Tamr and Google Agentspace
Better, Smarter Agents Reasoning with the Best Data Context
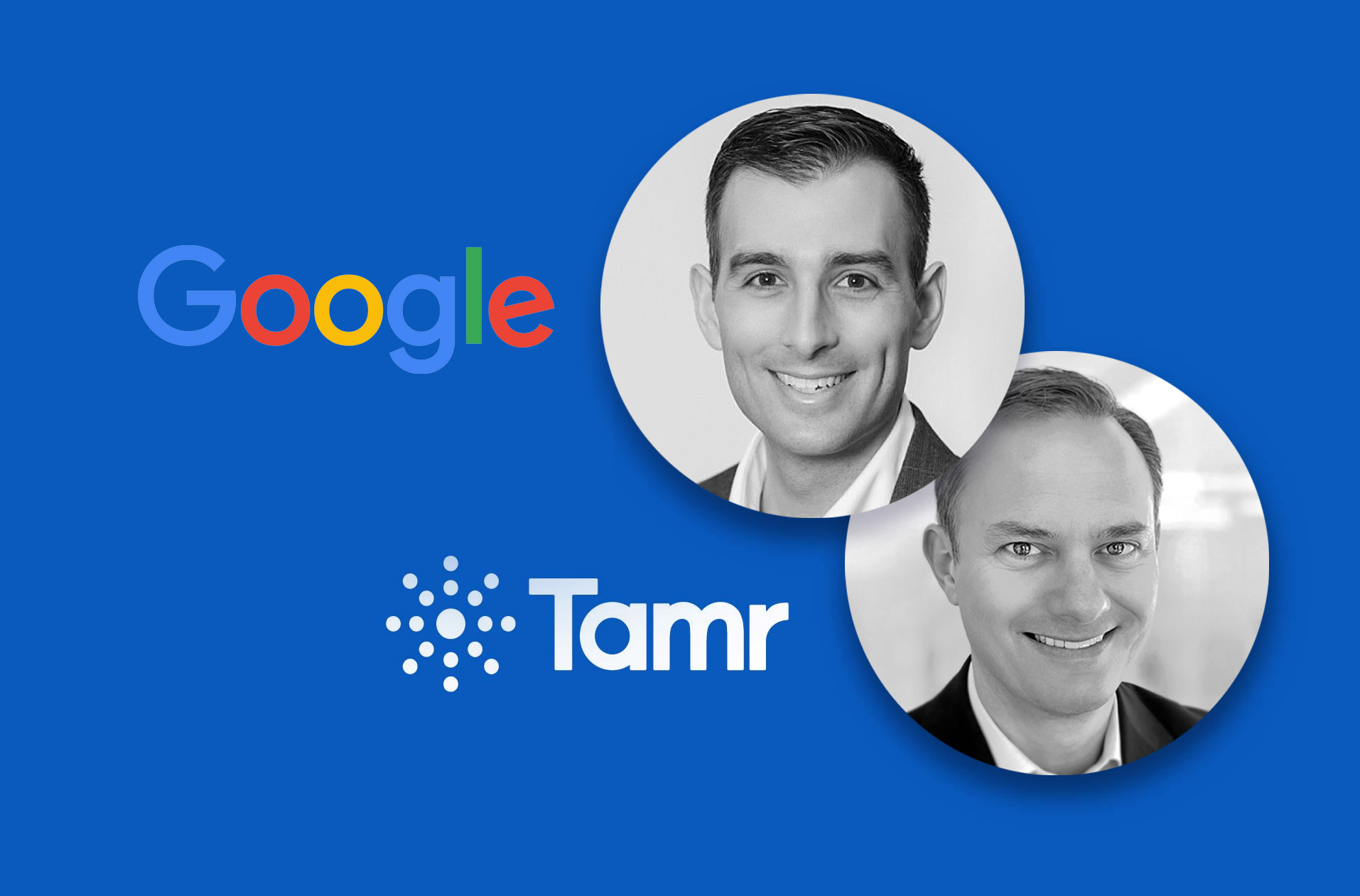
Agentspace is redefining productivity with a unified platform that eliminates the need to navigate fragmented systems. By combining Google’s industry-leading search, AI, and conversational agents, Agentspace empowers you to find, understand, and act on enterprise data faster and more intuitively than ever before. In this on-demand webinar, you’ll learn how Tamr integrates with Agentspace to deliver powerful business context. With Tamr’s AI-Native MDM platform, companies can surface a complete and accurate enterprise knowledge graph – providing trusted data context across all key systems and databases.
