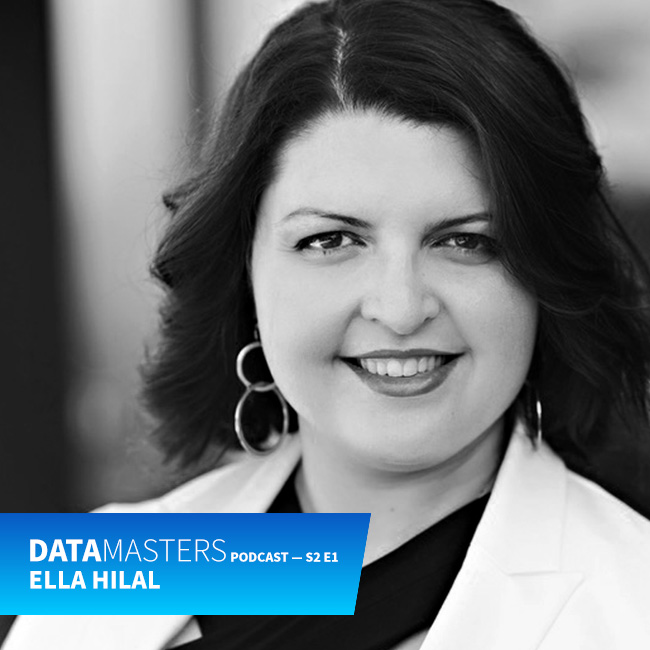
Shopify's Ella Hilal: Attacking data science with an "always learning mindset"
Ella Hilal
Shopify's Ella Hilal tells us why effective data leaders need to instill an 'always learning mindset' and possess a willingness to reject conventional wisdom. Ella also talks about how the data organization continues to evolve at Shopify, the unique challenges of serving entrepreneurs from all over the world, and how data is being used to power growth and expansion at Shopify.
I'd rather read the transcript of this conversation please!
Anthony Deighton: Welcome to another episode of DataMasters. I'm Anthony Deighton, Chief Product Officer at Tamr. My guest today is Ella Hilal, she is Shopify's Director of Data, as well as an adjunct assistant professor at the University of Waterloo, a data scientist, and an evangelist for women in technology. Today, we'll talk to Ella about why questioning conventional wisdom is often at the heart of good data science, why it's critical to have an always learning mindset, and how her team is playing a crucial role in the growth and revenue at Shopify. Ella, welcome to DataMasters.
Ella Hilal: Thank you for having me. I'm super excited to be here.
Anthony Deighton: Excellent. So, before we get into the details of Shopify, I'm sure people would love to know a bit more about your personal background and what stops you've made along the way in your career, in life, that land you in the current position you have at Shopify.
Ella Hilal: I started my career actually as a software developer, I was a Java software developer, and grew into a full stack. I also did my Master's and my PhD in pattern analysis and machine intelligence, and then I took it from there one job after the other, growing into my career, getting to lead from the middle, then started leading a team, moving into a manager role then a director role. So prior to joining Shopify I was the head of data for a company called Intelligent Mechatronic Systems. I'm also up till now an adjunct assistant professor at the University of Waterloo. And yes, I've been with Shopify now for, wow, three years, time flies. But yes, it's been a fun ride so far, it's exciting and every day there's something new to do and to learn.
Anthony Deighton: I think a lot of people in data science often have deep academic backgrounds, and now you work in the industry and I think a lot of people think about that distinction, is there anything you can share based on your, you've spent time in academia, you've spent time in industry thinking about that distinction?
Ella Hilal: I think the key thing that academia provided me is learning how to learn, and I think this in itself is a skill set. You don't have to be an academic to have it, there are people who acquire it over years doing other stuff, but I think academia definitely facilitates enhancement of this skill because this is exactly what you do for so many years, you're learning to learn. You're learning to learn from others knowledge, and then apply it, extrapolate, and traverse this knowledge comfortably.So I think this is a key that academia provides, the ability to learning to learn, and definitely this is something that becomes a superpower in industry. Anybody who is able to grow in an industry role because of how fast technology changes, because how fast things evolve, and how fast we need to ship meaningful products for our customers and our merchants, being able to learn, and pivot, and change, having this constant learner's mindset is essential. It's not limited to being an academic, but this is one of the key things that you pick up during your grad studies. So it helps but it's not solely limited to.
