while companies are cutting back on budgets as much as they can, they’re investing in enterprise data unification. It’s simple math: the more clean data they can pull together, the more “signal” they can get. But drug discovery can only happen if the data can be discovered.
It’s no wonder that “FAIRifcation” (as biopharmas call it) is top of mind among enterprise architects building the enterprise data architectures for the next 5-10 years. Even as they build out central repositories like data lakes or translational data warehouses, biopharmas must ensure researchers can find, access, understand and use data quickly, without proprietary tools for each data asset or hitting the equivalent of data firewalls. Further, any systems and data standards need to be interoperable. If you can’t find that piece of data that once was so significant or promising, all is for naught. Data *is* the business.
In a recent article about FAIR in Drug Discovery Today, the authors observed:
- Biopharma R&D productivity can be improved by implementing the FAIR Data Principles.
- FAIR enables powerful new AI analytics to access data for machine learning and prediction.
- FAIR is a fundamental enabler for digital transformation of biopharma R&D.
Moreover, the FAIR principles stress crucial preconditions for data sharing, urging researchers to take the possibility of subsequent data sharing and reuse into account from the outset.
When implemented proactively and properly by life sciences companies, FAIR principles can help create a data catalog, the digital equivalent of a card catalog in a library. Researchers, scientists, and business people can search and find the exact data asset in diverse scientific data, right down to the form (e.g., hardcover or ebook) and edition (latest or dated version).
All Roads Lead to Metadata
Metadata (data about the data) is what makes card catalogs work in libraries across the world. Ditto for data catalogs or master data management (MDM) systems in life sciences companies.
There are three kinds of metadata:
- Technical metadata: the form and structure of each dataset, such as the names of fields, data types, etc.
- Operational metadata: lineage, quality, profile and provenance as well as metadata about software processes
- Business metadata: what the data means to the end user, what are the semantics
Tamr can provide operational metadata from, say, mastering records, that could go into a data catalog; technical metadata about input datasets from profiling metrics; and the business metadata, the meaning of the data, which can be provided for Tamr-specific data by providing the appropriate vocabulary to define it. And as life sciences companies turn to Tamr to help unify data across silos, our solution can provide descriptive, rich metadata at scale, supporting the FAIRification process.
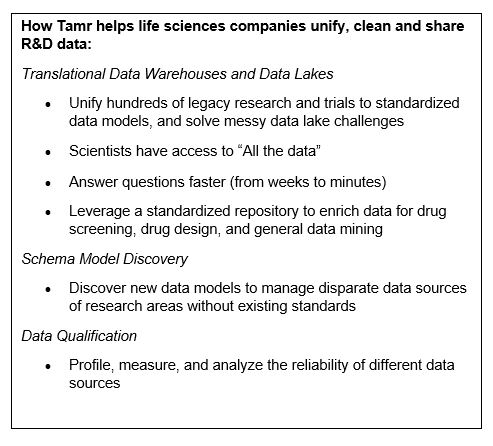
First, the Tamr data unification process starts by ingesting data from multiple sources, including the metadata where it exists. Tamr can tell companies the owner of the data, the quality (completeness, when last updated, what formats used) and how the data asset was created (if the dataset was the result of data integration, what were the original sources?). We can add this to the metadata that Tamr makes available to data catalogs. Second, Tamr also makes available metadata about its data unification processes, creating complete lineage for the data.

The Tamr system can capture information about the input data sources as part of the ingestion process for upstream provenance.
This pays off in multiple ways, including:
Master Data Management for R&D Data: In helping life-sciences companies create agile, scalable master data management (MDM) systems, the Tamr system creates metadata about its mastering process and includes this in the metadata it provides to data catalogs. With more-complete descriptive metadata, scientists now can have a confidence level in each piece of data they use for research, testing and analytics. For example: confidence that certain rows or entities of interest occur in the right cluster and thus can be trusted (or not). Lacking such history on a piece of data, people would be tempted to go off and create new data, wasting time and money while contributing to the death spiral of dirty, duplicate data (the bane of scalable MDM).