Learn from our expert speakers:

What you’ll take away:
- How Google’s Agentspace uses AI to assist with complex tasks and decision making
- How Tamr’s AI-native MDM delivers clean, connected data to serve as high-quality context for AI agents
- Real-world examples that highlight the benefits of a Tamr agent
Agentspace is redefining productivity with a unified platform that eliminates the need to navigate fragmented systems. By combining Google’s industry-leading search, AI, and conversational agents, Agentspace empowers you to find, understand, and act on enterprise data faster and more intuitively than ever before. In this on-demand webinar, you’ll learn how Tamr integrates with Agentspace to deliver powerful business context. With Tamr’s AI-Native MDM platform, companies can surface a complete and accurate enterprise knowledge graph – providing trusted data context across all key systems and databases.
Learn from our expert speakers:

What you’ll take away:
- How Google’s Agentspace uses AI to assist with complex tasks and decision making
- How Tamr’s AI-native MDM delivers clean, connected data to serve as high-quality context for AI agents
- Real-world examples that highlight the benefits of a Tamr agent
Watch Webinar!
Want to read the transcript? Dive right in.
Alright. Can everybody see me and hear me? Hopefully.
Wonderful.
Anthony, if you wanna go ahead and share slides, perfect. And we'll go ahead and advance to the next.
Good morning. Good afternoon or good evening, everyone. Thank you so much for joining us for today's session. Better smarter agents reasoning with the best data context presented by Tamr and Google agent space.
Before we begin, I'd like to cover a few housekeeping items. Closed captioning is available. Simply hover over the virtual stage and click the CC button located at the bottom of your screen. We encourage you to submit your your questions and engage in the chat, leveraging the q and a and chat tabs in the engagement panel on your right.
And we'll do our best to answer all questions at the end of the presentation.
And if we are unable to get to your question, we'll follow-up with you directly afterwards.
We've also added some additional resources, which are available in the docs tab, also located in the engagement panel on your right. And there you can find some additional related content.
And lastly, today's webinar will be available on demand after we wrap up. It will be sent to you via email directly. And with that, I'm going to pass it over to Tamr CEO, Anthony Dayton, to get us started. Anthony, over to you.
Excellent.
Alright. Well, thank you so much, Kara.
And, I'm Anthony Dayton. I'm the CEO here at Tamer.
And I have the pleasure of, joining you today with our friend and partner, Jim Fairweather, from Google Cloud.
Jim is the head of AI go to market for North America at Google Cloud. And in this role, he's leading the strategy, and execution for bringing Google's AI and machine learning solutions to customers all across North America. And he has a focus on helping businesses, of course, but also helping, high-tech startup the high-tech startup community of which, Tamer is one, really take advantage of and scale the AI capabilities that Google's developed, as they bring them to market. So it's a tremendous partnership. We really value the opportunity to work closely, with, Google Cloud on this stuff, and Jim has been a great partner, in that endeavor. So, welcome, Jim.
Thanks, Anthony. Glad to be here.
So let's talk a little bit about the agenda, for today. We're gonna start and really start at a pretty high level talking about, what's going on in the industry around agents, and agentification as we'll talk about in a moment. And really set some context for how we think about the shifts and changes broadly in the industry. They're gonna dig in some detail around Google agent space, the exciting new set of capabilities and product offerings that Google's announced fairly recently at Google Next, which I had the privilege and honor of being, in attendance at, as was Jim, of course. Then we'll talk about how, about Tamr and talk about AI native MDM. And then we'll kind of put these two worlds together. We'll think about what Tamr is doing in conjunction, with agent space, and then we'll leave you with a little bit of the art of the possible, what you should expect to see coming, from a product perspective.
So let's, let's jump right into it and let's talk about, AI and agents.
It's always risky if you put up a slide that has a timeline that goes back to the nineteen seventies.
But I did think it was useful to put what's happening here in context of a broader set of shifts and changes in the enterprise software, space.
So, you know, in the seventies going through to the nineties, we saw an enormous investment in taking manual and often cases paper processes and, digitizing them, putting them into databases. And that built a whole series of amazing, software companies, database companies, etcetera.
In the twenty tens, having digitized many of these processes, we saw organizations benefit from this digital data, and they could optimize those processes. They could build dashboards and analysis on top of them and really think about how to optimize the work people were doing because now they had access to digital versions of those processes.
What I think is going on for the next twenty years, the next twenty or thirty years, is, we're really shifting to automating a lot of this work and using agents and and AI to, really think about how we do work differently. How do we actually work differently in the context of these things? And in many cases, maybe having fewer people doing boring manual tasks, and being able to turn those work over to agents that can work, on our behalf.
So, with that as broader context, this new agent centric world that's gonna really be, I think, a big driver for the new interface for enterprise software, in the next twenty, thirty years, that introduces a whole series of new and important requirements.
The biggest of which is better quality data.
As we see the rise, and as many of you on this call think about implementing AI technologies, and you think about turning those over to, agents around agentification.
If you are working with low quality data, you really run the risk of turning over bad data to those agents and having them make really, in a way, bad decisions with bad data.
It also offers an opportunity for context. So being able to pass high quality data into these, agents, means that they're now operating on the best data that your organization has.