Anthony Deighton: Got it. No, that makes perfect sense. Now, one thing you didn't mention, but that I also did take a note of from your background is your interest in arts. And I understand in addition to the work you do, you're an artist, you've in fact sold some paintings, and it struck me as something fairly unique for someone with your set of accomplishments in the field of data, data scientists and artists are not often a congruent set. It's something that's a bit more abstract and creative versus analytical and data oriented. Maybe you could share a little bit about that and how maybe that side of your personality and brain helps.
Ella Hilal: Well, that's true. So I am an aspiring artist, I won't call myself an artist. I like to paint, it's actually very therapeutic for me and it's a way that I use the different sides of my brain. I do acrylic on canvas and I had a few exhibits, and actually few of my pieces sold. And I remember that every time somebody thought something like this happened, I will be always like, are you sure? Are you sure? Because the data scientist in me looks at being very pedantic about all the different things that went wrong that shouldn't go wrong. And in my head I think what gives me an air cover is the fact that I do abstract painting, so it's very forgiving. You might be painting a dog and it comes out like an aero plane and people are like, "Oh, it's really [inaudible]." Yes.Jokes aside, I actually think data in itself, the way you think about it, the way you actually build a data narrative and a data storytelling, as well as the way you build visualization in itself is a form of an art. It's not about knowing what you need to know, or slicing the data, or building the engineering pipelines, it's also about taking this whole data point and seeing it in your head in a way that builds this whole narrative and story that you can communicate to your stakeholders.So I actually see the two sides of my world mapping and cross pollinating a lot. Also the understanding of what catches the eye and how to make sure if you have an important data point, how do you visualize it? How do you make sure that you're able to communicate it? And that applies whether you're building an analytical product or even if you're building a merchant facing product, either way, you want to make sure that you're providing the most actionable and meaningful insights to your audience. So it does map a bit, not too far apart.
Anthony Deighton: Yes, I know, ultimately much of what anyone does is about communicating, whether you're communicating through art or communicating through data. And as you point out, visualization is really the intersection between the two. But I also imagine, you talked before about this idea of the learning mindset and really being something that you drew from your academic background, something that you find valuable in your work at Shopify around data science. So maybe we could dig into that a little bit more, talking a bit about the always learning mindset, which by the way, I think is really both useful and unique perspective to bring, how did you come to find it to be so important in your work? Are there some specific experiences you had that drove this home for you?
Ella Hilal: Yes, that's a great question. So when I think about it, technology keeps on evolving. Every other day, there is a new library, new tools, new algorithm, new model, new database coming out. So with the right learning mindset, with the ability to self reflect, being humble about the things that we don't know, and seeking the knowledge, helps sets us for success regardless what area we're working on and what's the field.With a very dynamic field like data science, this becomes a huge multiplier, because if you think just about it, the amount of whether it's research, the amount of new tools, the amount of new libraries, the amount of best practices that keeps on coming up, it's huge. This is a very prime field, lush and green. So there's a lot there that is happening, which is amazing for all data scientists, but with this right mindset, we can actually evolve and grow. Also, one key thing I learned very early, and as I mentioned, I started my career as a developer, loving your code, or loving your solutions, or your product, or algorithms too much makes it a barrier for you to build the right products, because the truth is what's right, right now is not what's right forever.So being able to pick up on what can be better and be able to seek that knowledge and evolve, is essential to keep building the best solutions, and there's air quotes around it of course, what defines best. But the best solutions for your customers and your merchants all the time, right? The whole point is making sure that whatever you're providing or whatever you're building is meaningful, useful, and efficient. And the definitions of these things evolve over time because of technology. Having a learner's mindset involves having the self awareness, the humbleness, and the ability to learn and seek knowledge that will evolve over time and will make your solutions, and your products, and your answers evolve over time.
Anthony Deighton: Yes, I think it's a great perspective and I can certainly relate as a person who's thinking a lot about products, we have a tendency to fall in love with our products, and I think what that prevents is that it stops us from questioning things. And your points about humility is really important, humility, the willingness to step back and ask, why do we do things that way? And that's something you've spoken about in the past this idea of five levels of why, maybe you could share a little bit about that perspective.
