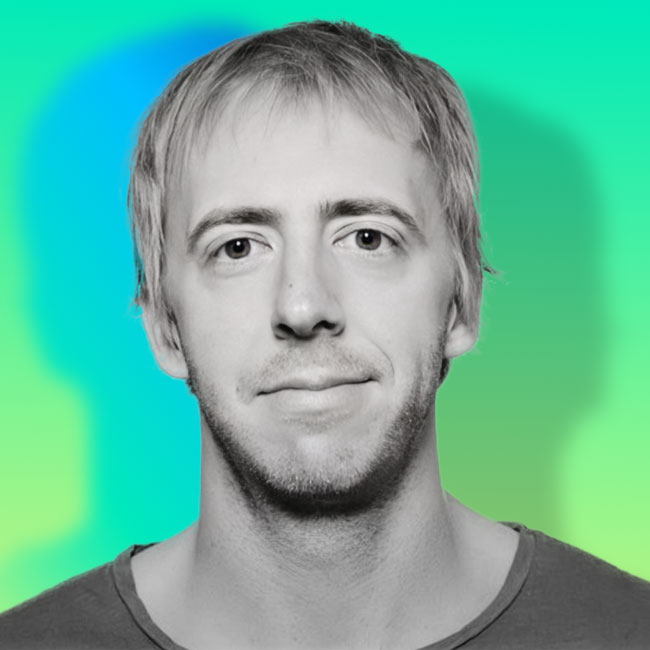
Mode Analytics' Benn Stancil on Data Collaboration
Benn Stancil
In this episode of the Data Masters podcast, we’re joined by Benn Stancil, co-founder and CTO at Mode, who zeroes in on collaboration in data to challenge prevalent notions of what successful collaboration in this field looks like. Benn debunks the misconception that effective collaboration in data and analysis is merely about mirroring the functionalities found in popular tools like Google Docs. He asserts that the cornerstone of successful data collaboration is the ease with which collaborators can understand how a dashboard or data visualization was created, updated, and evolved over time. This understanding enables collaborators to efficiently follow the workflow and engage with the data's history, facilitating smoother and more productive collaborative efforts. Furthermore, we delve into the dynamic nature of collaboration, illustrating how it adapts depending on the specific type of work at hand. Tune in for an insightful discussion that unveils the true essence of data collaboration.
About Mode: Mode is the modern business intelligence platform that unites data teams with business teams to build analytics that drive business outcomes.
I'd rather read the transcript of this conversation please!
Intro - 00:00:03:
DataMasters is the go-to place for data enthusiasts. We speak with data leaders from around the world about data, analytics and the emerging technologies and techniques data-savvy organizations are tapping into to gain a competitive advantage. Our experts also share their opinions and perspectives about the hyped and overhyped industry trends we may all be geeking out over. Join the DataMasters Podcast with your host Anthony Deighton, Data Products General Manager at Tamr.
Anthony Deighton - 00:00:38:
Welcome to DataMasters. Today's guest is Benn Stancil, CTO and Co-founder at Mode. So welcome to the conversation, Benn. I thought maybe we could start a little bit with your career history and trajectory as you came into founding Mode. You started your career at Yammer, which was quickly subsumed by Microsoft. And I think you had some pretty different roles there before jumping in and starting Mode. So maybe just share a little bit about the founding story and how you found yourself at Mode.