Long-Tail Data Mining: Metadata is essential to find prior art. For a biopharma unifying clinical data with genetic biomarker and biospecimen data for tissue management, researchers can now conduct different analytics across studies. Researchers who are investigating certain diseases and therapeutic areas can locate relatively similar biomarkers already targeted to manage those diseases. The results could help identify a drug already approved for a specific therapy, enabling finetuning of the drug’s chemistry for a new target instead of starting over from scratch. Metadata can help speed the R&D process, including demonstrating previous testing history to the satisfaction of regulatory agencies. In the past, researchers lacked the cross-therapy, cross-therapeutic similarity revealed with the help of metadata.
Pre-competitive Cooperation/Collaboration: When biopharmas can share pre-competitive data, it helps the whole community. For example, three or four companies may decide they’re going to create a data warehouse and unify data so that they can jointly work on accelerating basic research. Such effective data-sharing could mitigate the front-end risk and expense associated with greenfield drug development. However, the only way that companies can share information with each other effectively is if they have a productive way of making their data FAIR. Data must be not only findable but also interoperable–that is, based on common data standards, including metadata that fully describes the data for collaborators.
Happily, momentum is picking up in this area. In July 2019, The Pistoia Alliance, a global non-profit that works to lower barriers to innovation in life sciences R&D, announced the launch of its FAIR Implementation project, backed by pharmaceutical companies including Roche, Astra Zeneca, and Bayer. The first project milestone is the release (by the end of 2019) of a freely accessible toolkit to help companies implement the FAIR guiding principles for data management and stewardship.
“As a pre-competitive consortium, the Pistoia Alliance is the right body to undertake this project to develop a FAIR toolkit for industry use, which is being supported actively by Pistoia member organisations. The FAIR toolkit will be designed to help industry to implement FAIR in a very practical way. This is because all life science organizations will need to take similar steps on this journey, so all will benefit from collaboration and sharing through this project,” said Ian Harrow, a consultant to The Pistoia Alliance.
Beyond R&D
FAIRifying metadata can also help beyond R&D, including distribution, marketing, selling and monitoring medicinal products. For example: Biopharmas need to track the variety, quality, and performance of medical products distributed across the globe to meet sales/marketing goals and regulatory compliance.
IDMP (Identification of Medicinal Products) is a suite of five standards developed within the International Organization for Standardization (ISO). IDMP uniquely identifies medicines (e.g., manufacturing source, form, documentation) so they can be tracked as they are commercially packaged, possibly rebranded and distributed to various countries. IDMP helps manufacturers both understand supply chain issues and report to regulatory agencies. Tamr is using data classification and agile product mastering in helping several biopharmas to deploy IDMP.
Provenance and traceability of data sources is critical in understanding where a medicine’s raw materials are sourced, where it is manufactured, packaged and warehoused prior to distribution in order to address any quality issues that might arise. Therefore, making this metadata available as part of any mastering of medicines across divisions provides tremendous value.
Three Takeaways for FAIRifying Life Sciences Data
- Have a FAIR vision. Life sciences businesses need FAIR to make enterprise data management systems work. Think of a single library-style catalog for your R&D data.
- Pick the right, ideally open tools to implement the vision: capable of capturing, cataloging and mastering at scale.
- Make reusability part of the data culture.
While you clearly can’t make everyone go back and re-document every data source, you can introduce a process of change management that moves your company into a future where your data will be engineered for maximum reusability.
For example: One Tamr customer is automatically quality-monitoring assets as they are being pulled into its master data management system, based on certain criteria. If an external data source consistently has very poor data quality – poor formatting, insufficient cleaning, too many NULL values – the company won’t buy data from that supplier any more. If an internal data source consistently has very poor data quality, it creates a teachable moment for data stewards to help the provider get the data in shape (including appropriate metadata). Tamr recently added a new universal data quality connector that can help surface such problems and provide quality insight into data sources.
What this all boils down to is this: You can’t do drug discovery if you can’t discover your data. And you can’t easily discover your data without FAIR.
To learn more contact us for a discussion or demo.
Get a free, no-obligation 30-minute demo of Tamr.
Discover how our AI-native MDM solution can help you master your data with ease!