Ella Hilal: Yes, definitely. So this is something I know that my team will joke about the fact that I'm being a broken record about it, we need to understand the five levels of why. And the reason when you think about five levels is because the first one is, well, because that person wants it, why are you doing this? That person wants it, okay, why? Well, because this is how it's done. And it's like, no, let's go again. Okay, why? And then at the fifth level, this is when you start going back to first principles.So the thing is, we need to tie everything we do back to first principles, and then when we think about it, we need to ... So, just for context, first principles is going back to the very, very basics. Why are you doing this thing? The very basic human need that ties to it. Let's not make it too sophisticated, let's go to the first principles and then build up from there, because when you have the very first principles very clear, every layer that you put of complexity after is taking you somewhere towards the solution of the problem.I always say to the team, well somebody very smart one time told me this and I have been repeating it like a broken record, a solved problem is an asset, the solution is a debt. Every single solution, regardless how beautiful this, how elegant it is, it's a debt, because you need to evolve it, you need to make it better, you need to enhance it. So by thinking back to the first principles, you always can challenge the solution and make sure that you're always having a solved problem. And that does help a lot with technical debt and how you think about it.The other thing that is important too is thinking beyond, what is it now? I always ask, is the six month Ella would be grateful for the decision that I'm making now? And don't get me wrong, sometimes I throw my six month future self a lot of shit problems to solve, or a lot of hard problems to solve, but it's intentional, instead of blindly just passing the ball to my future self, I'm intentional about, okay, I know that this decision will cost me one, two, or three, and I can live with them so I'm just passing them along, or you know what, no, I should do another decision, that would be too hard to fix or to revert.So going deep into the five levels of why, going back to the first principles and applying some second order thinking or even third if we can is, what is actually the second or third level implications of a decision I'm making? All of them are essential just to make sure that whatever strategy we're building, whatever data products will solutioning, all are able to tie it to a meaningful thing that we can actually maintain for the long term and it's not just a bandaid that feels good now but later on we'll need to rip it off and throw it away.
Anthony Deighton: Relating this back to our earlier conversation around your academic background, I've often heard and I think it's true that if you really understand something, you should be able to teach somebody else. So the ability to teach somebody is also ... and I think that comes back to your point about first principles, if you truly understand something in a deep way, you understand not in a jargony level, but you actually understand it in the context of a way you could explain it to a kindergartner or to someone who's not trained in data science, is that something you've found?
Ella Hilal: Yes, that's actually a great point. So my dad actually used to say that all the time, I think it's an Einstein quote, if I remember right. If you know it, now if you can explain it to a four year old or if you can't explain to a four year old, you don't know it good enough, something like that. So 100%, but also on a leadership level, let's think about it from a leadership level. The fact is, good cohesive, well jelled teams are the ones who all are working towards a shared vision. And the shared vision, when it becomes too complicated, or it doesn't tie back to the first principles, or you can't answer the five levels of why, this understanding of the shared vision will not be consistent across.So what would happen is, you will have a team that each of them running in a different way thinking they're working towards the vision.So having this clarity, taking everything back to first principles, talking back into a clear why, is essential to get this teams to be efficient, cohesive, working together to where they need to go. I think this is something that I got to see over and over every time we build, for example, data product and reproduction it. The key is, we need always to start with the why, what is the value, not the algorithm, so easy to tie everything when you talk to even my much younger self, I will go talk about the algorithm and I do this, and I do that.Truth is, if we step back, we look at the business problem. And the business problem, for example, we were let's say Shopify Capital, we are helping merchants get the capital they need to grow their businesses, right. So when you phrase it this way, it's understandable and then all the different pieces plug into this overarching vision. But when you go and talk about, well, we're going to do this forecasting algorithm, and we're going to do ... Don't get me wrong, all of this is very important, but all of them need to feed into the mission, and how we're helping merchants, and how we're empowering them to live their needs. So taking it back to first principle and tying it all together, does help a lot even from a leadership level, not just from a personal building blocks into any algorithmic work.
Anthony Deighton: So building on that and maybe thinking a little bit about the organization, I think a lot of people listening to the podcast have as their responsibility, either building or leading a team within their company, data team within their company. And I know that's absolutely something that you've done at Shopify, I think it'd be fair to say Shopify is really a data driven organization and you've built somewhat unique team structure. So maybe just share, how is the data science team at Shopify organized? And why have you chosen to organize it that way? How do you think about the challenge of team building in data science?