And in a way, you should think about this as what would a human being do? So if if you ask a human to do something, they often will dig around inside your organization, try to pull together information, and then use it as part of the work that they're doing. And in that context, it is something we naturally do as humans. We think that's an important requirement in this age identification world, Which brings us to the third new requirement, which is partnership between humans and agents. That we're not imagining a world where we turn everything over to the human, but getting feedback and verification, keeping humans in the loop, is a really important part of this. Let me pause, Jim. I don't know how to put you on the spot, but, you know, hopefully, this fits with your world view and Google's world view.
No. Absolutely. No. It it resonates really well. And I I think I like what you said, and we'll get into this more about that.
As we enter into this agentic era, there's gonna be a new user interface. And I think every company needs to think about that, not only just for them and the products that they serve out to their own customers, but how their employees internally interact, within their organization. I I see this as being competitive differentiator, for hiring and and kind of the war on talent that we have. But you said it, and it it all comes back to the data and making sure we have context.
I mean, you can see this already in prompting and different things of helping these LLMs.
And then also on the partnership side, I think that will be a really interesting thing to watch at how much kind of leash we give these agents, and in, you know, what tasks they're they're doing. Because obviously, with more sensitive, confidential, secure stuff, you need to have it on a pretty tight leash.
So Yeah.
Couldn't agree more. Now, the other thing you should notice from this slide is if the timeline goes out to the twenty thirties, again, always a risky thing to throw in a slide. But the the the reason for putting twenty thirty there is, I firmly believe we're very much in the early innings of this megatrend. And that is to say, the capabilities of, large language models and other AI capabilities, they are really at the beginning of, their capabilities. So as, and many of us have used a lot of these technologies.
You know, it's really impressive what they can do. And yet, there are in a way, it's it feels like the beginning of something, not even the middle, and certainly not the end. And and the way to think about that, in my opinion, is that, today these models are a lot like interns.
And so the analogy I'm throwing out here is, contrasting an intern with your best full time employees.
Your best employees, you know, I say they have a work ethic of c. You know, like, you know, they work, normal business hours. They don't work weekends. They take holidays, etcetera.
Whereas an intern, you know, they don't have a life. They're willing to come in Saturday and Sunday. They're gonna come in at early. They're gonna stay late.
They work really, really hard. And large language models and models in general work the same way. Right? So you they're perfectly happy to work on the weekend as well as they're willing to work during the weekend.
Full time employees, however, balance this, with extraordinarily deep and rich knowledge and information about your business. They know, where all the information and they know all the people. They know how to get access to all of that context and knowledge. And they're generally very, very smart. Like, they really understand they have the data and the knowledge, and they and they they marry that with some rich intelligence.
Interns, on the other hand, know nothing. Right? They work really hard, but they just don't know anything. And, you know, they haven't had the experience that your best full time employees have had in there, and that rude intelligence is sort of lacking there.
And the risk with these interns, of course, is that you send them off on a task, they work extraordinarily hard, on the wrong thing. And again, the same problem exists with models. Now just to add one layer of detail to this, I think it's it's even worse than this because they're not just like interns. They're actually like MBA interns.
And the challenge with MBAs, and I can say this as as one as, you know, hopefully not insulting too many MBAs out there, is not only, are these interns lacking context and knowledge, they are extraordinarily confident and eloquent. Which is to say that they will say incorrect information with great confidence and and and sort of articulated brilliant. And again, we've all seen models do this.
And so this is, I think, the state of the art of where we are today.
But again, I think we're right at the beginning of a big, mega trend here. So, Jim, I don't know if you wanna layer any additional context on here.
No. I I love I love how you look at this. This is something we've actually, tried to help a lot of the companies with as they think about how do we even what what use cases or what problems should we put, AI, you know, or power AI onto. And I think it's when you think about it, it's like, what if you had a thousand interns, that could work twenty four seven? What would you have them do? It's a great framing for, like, the problems that you wanna because a lot of this is just, you know, part of, like, the low hanging fruit with the as we think about agents is how do we take away kind of the joyless work, that doesn't require, you know, the the human interaction or or EQ that, you know, we might need.
And so I I love this as a framework, and, and I and I agree.
Excellent. Well, let's shift gears a little bit and talk a little bit, you know, have you share a bit about what Google's doing with with agent space.
Yeah. Sure. Let me, pull up my screen here.
Yeah. Thanks, Anthony. This is, you know, look. We're in a really fun time in technology, and I think we're all we're all living through it. And, it's one of these times where everything is new and, at the same time, everything's the same, and and we're just working all through it together. And so I think as you as we talk about agent space here today, what I'm gonna show you is kind of the early stages of where we are. This product is not even fully generally available yet.
And what I'd like to say first too is just is thank you to Anthony and the Tamr team, for working with us on this because, Tamer something I've always admired about Tamer is just that they're they're early.