Benn Stancil - 00:01:12:
Yeah, for sure. So as you mentioned, I started my career in tech at a company called Yammer. Prior to that, I worked in DC in a policy research role, very different than things that go on in tech, but ended up in tech at Yammer. Was on a data team there that was one of these early incarnations of what has become a modern data team. So it wasn't a BI team, our job was not to produce dashboards and reports, but we also weren't a capital D data science team that was responsible for building models that generated predictive algorithms for products and things like that. We were something, we were basically sitting alongside folks in the business. They're trying to make decisions about which marketing campaigns to run or which products to ship, which strategic decisions we should make as an executive team. And our job as a data team was basically to advise them on those decisions or to give them support around here, what the data says about how different campaigns are performed, about how these products are performing with users, about what those behaviors might suggest about what we should be building in the future, things like that. So that team, because we weren't just building dashboards, but because we also weren't doing deep technical work, we actually didn't have any tools that worked well for us. We ended up in this middle ground between the traditional BI players like Tableau or MicroStrategy at the time, where it wasn't just about building these dashboards, but also we couldn't use the technical tools that were designed for proper data scientists, because even though we knew some of those languages, like we had folks on the team who were very comfortable on Python and R and things like that, we couldn't send Jupyter Notebook or an RStudio file to the executive team. We couldn't be like, hey, CEO, here's an R Markdown file. This is their answer to the questions. Like, please don't give us this. We need something we can actually consume, give it to us in a deck. And so we ended up doing a bunch of work in tools that were designed for us, and then having to translate them into tools that were designed for other people in the business more easily to consume. And so we ended up building internally was a tool that bridged this gap, where it allowed us to work basically by writing SQL queries directly on top of our warehouse, and then easily put charts and things on top so that we can work the technical flows that were the ones that were more comfortable to us, and the rest of the business could basically consume them in the way that they wanted to, and these nice layouts and things like that. And again, the point wasn't to build just dashboards. It was like, be collaborative with them, answer questions, help this back and forth that goes into us trying to actually help them out with what they're trying to do. And so we saw that basically it worked really well at Yammer after Yammer got acquired by Microsoft. That product started to spread inside of Microsoft. We realized there were a bunch of other companies that built similar sorts of tools. So versions of these existed inside of Uber, inside of Facebook, inside of AirBnB, inside of Pinterest, Spotify. They all had these same query tools designed for data teams to solve exactly this problem. And so in seeing that, we basically realized that there was potential value in building a product for this type of data team. And again, it wasn't just building dashboards, but they also weren't doing what was at that point, the very popular like data science predictive, like the magical work, but was doing a little bit more of just sitting alongside the business, helping them answer questions. And so really we set out for Mode to solve that problem and saying, hey, what if we build a tool that is designed for a data team, but recognizing that their job is to work with everybody around the business, instead of their job is to just do interesting analyses and ends up sitting on their computer and slowly going stale.
Anthony Deighton - 00:04:21:
Yeah, and so one of the challenges in thinking about starting a business like this, especially when you did, which was nine years ago, is you're entering a space that's relatively crowded and listeners may or may not be aware, but my history is I spent many years at a company called Qlik, which I think reasonably would be considered to be in the space. And today, you have Qlik, you have Tableau, you have Power BI, and so it's crowded in an odd way, crowded with some 800 pound gorillas. And so that makes it an interesting or challenging place to launch a startup. I'm curious how you thought about that at the time and how you think about it differently today.
Benn Stancil - 00:05:00:
So at the time I would say there's a couple reasons why we still, despite, there were certainly a lot of players in the space that are more naïve why at the time, you know, we felt like this was a thing to do. One reason, and this is probably true for a lot of startups is like you for naivete is some idea that basically, Hey, you know, every tool has their problems, Mode certainly has its problems, but every one of these other tools has their own problems and some belief that you can build something that's better. And in those cases, there were a couple big changes that were happening that we were in the midst of that we felt like we could take advantage of, like the cloud being the most obvious one that we started motor around the same time that Redshift started to become popular. And so there was a big shift from data tools being very much oriented around on-prem warehouses, people being very particular about data security and things like that to still being particular about data security, but being much more comfortable with doing that in the cloud. And so we felt that there was an opportunity to build something that was more cloud native instead of trying to, you know, a lot of these tools were going to try to move to the cloud. That was a notoriously hard thing to do. And so we felt that there would be an opportunity to, Hey, we're going to build a cloud first version of this. That also opens up other markets where it's not just saying like, hey, you know, the big players are going to want to, it's not that boeing is going to want to go out and like, Oh, we need a cloud tool. We're going to go buy it. It's more that these tools were becoming increasingly less expensive. So what used to cost a million dollars a year to run like a Teradata warehouse, you could now pay for one in Redshift for a thousand bucks a month. And so now there was to be a lot more interest in being able to use tools that could sit on top of those things that we felt like we could take advantage of and providing cloud software, give us the economic structure to basically be able to do that. The other thing that we thought was basically like those tools were for a, I don't want to say dated, but like a different version of the way that data teams were constituted. They were very much designed around the idea of reporting, dashboarding, that kind of stuff. What we increasingly saw were these teams that jobs were to be kind of strategic, that they were supposed to be, that they saw dash boarding as a necessary evil, but wasn't what they wanted to actually be doing. They wanted to be doing things where it's like doing more impactful analysis and those kinds of things. Now, I think 10 years into it in, you could talk about whether or not that actually has panned out, whether or not there's actually been like how much have data teams really delivered on that promise of being these like great strategic advisors and help you make decisions and things like that versus getting out of some of the dashboarding that they typically do. Like there still is a lot of dashboarding, much more so than I think people would have thought there would have been 10 years ago, given the trends we were on then. So, you know, to what extent has that worked out overall? I think data teams are still trying to figure some of that stuff out. But that was another thing that we really wanted to push into was how to enable teams to do more than the traditional reporting and BI type of thing they had done before. So actually, back in the very early days, we didn't see ourselves as a BI tool at all. We tried to like, you know, we tried to avoid that characterization. I think over time we came to realize that all of these things are BI and one form or another. They just take different cuts at it. And so we're much more comfortable with that now. But yeah, I think that at the time we saw it as more of a data team productivity tool as much as a traditional dashboarding API tool.
