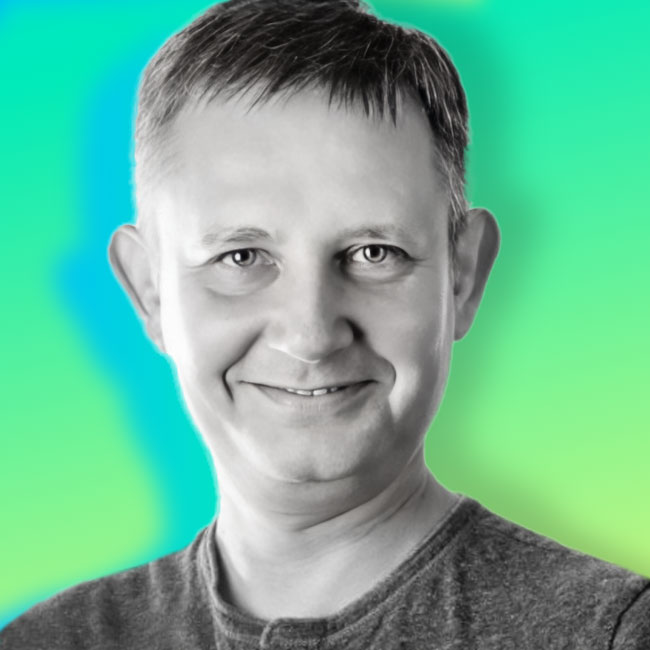
The Future of Data and Analytics Teams: Insights from Misha Advena, Head of Analytics at Miro
Misha Advena
In this episode of the Data Masters podcast, we're joined by Misha Advena, Head of Analytics at Miro, a visual collaboration tool. We discuss the best practices and potential pitfalls for organizing your data and analytics teams. Misha provides valuable insights into the intricacies of managing data and analytics teams, highlighting the shifting paradigm toward a greater reliance on data product managers and a reduced emphasis on technical expertise. His expertise and experience offer a unique perspective that can significantly influence the future of your organization.
Join us for this insightful episode as we navigate the ever-evolving landscape of data and analytics teams with Misha Advena. Gain a deeper understanding of the strategies and approaches that can shape the future of your organization, ensuring it remains agile and data-driven in an increasingly complex environment.
About Miro: Miro is a visual workspace for innovation where teams manage projects, design products, and build the future together.
I'd rather read the transcript of this conversation please!
Intro - 00:00:02:
Data Masters is the go-to place for data enthusiasts. We speak with data leaders from around the world about data, analytics and the emerging technologies and techniques data-savvy organizations are tapping into to gain a competitive advantage. Our experts also share their opinions and perspectives about the hyped and overhyped industry trends we may all be geeking out over. Join the DataMasters Podcast with your host Anthony Deighton, Data Products General Manager at Tamr.
Anthony Deighton - 00:00:38:
Welcome to another exciting episode of DataMasters. Today's guest is Misha Advena, head of Analytics at Miro. Miro is a dynamic online platform that empowers teams of all types to unleash their creativity and drive innovation through visual collaboration. With an impressive track record, Misha brings a wealth of experience to the table. Prior to joining Miro in 2019, he served as the Head of Analytics and Strategic Finance at Segment, the CDP, and played a pivotal role as the director of product Analytics at XTime, and demonstrated his strategic acumen as the head of strategy and planning at Ingecape. It's great to have you here today, Misha.
Misha Advena - 00:01:19:
Great to be here.
Anthony Deighton - 00:01:20:
So maybe we can start a little bit with just some background on Miro. I think many people are familiar with Miro. Probably many people are users of Miro, as we are here at Tamr, and I am personally. But maybe talk a little bit about the Miro solution. And then I think people might be slightly surprised to know that Miro has a head of analytics and that Miro thinks about analytics and data. And so maybe talk a little bit about how the organization views analytics and data as part of its solution.