And I think fortune favors the bold, and that's been true for Tamer in a lot of different respects.
But they've come on board with us early, not only to be a client, but to to be a development partner as we look at building connectors and agents.
And I think, you know, we're only just getting started with this ecosystem, and it's really exciting to see what's what's coming.
But if you think about the problem we've kind of always had, for decades is that we've had a lot of technology that has worked together.
Well, I'm sorry. We've had a lot of technology and across the years, and some of it's been good and bad. The position we find ourselves in now is that we have awesome technology.
It just doesn't all work together very well. And so when you think about, you know, getting all this technology and how do we make it work together, there's a lot of ways that you can do that wrong. So the execution is really, really important, because we need to make sure we're not entering a new fragmented environment as we enter in all these agents, as we enter in using different hyperscalers, as we enter in, using different applications.
And so that's one of the things we're trying to really solve for and work with is, you know, a provider that can drive that kind of end to end integration across all of your enterprise systems.
And so as you think about that in the different use cases, it's like, what if we could have all our enterprise systems talk together?
And, you know, I think about for the audience in this call is, you know, just take, like, the marketing and sales, for instance. What if we were able to go back and look at the best campaigns from last year across our CRM and, all our different, you know, content generation platforms and understand and summarize, get the insights from last year, and then be able to create with multimodal approach, new video, new audio, new, pictures in print?
What if in sales, we were able to get our, you know, our customer's latest earnings reports, summarize those, provide insights, and generate a podcast about it. That's stuff that's available today. We can do that now. Whereas, you know, before, this would take I know something we do every year is we're constantly changing and reorging.
As I look at North America and what we did this year with reorging, how we position and, you know, handle our clients, there's always this, knowledge exchange that goes on about, you know, different clients, especially I'm sure all of you have large clients who you work with.
There's always a trade of information, sometimes be, you know, hour, two hour phone calls about just sharing knowledge information.
Now you can take all the documents, all the meetings, all the notes, all the prep, along with external sources and create a fifteen minute podcast that can get people up to speed and make yourself a lot more efficient as you head into that initial call and ask, you know, better questions with better insights. So there's a lot of different use cases here, that we can go into, but but those are a few, that I think are just, again, low hanging fruit on some joyless work that that can be solved for immediately.
And so, this is kind of the interface. And it's funny, we've been using this internally ourselves and, you know, working out some of the kinks.
This user interface has changed about three times over the last three weeks.
And, Anthony, you've probably seen it change even since Next. So it's been fun and something we're iterating on really, really quickly, but, but this is what it looks like. And so we focus on these three different areas, which is finding, which is kind of, you know, the ability to use AI to search across text and image and videos to get those deeper insights, not just put put the information up, but put together in insights from it. And then we use the AI to reason and make sense of that complex information. And so that was kind of the podcast example with NotebookLM, and we'll talk about that a little bit more. But, okay, fine. If I have all this information, you think about, like, a company like Tamr is able to provide you all that current context information at your fingertips.
Okay. Then how do I think through it now that I have it? What what insights can I take out of this that I wouldn't have been able to see otherwise?
And then that gets us to our last point, which is act. And this is where we have the agents with human in the loop be able to take action on our behalf with some of those, you know, joyless tasks. Think about things as simple as Lender and scheduling and sending email follow ups, or, you know, working on Jira tickets and things like that.
And so we have a bunch of different connectors, over a hundred connectors for different enterprise applications.
It's all gonna be underpinned by our security and compliance controls.
And so these are coming on more and more every single day, and this is where the ecosystem will really, look to get built up. But the thing we're trying to do is provide a a trusted and secure framework to make all of this happen and really unleash, the information and the data across your enterprise.
And so taking a a level deeper looking at this kind of multimodal approach, you know, I I think a lot of times we think about text as the only way to get information or charts or, you know, sheets or slides.
You're now able to upload audio.
You're now able to upload, videos, pictures, and you can all use that as insights to get out and find whatever you need, for your enterprise and for whatever task you're trying to accomplish.
The next is this understanding piece, which is, again, underpinned by notebook l m. If you haven't played with this already, I would I would highly recommend you do. You can upload up to three hundred sources. If you want it for yourself and you need a a pump up, put in your LinkedIn, you know, address or your resume, and it'll make a whole podcast about you.
And, really, this is now available in fifty different languages. So if you're a global company, and need to, you know, make sure that everybody in your organization can use it, they can. But it's grounded in the information you give it. And I think that's really the important part.
It cuts down on hallucinations, incredibly well because it's only, acting on the information you give it. But you think about things like, FAQ documents and you have an event coming up, it can create all of that for you right away, with a click.
Again, this is the what we were talking about on acting is, you know, deploying and accessing agents to complete these tasks effortlessly. You'll see some things here which are like emails.