Ella Hilal: So before I dig into answering the question, let me give to the listeners a quick overview of what Shopify is, right. So Shopify is a commerce platform, we have the mission of empowering, making commerce better for everybody. We exist to empower merchants to build their dreams and just focus on what they need to do, right. Just making sure that they are focused on their business, and we take on the burden of building the platform, making it up, making it running. So Shopify exists to make commerce better for everybody. As we say, in their power levels, the independent entrepreneurs to build their own brand and live their dreams, literally achieve financial independence. So this is why Shopify exists, and this is our key mission.Talking about the data teams that I have built in Shopify, so I've been in Shopify for three years, I initially started heading the data teams for plus, which is the large merchant in Shopify as well as international, with a mission of taking Shopify to be a perfect market tech for every country out there. Today we're already in 175 countries but our objective up to now, of course, is making sure that Shopify meets the unique needs of entrepreneurs in different visions, because it's not one size fits all.Building different teams under these different constraints are a tad different, right. So there is a different way when you think about building a internationally distributed team, from building a team that is working with the merchants that have the most data as well as are the most demanding because of the skill of their business. And I think when I was thinking about it, I saw the problem as, yes, there's a lot of overlap but I thought about it from some key fundamental aspects.So number one, in data in Shopify, we are fully embedded, that means we work with every team embedded, sitting with them, living their pain, seeing the wins and living the losses. So, in my opinion, this is so essential, because I've worked prior into organizations where the data team was a separate unit on the side or something like that. The challenge with this is it becomes a handover problem, and you're not living the wins, and then at this point of time, you're five level why's, you can't go deep enough, you don't always go to first principle because you cannot actually impact the merchant directly, there is many layers of separation. But when you're embedded in a full product team, you're living the wins and living the losses. So at this point of time, the concepts that you're trying to move the needle on, can be at first principles because you're living the business problem. So this is essential.So the data science in Shopify is still a craft. So we're very focused on it, it's very consistent, but then we're also embedded within all the different teams. We also work with full stack data scientists. So what does that mean? So our teams are consisted of full stack data scientists, people who work from ETL, so from the acquisition, making sure that the data is in, all the way into the machine learning. We don't split the roles in Shopify data into, that's an ML engineer, or that's an ETL engineer, or that's an analyst or data scientist, what we do is we work across the stack.And I personally feel very strongly about this for a couple of reasons. When you split it, it's easier actually to hire, but the challenge is, again, your workflow becomes a handover workflow. So you need somebody to build you the pipelines, so that somebody does the analysis, so somebody then goes and takes that and build a machine learning model. So again, becomes very hard to have ownership on the problem. And I value ownership a lot because when you have ownership, it's your win. You're working so hard to move the needle on something, but then you're also so incentivized to do this because it's your win, you are able to make a life of entrepreneurs better.So what we have is we have data scientists that work across the stack, from acquisition all the way to the machine learning. And if you think about it, you cannot build a machine learning model without understanding the statistics of the data, and the statistics of the data come from the analytics. And for you to get the full analytics and really their full impact, sometimes you need to do experimentation, or hold, or something like that, so again, that's an optimization work that data scientists need to do.And then you can't do any of that without having the data ready in the database. So again, it's all interdependent. So when you have control over your pipeline, you actually have more autonomy and you can have bigger impact. And I also found that full stack data scientists tend to work much closer to engineers, you [inaudible] and PMs because again, they all are working towards the same mission versus a handover phase. Curiously, on my Python is 99, you're the one who didn't implement the drive into production, we don't have these discussions. We're like, it's not right, okay, let's fix it. And I think this is a mindset that I value a lot and I think does have the right impact on the business and the teams.
Anthony Deighton: So let's dig into, you make this point about these really unified teams and the tight integration with the product, I think that's really interesting and very valuable for listeners, but I imagine that also makes things challenging when it comes to recruiting. So what are the the key traits are looking for, maybe hard skills but also soft skills that you look for when you try to recruit, build your team?
Ella Hilal: Yes, that's a great question. I chat with that a lot with my team as well as my peers. So, of course, hard skills, this is becoming more of a standard thing now in the industry. So of course, we're always looking for strong Python, strong developers because we work across the stack. So just Python as a scripting tool on the side is good, but building production code is very useful, and it's a key skill that we actually interview for, being able to have this strong understanding of metrics, of algorithmic work, of ML models is again, essential. We work with Scala, with Python, we worked on Presto, on BigQuery, and just having understanding of all this and how the ecosystem works is great.But I think the thing that is unique too and we're very keen about is some of the soft skills. So I always say I'll hire on the three H's, which is humble, honest, and hardworking. And I know that's a simplifier, but I think they all tie into each other. And of course, it's not the only thing but humble and honest is being able to be self aware and be able to seek knowledge, seek feedback, want to work with others, build, I'm a big believer in collective intelligence, I think I'm smarter by everybody I talk to I'm not smart on my own, and I don't think anybody is, I think we're all smarter together as a collective and the strength is in the collective more than the individual. Of course, the individual's component it feeds into each other but we're stronger together.So being humble, honest, and hardworking is essential things from soft skill, being able to, again, have this deep thinking of the business case, or the why, making sure that they have high ownership, all of these are key things that are essential that when I am interviewing somebody I keep in mind and make sure to have the discussion on them.