Misha Advena - 00:01:52:
So Miro, you probably know what it is. It's an online collaborative solution. Our goal is to unleash potential and make collaboration easier and help people to generate, share, discuss ideas in a way that's natural. Because we have a lot of tools, but the previous generation of tools was kind of limiting people like documents, all those spreadsheets. And essentially Mira is this Infinite Canvas, which can be used for putting different types of visualizations, data, insights in a way that works for people. And it's collaborative. So people can do it together. Analytics, I feel extremely like it to be part of analytics at Miro because if you think about it, like what analytics is doing, right? Like it takes data and tries to make sense of it. And it tries to have a business impact using it. And like there are two factors there. First, you need to have data that's meaningful. And second, you need to have people who are willing to listen and engage with you and take your insights and try them in practice. And in these two dimensions, we are extremely like it because if you think about our product, essentially, we know everything that our users are doing. So all value creation, all things that people are doing in the product, they're all sort of all. So we have a ton of data about what people do, how they do it. And we have data that has potential to unleash next level insights. On our stakeholder side, it's the most data-driven team that I have seen in my life. It's both a blessing and the course blessing because people really want our input. People really want to make decisions partially based on data and on data insights. It also means that they're extremely demanding. So people listen to us, but also have so many questions that they want answered.
Anthony Deighton - 00:03:46:
Yeah, so I think that's fascinating in a sense, the product that you are, that Miro's building, being a virtual whiteboard sort of disrupts the whiteboard market in the sense that whiteboards that we might have in our traditional offices with those pens that never seem to have ink, nobody has any idea how people use them. But in your case with Miro, people, you and the Miro team have just an incredible volume of data about how people use whiteboards and how they collaborate on them, et cetera. And so I'd love you to share a practical example of how data and analytics, how the analysis of that data changed the way Miro thought about how people use whiteboards or had an impact on the product direction.
Misha Advena - 00:04:37:
So that's what we try to do every day. I'll give you a lot of examples. I'll start from simple examples and feel free to add more questions. So basic question, we have a huge product team, we have a huge engineering team, which features do we prioritize? And I'm not claiming we have an answer to that, but we are trying to support the process. And the first step is always, okay, there is a feature, can we measure whether people are using it? Yes or no? And that's already by itself not trivial. You need to track events in a way that allows you to calculate usage. You need to be very clear about what usage means, what kind of data is needed for that. Foundation, just like telling our product people which feature is used, which is not used and starting it as a baseline. Next step is, okay, people are using features, but does it really create value? And that's tricky, right? Because how do you know? There is no way of knowing. Our way of looking at it is, we have our North Star metrics, we have different levels of usage. We have monthly active, weekly active, engaged. There are so many, there are a lot of metrics of engaged usage. And then our attempt is to say, okay, now Feature A was launched, does it really result in better usage in general? And relation, causation is always a problem, but our goal, again, starts from understanding do people use Feature, yes or no? And if they are used, does it have an impact on general usage? or something.
Anthony Deighton - 00:06:08:
Is there an example of a Feature that either had unexpectedly positive impact on usage and engagement or maybe even more interestingly, unexpectedly poor, something that the team intuitively thought might be really successful but in fact was not?
Misha Advena - 00:06:26:
So it happens all the time, usually it's later because people in product, they are optimistic by nature and they just like optimistic about how amazing Feature A is going to be and rarely happens that a thing becomes way more successful than they should be or that projected to be. In real life, it's not yes or no answer because in real life you do something and then you look like what worked well, what didn't and then you iterate and you try to understand what went wrong because like only after a few iterations, like you are in position to decide whether it's really working or not. One in my, during my life at Miro, there was one moment when things just grew faster than anybody could have ever imagined. I know they want to guess what was driving that. I'll tell you a graph, like a usage graph, like it was like this, it was like growing kind of 10, 20%, I know like quarter and then it doubled in a week and then it doubled again. Do you know when it happened?
Anthony Deighton - 00:07:26:
I can guess the moment at which everybody is forced to work from home, that they can't gather around the proverbial whiteboard. I'm confident that I was going to ask you about the pandemic, but I thought maybe just.