We're gonna provide Google is gonna provide out of the box some premade agents, that would like deep research and, this idea generator that we'll talk about.
But, also, this is where I think it's an incredible opportunity for ISVs, software companies, companies like Tamr to come and show up on a new surface for your customers and provide something that, they've never seen before. And I think that's where you can almost you can really increase the moat and competitive advantage you have by building an agent, that can do that can really blow people away with what it can do. It's taking your software to the net that next level, to be able to serve your customers in a way they never thought possible.
And that's what we're really excited about seeing this ecosystem kinda take advantage of.
Agent gallery. This is gonna be a place where as different agents come in, you will have, as administrator, kind of access to show or allow what agents people can take advantage of.
We're gonna have this ability for you to build your own agents. So for folks that don't need, you know, a programming background or design background, you can actually business users can create their own agents and publish them, to, to the enterprise if they'd like. So for those specific tasks that are being repeated and done over and over that might be specific to your job or your unit, your division, you can, again, create and automate those joyless tasks, and and really increase the efficiency.
Deep research is one we've come out with. If you if you played with Gemini, you've had access to this, but can work through a lot of different thinking and reasoning processes, and, again, being able to use your data, to think deeply and and give you an as you as you dissect kind of the complex, data questions we have.
And then this idea generation one is interesting. I think folks for a while have thought about how to use, LLMs and AI as a thought partner.
This kind of puts that on steroids.
And what this does is almost like a March Madness or playoff, setup of ideas. And so it'll actually put ideas against each other and eliminate down to, the one that you wanna go with next. So really excited about this. This will be rolling out shortly, something we announced recently at next.
But as you think about it, what what we want agent space to be, there's no doubt that agents are here and now.
And so what we want agent space to be is this place, where you can have expert built Google agents that we'll provide you from, you know, first party. You can have your high, your high code agents, which is something that, you know, a tamer would build or you can build internally for your own specific agents for internal folks.
And then we have our no code agents, which is something like something I could build for my team or or other divisions. Then we're gonna have our partner agents, which will be available in the Google Cloud marketplace, in a really secure environment, for folks. You know, Tamr can upload theirs, and you can get it right from Google Marketplace. And, again, that would be part of, commitment contracts and and things like that. So, this is a view of where that would be, and we have, you know, great partners like Accenture, Deloitte, Tamers here.
And this is gonna be a place where, you know, people can can get them. So it's it's not gonna be rogue. We want to derive controls and, you know, administrative support on how these things get downloaded and used.
But we're building out an entire marketplace for it. I mean, we think about this as kind of being the next wave of you think about an app, there will essentially be an agent store, now. And so I I think for you, as as folks think about this for internally, but also for how you show up to your end customers, this is a place you don't wanna, be left out of. And so, we're really excited, to to work with a bunch of companies on this and and Tamer being one of our earliest. So, that's that's most of it from from me, Anthony. And, you know, again, I think the the underpinning on this and the thing that everybody has to keep in the forefront of their mind is security.
This and I think that's, you know, one of the reasons we launched this agent to agent protocol to kind of, like, support the the MCP revolution that's going on is, we wanna make sure everybody's secure. And and as we use our knowledge graph for search, there's a personification that goes by that. And so we wanna make sure people are accessing the right information that they can, and that's why we wanna provide this this platform for everybody, to make sure that they can get the best information for them specifically in the right context. So that's that's it for me, Anthony, but I'll I'll pass back to you.
Cool. That's great.
So let's jump back into the slides. And let's shift gears and and talk a little bit about, Tamr sort of generally, and then, we'll dig into, what we're doing together, around agent space. So, Tamr, if I sort of step back, came out of academic research at MIT around, in a way, a simple problem. A simple problem but hard to to do, which is imagine I had data across hundreds or thousands of tables, millions or even billions of records of data, and it was all about the same thing. It was all about my customers or my suppliers, whether those customers are people or companies. And what I really wanted to do was unify that data, bring it together.
That is a less and difficult task and, actually love that, way of thinking about a gym. Like, what's thankless and difficult work?
That's difficult and thankless work. And the academic research that, Mike Stonebraker and Andy Palmer were focused on was, like, can we train a machine to do that? Is that a possibility?
And it turns out, you know, after a bunch of academic research and eighteen patents, yeah. You can actually train a machine to do that work. And that became the, core central foundation of Tamr's AI native master data management solution. So and and we believe this to be true that at the core of every MDM problem is unifying that data, bringing it together, doing that at scale, and then putting on top of that a whole set of APIs and user interface, which makes it easy for people to consume the data, but also prevents data from being entered incorrectly and allowing people to surface the right data at the point of decision.
And that becomes this AI native MDM system.
And we'll come to this in a few slides, but at its core, really, what the conversation here around agent space is about how do I make that AI native system available, in a really simple way into, amazing new platforms like agent space from from Google. And what would that mean and what would that allow?