Anthony Deighton: By the way, I think those humble, honest, and hardworking are really excellent criteria, something people should take a note of. But this issue of honesty is a challenging one because being honest, especially as it relates to data science, can be very difficult, it puts your team and potentially you in the position of having to challenge the conventional wisdom inside an organization. And you started our conversation thinking about the way we've always done things, right, and sometimes, or I would suspect often, you find that the data tells you something different, it causes you to challenge the way people have often thought about it. Maybe there's something you could share that would be illuminating for listeners.
Ella Hilal: So I can share a story that just recently happened. So I lead the growth data team, and I know growth might not always be an known thing. So what we are focused on is making sure that merchants who are interested in joining Shopify can join Shopify in the most seamless way, and we're setting them up for success. So it's not just making it easier for them to join, it's also making sure that they are activated, and they are set on a path for success, building their businesses and their dreams.So growth in itself has multiple facets. So has the acquisition part, which is a marketing aspect, but also has a very strong product in engineering side which is, at every point of the interaction, how can we make sure that it's easier, it's smoother? And how do we make sure that they are able to join Shopify and get the information they need right away, know what tools to install, just make their onboarding journey much, much better?So it does have the multiple aspects to it. So it has a very business front facing teams, it has a very strong engineering and product side, and is very dependent on data, which is awesome. So this combo is great. So one thing that we have just done, we have done an analysis to understand, what's the impact of the different channels and different behaviors on our retained merchants? So, of course, everybody's baby is the most beautiful baby, including mine, I love my babies, right. But the truth is, not everything behaves similarly, right. There is varying impact of the different channels for example.So if we're considering Facebook, versus AdWords, versus Instagram, all of these are different tools and people interact with them differently. And accordingly, when merchants come in they go through different flows, but then the flows merge and they tend to behave differently on the platform. So we have done a deep dive, actually a large deep dive to understand what's these behaviors. And based on that we had really good discussions with our peers on, what's the impact of these different channels? And what's the impact of the spend on these? And what's the ROI? And what's the action that lead to retention, right? Lead to this mentioned thing in our platform and being successful.So the key here was coming in with important questions is like, hey, we observed that in data and this is what we believe is happening, what do you think is happening? And this is where humbleness comes in because the truth is, it's so easy, there is confirmation biases, right. You can trick yourself into confirmation bias, I think this channel is not good, and I see something, it must be not good. But there is data issues, there is incomplete data or incomplete context too, right, the data lives within a context.So coming in with the right questions, and again, asking the five levels of Why, which is, why do you think this is happening? Okay, so that, but why do you think that thing that you just mentioned is happening? So as you dig deeper, actually, everybody becomes way more aligned, even if you're challenging the status quo, because at the end of the day, again, we're all working towards the same mission.One key thing that I actually highly, highly value at Shopify is all companies say we're mission driven, but the truth is every single human in Shopify lives and breathes the Shopify mission, which is making commerce better for everybody, literally bending the curve for entrepreneurs. So we all live it and now as soon as you start talking with, well, we don't think this is the best way to bend the curve, you find people are like, you know what, maybe you can pivot in that way, maybe you can do it this way.So because we, again, take it back to first principles, which is where we're heading and where we want to go, and go in with humbleness and openness to hear, because we're not going in, we always say strong opinions weakly held. So we go in and this is what we observe, the why of it, people become more open to have the conversation, and at this point of time, we converge to a better direction together. So yes, that's the most recent example that came top of mind.
Anthony Deighton: So that's a great example. So let's talk a little bit about Shopify which is growing incredibly quickly. So I think it'd be fair to call it a hyper growth company. And that presents, in itself, the simple growth problem is itself a problem. So how is the data team growing and how is it responding to the changing environment at Shopify as the company gets bigger, as you as you hopefully achieve that aspirational mission?