Anthony Deighton - 00:07:58:
Yeah, I think this challenge of the dashboard is, and even when frankly I think Qlik struggled with, there's the sense that dashboards aren't enough. And also the sense that the data behind the dashboard is the thing. And it's the thing that often people struggle with the quality of that data. So they're struggling with it, they see the data in the dashboard and they're like, well, this is wrong, this column is wrong, these records are clearly duplicates. It's a problem, clearly, Tamr's trying to go after. So I used to say this, the most popular use case for these tools was as export to Excel. So people would go into Qlik, they would do a bunch of work, and then they would dump the data in Excel, often to fix it up, often to repair errors in the data, but also sometimes to mash it up with other data. And I think we struggled a lot with trying to think about how to, in a sense, avoid exporting to Excel as a way to sort of, the theory being that if you keep people in a tool, that's better. I'm curious how Mode thinks about that. By the way, as a slight joke, I used to say that every time someone clicks export to Excel, an angel loses its wings. That was the internal, and as a way to get people motivated to solve the problem. And I don't think we ever did, truth be told, but I'm curious how you think about it.
Benn Stancil - 00:09:10:
Yeah, I mean, we had a similar feeling that Node's second most popular, Node's like a query, like it has a thing that connects to databases. You can run queries, build charts, things like that. Node's first most popular feature is connecting to a database because you can't use it unless you do that. Its second most popular feature is export to CSV. And like we had some of the same anxieties, I would say about that, where it's like, this feels like people are ejecting from the product. Clearly we are doing something wrong here. How do we like to keep people in it? I don't think we've really solved that. I don't think anybody's really solved that. I think this is a little bit of an unanswered phenomenon, honestly, in the data space where everybody has kind of the like, well, you want to be able to play with it. You want to be close to it and tweak it and all that. And no matter what, you can't keep people in it. No data tool seems to be successful in doing this. So our view has been like, we have gotten a little bit past the idea of thinking that that's necessarily a problem, that if we are a path to people needing to get something to Excel and that's what they're going to do, okay, that's fine. So long as we can be on a fast path for that. My hope is over time that we eventually figure out what it is that that secret sauce inside of Excel is that nobody seems to be able to escape. But yeah, I think we have struggled with this or had the existential worry of like, what is it that we're doing so wrong if everybody just wants to use Excel? Don't people hate Excel? I'm like, people complain about Excel, but everyone's use Excel. And so I don't actually have a great answer as to what that is. We all have like our half dozen theories as to what are the things that make Excel so popular. But I do think that there is something we still haven't figured out there.
Anthony Deighton - 00:10:37:
The other thing that I noticed as I look at Mode, and maybe this is a bit from the outside, I'm curious on your reaction here, but the idea of collaborating around data, and maybe this is part of your background from Yammer and thinking about how you create a collaborative space for people to interact and comment and that sort of thing. It does feel like the idea of working together with, I mean, Excel by its nature is a single-user tool. Maybe Microsoft would argue differently around Office 365, although it's window dressing at best. But anyway, it's very much a single-user tool. But this idea that we all want to work together with data seems very intuitive and seems like a place of maybe a fissure that modes picked off. Is that fair?