But, you know, master data management is a tough space, quite honestly, because historically, it's been really difficult for, you, for our customers, to be able to solve this problem. Typically, what we see customers doing is one of two things. Either throwing people at the problem. So they will literally take, teams of people, often in low cost, area, and they will go record by record and try to resolve data and fix it up, to curate it, etcetera.
That is really not scalable. It's typically quite expensive, and and difficult.
The other thing we see customers doing is, I always call this smothering it in process, which is to say lock the data down and make sure nobody changes anything, because any change the data then kicks off this expensive process of trying to, fix it and resolve it. And so this you set up these very complicated rules based systems which are themselves difficult to maintain and keep up to date. But really fundamentally what you're doing is preventing people from, modifying or using frankly even the data. And and as you would imagine, when you put up a system like this, people go around the system.
They're like, great. You know, if the master data is too difficult and complicated to use, I'll just go outside the system. Our view is with an AI native approach, we can break this log jam. We can actually get to this high quality data organized by entity, inside the enterprise and power an MDM system, using this approach.
That AI is the great unlock in the MDM space.
And what that looks like is a set of golden records around the key entities that matter to you as a business, your customers, your suppliers, people, companies.
And there's a set of tasks that we ask the AI to do. From mapping to a conform schema about that entity, cleaning and validating the data, verifying that the data is real in the world, so what we call a verified match.
Then the obvious, which is clustering the records together around the same entity, enriching it with third party data, and ultimately and frankly, most valuably, publishing that as a golden record. The best value the best record associated with this entity available, across a number of different endpoints. And so, what that looks like in in reality or in, you know, practically is major use cases. One, analytics. So we allow those golden records to show up in the analytic tool of your choice, powering dashboards and analysis, helping you make better decisions.
Directly through consumption interfaces.
So literally, I can go look at a three sixty page about that entity. And then through APIs into these operational systems so that in real time, you can power, your operational processes and actually do those processes more efficiently and save money. So sort of thinking left to right, many hundreds of source systems through Tamr running on and with Google Cloud out to these three important, consumption based experiences.
What we're talking about here in the context of, the relationship, with Google on agent space is one really important, I would argue, maybe the dominant consumption pattern that we are seeing and we will see in the future, which is through these agent like experiences.
So leveraging, standards like MCP, Jim mentioned, and also agent to agent to provide the important security wrappers around MCP.
We can take the data from Tamr, all of your mastered entity data organized across the key entities that matter to you as the business, and have that available, into agent space. And this directly provides the capabilities we talked about at the beginning of this webinar. Things like quality context and human to human or human to agent partnership that makes that data really, really, useful. Now, I think this is, maybe helpful to understand, you know, what is Tamr and how does it work with agent space, but it's probably even better if we show it, as a as a real thing.
So I'm gonna walk you through, a kinda click demo of what it looks like to use Tamer MDM data in the context of, AgentSpace.
And, so we'll start the demo, in a way without Tamr. So if you come into AgentSpace and you ask AgentSpace a really simple question, and, Jim, I hope you don't mind. I'm kinda picking on you for the demo. But I I asked the system, what do you know about Jim Fairweather?
And one thing we know about large language models is since they've been trained, generally on a lot of Internet data, is they don't know a lot about a specific, in this example, person named Jim Fairweather. And the model does kinda what you would expect, which is like, well, you know, Jim Fairweather is a common name. There's probably a bunch that there probably a bunch of Jim Fairweathers. I'm not quite sure who or what you're talking about when you do that.
And maybe this is where the conversation might have ended before Google made agent space with the rich agent and connector framework available to us. Because now what we can do is we can go ahead and click on Tamers MDM, where we've organized a bunch of data around customers, and now we can ask the question again. Search for Jim Fairweather, and we call out to, Tamr's MDM system and ask Tamr, what do we know about Jim Fairweather? It turns out we know a lot about Jim.
So, you know, here we've, pulled together a bunch of information about, you know, Jim, and, and that is now added as context into the conversation we're having around with this, with this agent. And now we can kinda do something sort of exciting. So imagine I wanna go and meet Jim, and we're gonna go meet in San Francisco, and I would like to meet him, but I wanna meet him at a coffee shop. And so here, I'm gonna ask the agent, hey.
I'm excited to meet Jim in San Francisco. Could you find me a high quality coffee shop near his office? And here, the agent space is smart enough to know that Tamers MDM doesn't know anything about coffee stores, but Google Maps does. And so it can go out and ask Google Maps with the context of Jim's location in San Francisco for what are the coffee stores that would be near the offices, Google's offices in San San Francisco.
And, you know, it's given me a list. I picked the first one. Saint Frank Coffee seems like a good place. And now we can kind of have, the moment of truth where you can bring together the language capabilities of, Google's amazing, models with the context and information from, in this case, Tamers MDM data organized around customers and this Google Maps information.