Misha Advena - 00:07:39:
It was more scientific. Yeah, you're absolutely correct. So like we have this like joke, internal joke that like, like apart from another pandemic, like is there anything else we can do?
Anthony Deighton - 00:07:50:
So yes, the conspiracy theories that Miro calls the Pandemic, I'm sure are rampant. So I was going to ask in the context of the Pandemic, you know, so tracking features and understanding how people use the product during normal times is one thing, and then you get put into a situation where the Pandemic drives significant usage, but I also wondered coming into this, whether the Pandemic had unexpected, other than simply driving additional usage of the product, whether other features or capabilities, it changed the strategic direction of the company. Again, other than the obvious, you just needed way more capacity to support way more users in the product, but did it change the way people collaborated virtually?
Misha Advena - 00:08:36:
Of course, the answer is yes, because if you look at the way people collaborate, you have synchronous, asynchronous mode. And synchronous, essentially people doing things at the same time, asynchronous. You build the board and then somebody else reviews it in some different time. Once pandemic happened, because now people are working from home, asynchronous collaboration increased.
Anthony Deighton - 00:08:58:
So what I hear you saying is that after the pandemic, Asynchronous Collaboration became a bigger part of the usage of the platform versus synchronous. As I think about it, might be counterintuitive, logic being when more people are stuck at home and the only mechanism of engaging and interacting is virtually, the idea that the more synchronous or virtual whiteboard use as a proportion of total usage might be what I would have guessed. But you're telling me it's actually the opposite.
Misha Advena - 00:09:30:
Yeah, like these questions are complex and difficult to answer because like when, what's happening in real life is that you have usage patterns, which is one dimension. And then you have the composition of your customer base. And in real life, things are happening at the same time. So usage patterns are changing, but the mix of customers is changing as well. So kind of a great example, like depth of usage, like what percent of people are using things like once a month, four times a week. Like we really hope and we really try to drive more in depth usage for customers whom we have. But at the same time, when you are growing, you have a share of new users who by definition will use products in a lighter way, at least in the beginning, it's growing. So yeah, it's like things are shifting and changing at the same time and we kind of want our goal to be the solution of choice. Like we really want people to use our product as much as possible. But also what it means, it means that if you have one person in an organization who starts using Miro for a lot of rituals, now you start having other people who are, to whom this presentation got sent or people who are attending meetings that are using the board. So you have one person who is using the product in a more deep way, but like this person through virality adds new users who may be at least in the first stages just like viewers and interact with the product in light.
Anthony Deighton - 00:10:57:
Yeah, interesting. And again, in the context of a pandemic, where you're seeing explosive growth, the proportion of light new users is probably much higher because they're just simply adding more users every month. So we've talked a little bit about the role that analytics plays within Miro. Maybe, good share, you have a long history of building analytics teams and helping to support decision makers within an organization. I suspect many listeners are in a very similar role. And there seems to be this perpetual debate within the community about how to organize analytics teams, whether they should own and control the data layer, whether that should be separated, whether they should be part of IT, whether they should be separated from IT, whether they should be in with decision makers, whether they should be a central team that's outside of decision makers. Maybe share a little bit about what you've seen work well at Miro. And to the extent that that's changed, I'd love to hear a little bit about how it's changed.