Ella Hilal: Yes. So that's a great question. So just to give a little bit of context, so the GMV that was traded on Shopify was over 230 billion in total sales by our merchants last year, that's huge. And we have over 300 million customers around the globe have purchased from Shopify merchants from 2019 alone. 300 million, that's again, massive. So definitely, Shopify has been growing very fast and data is a key superpower that Shopify tries to use across all its product and all our solutions, we have been heavily recruiting, we have been, again, relying on brilliant people who own the problem end to end using full stack data science, we hire people with high ownership so they can help and roll the sleeves.And just to give context, some of the products that we ship that is data informed and ML driven, is something like Shopify Capital. So Shopify Capital, if you don't know, is extending loans to merchants to build them. And at this moment, we hit a milestone of a quarter of a billion dollars, that's as of September 30th 2020. We have another thing that's called Shop Pay, which is an App where people can see their purchases, track it, make pre-orders, and we have over 14 billion in lifetime GMV, which is gross merchant value, which is all the sales that happened on it, and over 16 million shoppers opted in at the end of last quarter, Q3.So again, our scale is growing and our merchants are coming and growing. So to give you another example that I think is cool is Gymshark. So, I don't know, do you know Gymshark? It's activewear. Okay, so Gymshark is an activewear company that started in the UK and it's a global brand now, and it's amazing. Started from a small merchant just making some activewear, all the way to this big, ginormous, global merchant worldwide, and all of it happened on Shopify. So it's just heartwarming to see small merchants starting their business out of their garages or out of their dining room tables, and then growing to this massive, large scale.So yes, definitely the skill has been huge, and the growth and rate by which the growth is, is huge, but I think it's also a super fun ride for all honesty, because it's a good mission, it's one of the very few missions that also is aligned with like it has a good economical impact on countries, it has a good impact in merchants themselves, right, they're reaching financial independence. As well as it's a good option for buyers because it gives them by diversity of options to opt in. So all of that in addition to how the skill and the impact, as well as how data-driven, and how honest, and humble, and great people are at the company it's been crazy but has been a fun ride.
Anthony Deighton: And your example of a UK company leads us to what I think is a another interesting topic which is, Shopify is going international and really thinking about a global push, has that had any impact, from a data perspective, on how you thought about building and answering data questions?
Ella Hilal: 100%. So Shopify is already in over 175 countries, the global push was to make sure that we are a great market fit. And I think this is where the data becomes interesting. So for example, North America is very credit card heavy, and it's so easy to assume all first world countries are credit card heavy, well, it's easy. No, that's actually not true. If you look at it, you would have, for example, Germany, they appreciate direct pay, we have like similar too in Canada, we have e-Transfers or something like that. In most of the world, actually, cash is king still.So assumptions get challenged. So again, as I mentioned, data lives in a context, and it's important to understand the context around the data to be able to understand the trends, the patterns, and what you're building for. And the more you dig into the different unique needs and requirements of each country, you find, actually, there's a lot of differences. So for example, interactions with an online platform might be totally different based on colors, because in certain cultures colors have very, very particular meaning and carry a lot of weight. So accordingly, choices of colors are important and interactions with websites and with stores might differ based on that. Again, context is key for everything.Then you have global platforms that we're not heavily using. So for example, WeChat is a key, key platform in the APAC region that is very heavily used, but its presence in North America, of course it's present but it's not as heavy, or as large, or not as ubiquitous as in APAC, and there's WePay, there is Baidu as a primary search engine. So the as you dig into the different regions, there is also unique needs, whether it's selling needs, whether its interface look and feel needs to the platform, whether it's also onboarding needs. For example, Japan is a very high touch country, so you can have everything automated, but they do value having the conversation. So having certain type of support available is different.So again, as we invest more in the different global markets, from a data side, we have learn a lot as we put more context, and we have amazing in-country teams that help us collect that in context, as well as we have now data teams embedded globally. Just having that additional context around the data makes us understand what's happening so much more and build much better products for our merchants globally because we understand how they're interacting with it.
Anthony Deighton: Fantastic. So, well, I think we're coming up on time and I just want to thank you for amazing insights. I mean, Shopify sounds like both an amazing company, great mission, high growth, and it sounds like the data science team is really at the center of that. So when we think about this broad theme of creating the data driven enterprise, at its core, I think every business ultimately is a data business. I think it's fair to say Shopify typifies that as really an exemplar and you're really at the center of that, so congratulations on remarkable work.
Ella Hilal: Well, thank you so much for having me. I'm super excited to be here and it was great chatting with you, it was a lot of fun, thank you.