And I'm gonna ask the system to write me an email from me with context about who I am, what Tamer does, to go meet with, in this case, Jim, but at this coffee store. And what you see when you get back this email, well, you know, you can read it at your leisure. But what you look if you look into the details of this email, it's extraordinarily well thought through. It knows me.
It knows who Tamr is and what we do. It knows Google and what Google does.
And it knows this particular coffee store. And it's bringing all that context and information together and writing a really high quality introductory email, for me to send to Jim with, you know, a specific location and explaining why Jim at Google ought to be interested in meeting with me, from from Tamr. And so, again, I hope what you see, in this this quick little, click through is why it's so valuable to bring data, and contextual information is, agentic experiences so that we can, you know, create outcomes, just like this.
So with that, we're gonna open it up to, q and a. And, yeah. Let's let's, let's jump into that. And, Carol, I think you were gonna help us mediate the, the the q and a.
So many buttons to press. I think I'm off mute. Hopefully, you can me.
Thank you both, Anthony and Jim. So, yes, we'll shift into a live q and a. So if you do have any questions, feel free to drop them in the q and a chat in the panel to your right.
But we'll jump in. So I guess first question for you, Jim. Can you please elaborate a bit on Google's broader strategy and long term vision for agent space?
Yeah. I mean, look, I I think we we believe this is the future of knowledge work.
And so I I think what we've tried to do is provide a platform, that's really secure, that is open to doing a lot of things. And and I think if I were to say I know where this is going, I'd be lying. I mean, it's it's it's moving so fast. And I think what we need to just do is be open and available.
I think that's why I get excited about working with clients like Tamr and, what they're gonna do with it. Because I think I've I've presented this to a number of c CEOs at different ISB and tech companies, and it's kind of like everybody they once they get it, they start to have a new idea of how they can, engage. I think the the interesting piece will be how these agents start to talk to each other. And I think that's gonna be kind of the future of where this goes is that, you know, I I think we'll see everybody building agents this year.
I think by the end of the year, we'll see people using agents, in a in a regular way. And I think at the beginning of twenty twenty six, we'll start to see a separate who's agent's good and isn't. It's gonna be hard to catch up. So I think I think everybody needs to be investing in this, now and, you know, showing up in that way. But I think the folks that are on the on the bleeding edge of this, you know, tamer being one, is already thinking about how does my agent talk to another agent to be provide more value to my end customer.
Great. Thank you, Jim.
I would just add that I think this is very similar to and hopefully that first slide setting up the the big trends here. This is very similar to, you know, being at the very beginning of the digit you know, early digital transformation initiatives where people were moving business processes into digital, or even early, analytic journeys where people were moving and creating more analysis to help optimize process.
You know, this is it's very early days and and and, you know, we probably don't know the half of what we don't know very much to your point, Joe.
Alright. Last question over to you, Anthony. That was a cool example for finding out about a person. What are some other ways you envision team or customers using your agents?
Yeah. So, you know, imagine, you're in charge of, supply chain for your business, and, you there's a, you know, a news event which you worried, would be, would sort of impact your your supply chain.
Supply chain data is probably being held, in some of your ERP systems in terms of what are open orders. You might have supply chain. You may have some document data stored in Google Drive about, you know, contracts you've signed with those, suppliers. And of course, you need to have a a three sixty view of that supplier relationship. So being able to link all of those, suppliers together really understand who is the, you know, who is the supplier that may be disrupted.
Being able to pull all of that together in context of a language model, might allow you to, for example, give yourself a dossier, a sort of summary of what this potential supply chain disruption would do to your known and existing suppliers. And then just to make the example a little more fun, imagine if you're driving to work and you want that read to you or given to you as an audio that you can listen to as you drive into work where it would sort of summarize that information, and give you the context of knowledge and information you need so that when you walk into that, into your office and you can make some phone calls about how to, you know, move, so you know, bring on board or bring online, some new suppliers, then you can you could do that.
Another example you might think about is, imagine sending to every one of your most valuable customers a personalized video which, articulates the products they bought from you and what their next most obvious, purchase experience from you might be. That would require context and knowledge about products, but also your customers.
And also, you know, potentially image data about what products you're offering and things like that. And then, you know, make it into a fun, you know, God forbid, tech TikTok style like video that you could send off to the customers. Those are just some, you know, off the cuff ideas that that might be so I don't, Jim, if you have I don't know. We'll put you on the spot, but ideas.
No. I wanna see your TikToks.
TikTok. No. No. I I think you're right. I think there's there's this, like, hyper personalization that can be created.
And I think that's too you know, when you have these, like, agents that you could build just for you, I think we're gonna have some of that. And so it's, but the data the data is so important. It it has to be in the right context. And I think that's the really interesting piece about leveraging a without context, it's it's it's just not gonna work as well.
But when you give it the right context, these things are so powerful now that you're gonna get the right answers.