Misha Advena - 00:11:57:
It's a great question. I don't think there is like one answer for it all. It really depends on the stage of the company. And I've observed this thing to be changing over time. So like one example, now a lot of organizations have a whole role called analytics engineering. I think when I started in analytics years ago, this role didn't even exist. Sometimes you have data engineers, but analytics engineering is this kind of pretty new concept that found a lot of success because people found that those talking about our organizational structure. So we have a data stream, which combines data engineers, Analytics engineers. And their role is to figure out what are data sources and then get data from data sources and then build data models. And then once data models are in place, now it's when Analytics come in and try to leverage those data models to report on the set of metrics that we have and take those insights. And our goal is that our mission is to drive better decisions using data. Our data stream team is central. Our analytics team is centrally embedded. And central means that we are one big team. We are all under one roof. Embedded means that teams consist of functional teams focused on supporting their center. marketing and analytics. sales analytics, finance analytics. And it's a balance. It's a balancing act, right? Because for an analyst to be successful, analysts need to be extremely close to their stakeholders. They need to understand what it is like, what this team tries to accomplish. What are their challenges? And then use metric, metric analysis insights, data insights to be a good partner and to find a way to help them. And how to do it is extremely stakeholder specific. At the same time, you want to have some consistent way of doing things, right? Like how do we define metrics? How do we build boards? How do we build data models? When you build a data model for your stakeholder, how do you make sure that people in another team can use it as well? So you need the central component that provides the guidance and that provides some kind of strategy vision, operational cadence that aligns the way we do things and that allows people to learn from each other and to collaborate and not create silos because silos is just like the one thing that everybody wants to avoid. You don't want to have two reports that report on the same thing and have different numbers, just like a recipe for disaster. So this is how we organize it today. Common wisdom is that with size, kind of usually people start from a central, central team, like when you have one analyst, they add everything. So like in smaller companies, usually it's one central team. Then when you get bigger, you start creating some teams and like when you grow further, the need for functional expertise becomes more and more and teams tend to become heavier on the functional side of things and lighter on the central. In my head, it kind of, again, it really depends on organization, on kind of business decisions we need to report. It also depends on people because at the end of the day, kind of, right? Like, you want managers to be strong and to be able to support their analysts, but you have only one, like if you have two people in marketing analytics, it's impossible to have one person to be a strong manager because strong managers want to manage bigger teams. So it's always balancing acts between what is needed for the business and based on the size, what structure can get you to look result in most of the
Anthony Deighton - 00:15:32:
That makes a lot of sense and I appreciate you started with this idea that there's not one way. But let me force you to take a stand. If there are many good ways of organizing an analytics team, what are some really bad decisions that either you've seen or forbid that you've made? What are some common mistakes you see in organizing data teams that listeners should avoid? Like learning from prior mistakes.
Misha Advena - 00:15:59:
A lot of mistakes were made in my life. So that's definitely true. So concepts of silos, like my one, talking about like, it's like overweighting functional components and not investing enough in central components is one way to get into trouble. And a potential problem I definitely face is that if you have super strong functional teams, but not enough effort into building a central kind of foundation, you start creating silos, you start seeing different numbers, you start seeing two teams working on the same thing or nobody working on something that's individual. If you are too central, also a mistake that was made a few years ago. If you are too central, your analysts become too far from real stakeholders. And they become like, they start perceiving their work as building a task work as opposed to driving business decisions, which is a very wrong mindset. And it's just not helpful. But like when you are central, when you are not part of a team, when you're not attending their meetings, when you are not having lunches with them, it's just so easy to become isolated. So both things have pros and cons and finding trade balance is super important. Relationship between direct team and analytics, again, like an extremely tough one, right? Because you want teams to be as aligned as humanly possible because we all know that the people are more technical, they love building technically sophisticated solutions. Analytics are more business focused. They want something that may be not as technically sophisticated, but like way faster and way more flexible so they can accommodate their state stakeholders. So you want these to be as close as possible so that roadmaps are aligned. At the same time, data teams need to be more technically substituted and they need to be closer to engineering because a lot of data is generated in our production system. So it's difficult. It's a challenge.
Anthony Deighton - 00:17:52:
100% agree with what you just said, but I would add to it that another challenge and distinction between business stakeholders, analytics teams and data teams centers around the accuracy and reliability of the underlying data. So the common question is, how do I bring in another source? How do I... this data is incorrect, like clearly wrong. This data is missing. These sorts of questions flow, if you will, from business stakeholders through analytics teams, maybe down to data teams if there's actually an underlying data problem. How does your team think about ensuring the accuracy and reliability of data? How do you think about managing that data and decision making process, in a sense, like a product? And think about getting feedback and then improving. And by the way, it's not just about the data. It could also be around a metric. You might say, we've been calculating, you know, we want to move from weekly active users to daily active users, or we want to change the definition of a week, or these sorts of questions. So what do you think about that feedback process and that management process?