Benn Stancil - 00:11:24:
We have done some work on that. And I think one of the things that tricky parts slash like interesting parts about this is what does it mean to work together on data versus something else? I think that it's not clear exactly how to collaborate, I think it's easy for people to think that collaboration is comments. And it's not exactly clear to me that that's or like co-editing in the Google Docs style thing. I think these things all have those different collaborative modes. Basically the way that people want to collaborate on data and analysis is different in the way they want to collaborate on putting together a doc versus collaborating on a PowerPoint versus collaborating on something like a design and something like Figma. And so I think that a lot of the success or failures in the collaboration angle to data tools is less about, you know, do we implement comments in the right way, but more about, do you actually identify the places that people want to be collaborative? Like, do you figure out the things that are actually the places that people are trying to collaborate versus just tacking on the stuff that we assume to be collaborative? And so honestly for us, the thing that we felt was the biggest pain point in all of this and the thing that prevented most of the collaboration that we wanted to have when we were at Amherst or in the early days of Mode was less about like commenting and more about just people being in the same space. It was that data teams worked in one tool, packaged it back up, shipped it over into another tool. And so there was this very slow collaborative loop where it was, we would do work, we would send it to something else, we'd have to take screenshots of stuff. They would ask questions. You'd have to go through this process of recreating the thing. If you sent it to somebody else who wasn't the original creator, they would be like, I have no idea what this chart is. I'm going to do the thing where I like to measure the number of pixels between these dots and try to figure out what that number was. You know, like the screenshot of the PDF. And so it was more about avoiding those things than it was about commenting. And so that's, to us, the key part of it was how do we make sure that people can work on the same surface? How do we make sure that when a business user looks at a dashboard and they have questions about it, that the people who created it can very easily see how it was created. They can very easily update it and follow the flow of what it was. They can follow the flow of how it actually got to the place that it got there. They can see the history of it. It's those sorts of things to us that are much more like what is important for collaboration and data as opposed to, you know, Google Docs style editing. Like, sure, do you want to co-write a SQL query? Probably not. Maybe there's some use for that. Like it's a little bit more like a training tool. But yeah, the nature of collaboration is just very different depending on the types of work we're trying to do. So our approach to this was much more, it's actually about connecting all these pieces together than it is to mimic what say Google Docs does for Word.
Anthony Deighton - 00:13:56:
I like that idea of the common surface, this idea that you're working in the same playground or on the same surface or on the same sheet of paper, maybe you're doing different things. I think your point about co-editing a SQL statement is very apt. I mean, maybe two developers might do such a thing, but the idea that I might write a SQL statement and you might interrogate the results of that and create a tight feedback loop between those two things does feel quite reasonable.
Benn Stancil - 00:14:21:
There is something interesting in that, that even on the SQL side, one of the things that we saw early at Mode, that we did not expect at all, and this has been one of the very unexpected successes honestly in the product, is when we first built it, we expected it to be something that would be closer to like a Figma type of product, where you'd have very clear people who are the creators, in their case designers, in our case analysts, who are writing SQL queries, and then you have people who are just consumers, and you don't really have that much overlap. And so we would sell to the data teams, they'd be creating stuff, everybody else would be these passive consumers or would tweak parameters or filters, but wouldn't actually go in and write anything. And one of the things we realized actually pretty quickly was because we were saying, here's a chart and here's a SQL query that's behind it. Like SQL is relatively straightforward to understand. Like if you don't understand it at all and you read some big complicated thing, But if you see a fairly simple query that's got like where the date is greater than 2020 and less than 2023, like you can figure out what that means. And people did and they'd be like, oh, I can change this. And I bet I know it will happen. You know, this currently says where an account ID equals 10. If I change it to account ID equals 11, it seems pretty obvious what's going to happen. I'm going to do that. And so we actually saw a lot of people who were not technical or not people who saw themselves even as people at Comfort Writing SQL using this as a way to actually teach themselves how to do it and gradually becoming these pseudo analysts where they weren't necessarily trying to write SQL to do a bunch of analysis, but they could read it well enough to be able to make tweaks. And so again, that type of collaboration was somewhat unexpected where we basically realized if we put a bunch of dashboards and charts in front of people, but showed them how they were created, people were curious enough. They were very capable of reading it and being like, oh, this is a good way for me to learn what I'm going to do. And so that type of stuff actually made a big difference too, where it wasn't even just like, how do you make them put them on the same surface, it's actually putting the two things side by side. A lot of people started to like teaching themselves things that they wouldn't have expected to teach themselves otherwise.
Anthony Deighton - 00:16:20:
Cool. So shifting gears slightly, a question I've been asking lots of people, it is very clear that in the public zeitgeist, this idea of ChatGPT has entered our vernacular, kids are using it for studies, etc. The general underlying concept of generative transformer models and Large Language Models itself is interesting from a machine learning perspective. And it would seem to me if one of the challenges modes working to solve is the interface to data. This could be quite relevant and interesting. I'm curious from your perspective, if GPT and Large Language Models are overhyped, underhyped, what's your view?