And it's, again, hyper, hyper personalized.
Alright. Awesome. We have a couple more questions that just came through, and we do have a few minutes. So next one is to you, Anthony. Exciting stuff, was the opening. Curious in that example of the coffee shop recommendation. Do similar authority relevance, etcetera metrics inform how these recommendations appear?
Sure. In the example that I provided, in a way, what we were doing was linking together two MCP services.
One from Tamer to give context about the person, in that case, Jim, very, of the moment example.
And then the second was Google Maps, which is obviously a very rich source of location information and in particular very good about things like, stores and, you know, that you could have also asked the questions like, you know, is the coffee shop open during the time that I wanna meet? Things like that.
And so, my expectation is, that we're gonna see a lot of additional services being, built and launched, that offer a array of different capabilities.
And really what the agent to agent protocol from Google provides, as extending what's available from MCP, is a way of explaining to the language model what are the features and capabilities of the underlying service.
And so I think this also produces some opportunities from Tamr's perspective in MDM. So for example, in the example I provided, it was really about, giving in in the example context about the person.
But imagine that when I brought up, Jim, I have, a new, a new address for Jim and his address is, incorrect. Actually, as it turns out in the example, his address is incorrect but even that minor point aside.
I might be able to say back to the system, hey, you know, that address for Jim is wrong. The correct address is, you know, one two three main street, etcetera, whatever the right address is.
And that feedback would go into, another m c service container which would accept an update to a golden record.
And typically, in Tamr's case, because it's an update to master data, and it's coming from a user such as myself, it would be put into a queue for a human to review before being written to the golden record.
And, you know, again, you might have some logic around that. Like, if you're a data curator or you own that entity, maybe your rights are you can just go ahead and update the entities. The model could accept that feedback and then write it directly into the master data system.
Or maybe in my case, I don't have that right and it goes into a queue and the curator, approves that change when they see it come into their, into their queue. So, you know, you can imagine, updates like that. You can also imagine thinking about history. So, you know, Jim currently works for Google, but presumably, he's had a long and illustrious career before that and worked at other places, lesser places, of course. And but it might be interesting to say, you know, how what's Jim done over time? And like or think about it from a b to b customer perspective.
Has there been a merger of acquisition for a customer? And being able to keep that contextual history that we maintain in the MDM systems to understand how, the data shifted and changed over time. And that's also, last thing I was gonna say here, opportunity in the context of agents. Because you could imagine an agent working on behalf behind the scenes watching the data change in your MDM system and then alerting you when data shifts or changes that, there's a, you know, divestiture or a merger between two of your customers, etcetera. So I've put over those are a bunch of examples that that address the question.
Anthony, I think that's gonna be a really important piece as this works forward, and that's one of the things we incorporate in engine space too is being able to, like, leverage real time web information versus just whatever your data sources say. And that's, just a new way to keep everything fresh, and accurate. So I think that's gonna be a huge piece for a lot of folks.
Great. Alright. We have time for about two more questions. So, Jim, next one is for you. Could you show us more about how permissions work? For example, can we have different agents assigned to different teams?
Yeah. Absolutely. So permissions would work kind of, in the same way they're set up today. So we kind of adopt whatever permission framework that you're using today. And so, theoretically, you know, when folks would sign in, they're saying permissions would be would be available.
So Great.
Thank you. And final question over to you, Anthony.
Is Tamr an AI driven data pipeline automation platform?
Yeah. So, yes and no.
Which in fairness is a bit of a wishy washy answer. So, the question presupposes that or or imagines a, generally horizontal, data platforming, pipeline, environment. And no, Tamr is not a sort of general purpose data pipelining, system.
Having said that, many data pipelines are used or the the purpose of many data pipelines or some data pipelines is to bring data together from a number of different systems and, build a unified view of, for example, your customer. That's very common, requirement.
In that case, then the answer is yes. Meaning, what Tamr provides is that outcome, which is a three sixty view of the customer, a three sixty view of the supplier, whether it's people or companies.
And behind the scenes of course we use data pipelining technology. We, you know, there's a lot of, technology we're using, behind the scenes to achieve that outcome. But it's not a general purpose generic data pipelining capability. It's built for a specific purpose, and that specific purpose is AI native MDM.
So hopefully that helps.
Yeah. That's perfect.
Thank you, Anthony.
Alright. Well, that concludes our webinar today. Thank you so much for joining us, and we really hope you found this session valuable.
Just a quick reminder as we saw this in the q and a, today's webinar was recorded, and we will share it with you on demand shortly. We'll send out a link so you can rewatch it or share with your colleagues. And before you go, we'd love your feedback.
So you'll see a survey launch just shortly.
If you can take a minute or two to fill it out, this will really help us continue to improve and bring you the content you want to see.
And thank you again so much for spending your day with us. We hope to see you at a future event soon.