Misha Advena - 00:19:01:
Well, I think there are two questions there and they're different. We really need to look at them separately. First one about the quality of data sources. And again, my favorite saying is that if you talk to a data person and he or she tells you that their data quality is 100%, you're like, that's a liar. Don't trust that person. That never, I never have done that in my life. I don't think you can talk about that. And the reason it's impossible is that if you think about people who generate data and people who use data, like those people are very different. And people who generate data, usually data is like one of the outcomes of what they do. For example, data from salesforce, right? It's entered by sales people. Sales people are hired not because they have amazing skills in recording everything. They are hired because they are good Salesforce people. They have to enter data. So like there needs to be a process to make sure that data that they enter is not horrible, will never be 100% correct by itself unless there is a very sophisticated process of control and of making sure that they do what they want to do. Similarly, like a product, right? Like events, we need to implement events. But like when product people are building features, defining events, and for engineers implementing events, it's like, I know, like at the end of a very long list of things to do. And it will never naturally get prioritized. And it doesn't have to be prioritized because its quality of data tracking is priority number one for your product people. It means that they are not thinking about customers, which they should be doing. So kind of it's finding a balance between getting data that you're really and not kind of killing everybody else around you in the process. And it's impossible to solve problems. We definitely have it. Like, well, I think this thing goes in circles. Like you do something, like it gets good enough, you start using it. And they're like, oh my God, no, actually, for what I needed and we'll go over again. The thing that makes this process extremely difficult, like that's my current opinion, I know maybe it will change, is that like to make right decisions, you really need to connect what business stakeholders need from your insights all the way down to what does it mean for metrics. sources of data we want to track. And it's like, essentially, everybody in an organization is involved. So it's like five teams involved in that. And you need essentially, like you mentioned data as a product, if you treat data as a product, you need to have a product manager who can put this all together. Because like at the end of the day, it will always be about compromises, like you will never get all the data because otherwise it's too expensive to like, take too much time. So you want to get data that you need. while not getting data that you don't, but you don't know which data you need in the beginning, because it will be iteration. And creating this end-to-end alignment between teams is super difficult. And it's a skill set that nobody has. It's not an analytics skill set. It's not a data skill set. It's like project management, project management. And those people are first, extremely rare. Second, if somebody can do it that well, that person usually produces. So yeah, it's in real life, whether you hire somebody or you just like to take somebody who spikes on the skill set and ask that person to do it. And that can be extremely impactful, but those projects are very painful. Coordinating a lot of people is difficult.
Anthony Deighton - 00:22:35:
I think the point you're making there is really insightful. This idea that at its core, the challenge of managing the connection between business stakeholders all the way back to data people who are engineers that are building products and generating this data or salespeople that are entering it to your point. Ultimately, that flow feels a lot like the flow for building a product, building a product feature. And the other important point is there's no such thing as perfect. So while we might think that data is this pristine thing, that, you know, beautiful and perfect, the reality is it suffers from the same trade-offs that any product feature or any capability in a software product suffers from. And so the same techniques associated with managing them can be very effective. Would you agree?
Misha Advena - 00:23:23:
Yeah, absolutely. Anyway, one way to describe it is that... Like we hire people into analytics. frequently based on their technical skills. Like you need to write SQL, you need to know GPT, you need to be able to write, I don't know, like, look-a-mill things. But like when we ask a question, like, what really makes a difference? Usually it's the product management mindset. But like we hire people for X and then we ask them to do Y and then we get surprised that things don't go as planned. I think you described it super well. Like if you think about analytics, essentially it's a proper EPD organization. You need to have product people who will connect the dots. You need to have... engineering people are psychology, not engineers. And then you need to have, I don't know, people who build solutions that can be consumed. So essentially, you need people who can do it all, who are technical enough, who can write code, who can build beautiful visualizations, who truly understand the stakeholders, who can take insight and present it in a way that your non-technical stakeholder can understand. And you need people who can think that like, okay, we have data, this is the result. It's never conclusive, it's never black and white. It's kind of like this, but they need to be able to have a point of view and have a way to combine data that we have with context that stakeholders have, and then connect the dots and like make sure that decision that people end up making. It's not necessarily the correct one, but like it's more likely to be correct than not.