Benn Stancil - 00:16:59:
My view is it is like short-term overhyped, long-term underhyped. So I think that the immediate things that we are doing with it are okay. So I want to take two examples, like, you know, everybody is trying to plug various things, you know, like GPT Plugins essentially into their products mode is in the process of the same explorations and all that sort of things. A lot of them take a couple of forms. There are three main forms I would say that I've seen so far. One is like, write a question, get a SQL query that answers the question or some version of that. Another is a code assistant that helps you write queries or whatever, similar to Copilot. And a third one is to summarize a bunch of stuff, like give me the summary like an auto document type of thing. I think the first one is really tough. And I think we're basically like, it works like a toy, but if you do that on top of actual complicated data and stuff like that, there's a lot of stuff you have to figure out to make that work. There are some companies that are attempting to do this in much more robust ways. I think they'll get there. So I do think it will change the interface for how we interact with data, but I think it'll take longer than we think because figuring out how to make these like models actually do that properly and stuff is like a much harder problem than just like, here's a schema, write a query. They do not yet do a terribly good job of that. But I do think it can change things enough to be like a good enough interface that this is now going to be somewhat more of the norm of how we will think about interacting with stuff. The second point I'll make, which is about the third thing about the auto-documenting, I think this is actually where stuff is particularly interesting. On the auto-documenting thing, it makes sense initially to think about, oh great, we create documents, we want to auto-document it, we use ChatGPT to do it, we'll spit out a bunch of docs, great, that's what we're going to do. It makes sense now, but the question it really raises to me is if you can auto-generate docs, why have docs at all? What's the point of creating a bunch of docs that you can create on the fly? Why do we actually even need those things to exist? And I think that's where a lot of what can happen with ChatGPT or with these models or the whole collection of AI tools that are now starting to emerge is the entire way that we start to use products that look different. Like we assume, oh, we need to have all these documents because people will search for them, they'll read them, all those sorts of things. It's like that foundational assumption about how tools work, how the internet almost works, doesn't necessarily make sense in a world where all of these things can be created on the fly. And I think that's the part where I think it's like very underhyped, not necessarily in the sense it's going to solve all of our data problems, but in the way that the world looks in 10 years, isn't going to be like, oh my God, every website is BuzzFeed, every data tool is just like auto-generated on Docs. It's like if everybody can generate BuzzFeed, we stop caring about BuzzFeed and we start actually creating things that look entirely different. And so I think it's underhyped because we are currently exploring the edges of the way that the models of our world currently work. But at some point, we'll break out of those models and they'll have like an entirely new thing that we have to build on top of it, like changing the foundational interfaces into technology in ways I don't think we've really started to comprehend.
Anthony Deighton - 00:19:54:
Interesting. Yeah, I think that's a lot of built in assumptions. And your point about documentation is a really good one, because the reason we write documentation is the interfaces for a lot of these products are so arcane that they need an explanation on the side to guide you through the use of them. In this context, imagining that we simply just interact with them in a very natural way becomes much more reasonable.
Benn Stancil - 00:20:16:
Yeah, and it's weird to think like, oh, we have to actually have the exact same documentation for all of our software because our software is going to look exactly as it's like, no, we don't need to auto create a bunch of documentation for how to use an iPhone. Like the user's guide for an iPhone is like two pages of a picture book. And it's like because they made it so simple to use. And so like, if all the software ends up being the same thing, if we can just ask a question and get an answer, why do we care about documenting the data at all? Like, I'm not going to ever read that. And even if I did, I'll just ask it to generate the documentation when I need it. And so it's like, I don't know, there is something that feels like we're designing that for a world that no longer exists. And I get it. Sure, it's a transition. It's not to say the people who are building are doing something wrong, but it feels like that's a transition into something that is totally new.
Anthony Deighton - 00:20:57:
Yeah, and that also speaks to things like commenting all your SQL code or like schema, like writing long paragraph definitions of what the schema means and things like that, which often are needed for compliance reasons or for government regulations, but don't actually have any utility. And just to your point could just as well be generated on the fly. So in that spirit, and maybe to close us out a little bit, I'm curious from your vantage point, having spent a long time thinking about data and analytics and how people consume and use this, if you cast your eye forward five, ten years, which admittedly is a long distance in the future, what are some predictions you make for the way the world's going to look and feel very different for how people interact and work with data?
Benn Stancil - 00:21:42:
I do think that the chat style model, whether or not it's actually chat, like I don't know that it'll be chatbots. I think that we've again, that's the easy interface into this stuff and ChatGPT released a ChatBot that became very popular. So everybody's like, let's go ChatBot. But I do think there will be much more like ChatBot like things that become the traditional or like the expected way to do this. And part of that is like, there's a lot of reasons why that doesn't exactly work. There's a lot of things where you could say, well, it's not going to be quite accurate enough. How will you answer complicated questions? All those kinds of things. My view of that is, I think it pretty quickly, if we get that good enough, then people say like the speed with which they can ask questions, the depth which they can potentially ask them is like well exceeds what they can do with data teams alongside them and stuff like that. And even if we're not getting as good of an answer, even if it's not as good as doing a bunch of in-depth research, it's good enough and fast enough that we'll start to say like, this is now what we want to do. So for instance, if I want to research a subject, Wikipedia is not entirely right. Wikipedia is not as like in depth in a lot of cases, if I wanted to go like, actually do real research on the thing, but it's so fast, it's good enough that like, you could replace, I could basically just exclusively rely on Wikipedia and I feel like that would be a place that's good enough for me to get most of the answers I need. And I can see the same thing basically happening with how we interact with data, which is yes, we recognize this isn't always exactly right. We recognize that there are problems with using it sometimes. But it is so much faster than the alternative that we come to accept that that's like, that's as good as we're going to get. And we'd rather have that than, you know, the very curated, personalized answers that require a bunch of in-depth work. It's just not worth the cost.
Anthony Deighton - 00:23:20:
That's an interesting point. I'll push back slightly, or maybe tweak your answer, or see what your reaction is. The example I'll give is writing a poem. So one of the early use cases of ChatGPT is you can have it write you a poem. And it is absolutely the case that, to your point about speed, you can write poems very quickly with this GPT interface. But generally speaking, they're terrible. I mean, they're not awful, but they're not great. But they're really fast. And in some ways, I think what that means is that there's a premium placed on the curated poem, the poet who truly writes something that moves you versus the drivel you're getting out of GPTs. And in the same way with analytics, I appreciate I'm maybe stretching the analogy here, but the quick answers that you get through the interface, to your point, are much faster. But that frees the time for the analysts to handcraft something truly moving as it relates to the data. I'm curious how you react.
Benn Stancil - 00:24:19:
So I guess I'd have two thoughts on that. I think that one of the things these things will come to be able to do pretty well is all of the demos are like, give me revenue by country by month, and it spits out a thing. And then you'll see like, oh, revenue is up in the UK, and it's down in India, what's going on? And you may ask, like, explain this to me, and it'll give you like, we broke it apart by product skew, here it is, okay, that's helpful, fine. Explain that to me, and it'll keep doing the same thing. And I think they will get pretty good at that sort of thing, like how far on the string can you pull where you get the basic dashboard and like, oh, this looks like a thing. I have a hypothesis that I think the reason revenue was down was because there was a big holiday in India this month. Can you tell me if that's true or not? Like, oh, here it is. And the one of the things they're actually very good at better than actual analysts, I think, is the SQL writing stuff. They're not great at it, they are very good at being creative. No, to your point, their poetry is bad, but if you say like, come up with 10 reasons why you think the revenue is down in India and it's up in the UK, they actually can come up with pretty good ideas for that. And they'll come up with ones that are often things I think that analysts won't. So I think there will be a way to actually interrogate them in that way to ask these questions such that that type of analysis, I think probably will become much faster. To your point, no, there is a, should we build a factory in Japan? That's a much harder question. That probably does require like, you do want some person who's actually thinking about it. I do think you will keep the poet as it were to help you with those sorts of things, though I don't know if they are good at answering these questions. The poet themselves may want to use the tools to help them get there. And I don't actually know exactly what happens there, but I think there will be more pressure on like analysts than perhaps we like to imagine, though I don't think it will be because they are like magically writing SQL queries. I think it will be more because they are better at reasoning through problems than we want to give them credit for.
Anthony Deighton - 00:26:09:
Yeah, fair point. And I think the idea of treating analysts as poets certainly would make them happy. So if nothing else, that's something to take from that. Oh, look, Benn, it's been a pleasure to have you. And I appreciate the thoughts and insights, sharing what you're doing at Mode, and all the thoughts on generative models and what's going to happen in the future. So thanks so much for the time.
Benn Stancil - 00:26:34:
Yeah, for sure. Thanks for having me.
Outro - 00:26:36:
DataMasters is brought to you by Tamr, the leader in data products. Visit tamr.com to learn how Tamr helps data teams quickly improve the quality and accuracy of their customer and company data. Be sure to click subscribe so you don't miss any future episodes. On behalf of the team here at Tamr, thanks for listening.