Anthony Deighton - 00:24:54:
So I'm going to challenge you to cast your eye forward a little bit, predict the future, which as a data person, analytics person, you should be always very wary of doing. But I am curious, and you make a really important point here, which is that the perfect person on an analytics team is an odd combination of technical, business-oriented, product-oriented, et cetera. But cast your eye forward a little bit in thinking in the context of how data and analytics is changing. What's your prediction for the future of analytics? What should we expect? And that way, I think, what should listeners be thinking about planning for in the future?
Misha Advena - 00:25:33:
My best guess is that we'll see way more product managers, people being extremely successful in Analytics, and the role of technical expertise will go down. And the reason for that is that technical things you can buy. You can outsource, you can buy snowflakes. When I started analytics, it was oracle. We had database people who were building databases. Not anymore. You have solutions that manage solutions that work pretty well. And technical problems. Like you have a bunch of API's, a bunch of Vendors who have solved them. Because like that, similar people tend to use the same systems. So just like all this technical complexity of artist training data. I think it will get more like you can buy this, but your business is unique. Kind of business challenges your company has today are extremely unique. This one you cannot buy from your vendor. You need people who will be able to understand what those challenges are. And we'll be able to use data and tools that we have to be able to solve this and this is more product manager, flash consultant slash I don't know how to call it mindset versus somebody who is extremely great.
Anthony Deighton - 00:26:45:
And I think that's a great insight in that the general trend you're illuminating is this idea that technology gets out of the way, that the role becomes less technical, less about coding, less about the infrastructure, less about the mechanics of it, and more oriented towards these product management skills of being able to align the kind of work we're doing analytically to decision-maker business people. And at its extreme, and let me push here for a second, could you imagine a scenario where the decision-maker themselves, you know, is ultimately just asking and answering questions of the data and all the technology behind that is essentially automated, or is that too far?
Misha Advena - 00:27:32:
You can abstract away building blocks. Somebody needs to decide which building blocks you need. So kind of... like in the ideal world that you are describing where it's fully self-service, like first, like somebody still needs to design the underlying foundation. And so that's like role number one that probably is here to stay for at least some time. And it's actually super complex. But secondly, like the way we can create value, it goes back to my comment that the answers you get from data are never white, black and white. It's never like going that way. It's always, it kind of goes this way. But like last month, it was different and it's not particularly significant. And by the way, like it's super volatile. And like you need somebody who can interpret data in a way that's actionable while still keeping like intellectual honesty and intellectual rigor. Or because otherwise, like if you don't have a point of view, if you are not ending your analysis with a test, okay, and it means you need to do A, it will not be impactful. But if you are not rigorous enough, then you are just not capturing the value of data, then it becomes just an opinion for a person who likes will have opinions. So kind of there is a value from being knowledgeable enough about what data means, how it gets collected, like what it truly says and being able to breach all this data variability and noise to get to the right signal.
Anthony Deighton - 00:28:59:
Great. So, Misha, it's been a pleasure. Thanks so much for joining us on the Podcast. We really appreciate the insights you've shared and wish you the best at Miro. I'm looking forward to jumping on a virtual whiteboard with some colleagues probably in my next meeting.
Outro - 00:29:18:
DataMasters is brought to you by Tamr, the leader in data products. Visit tamr.com to learn how Tamr helps data teams quickly improve the quality and accuracy of their customer and company data. Be sure to click subscribe so you don't miss any future episodes. On behalf of the team here at Tamr, thanks for listening.