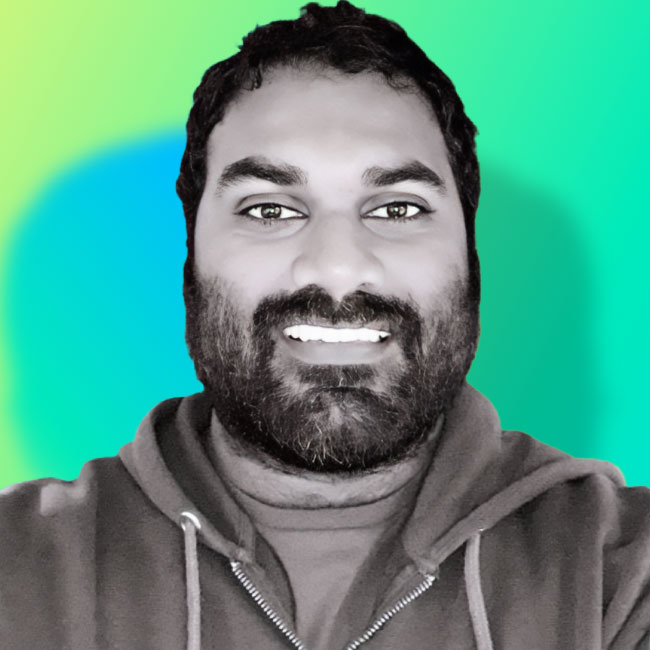
Exploring the Modern Data Stack and AI:A Conversation with David Jayatillake, Co-Founder and CEO of Delphi Labs
David Jayatillake
The modern data stack is dead, according to David Jayatillake, co-founder and CEO of Delphi Labs. Or is it? In this episode, we dive deep into the modern data stack and the transformative power of artificial intelligence and large language models like GPT-4.
David has a wealth of experience in data and analytics, having worked at companies that embraced modern data practices and tools. He shares his insights into the evolution of the modern data stack and the problems it was designed to solve. We also discuss the intersection of AI and data analytics, highlighting the potential of large language models like GPT-4 in revolutionizing how we interact with data. David envisions a future where accessing and analyzing data becomes as natural as searching the internet, thanks to AI-driven natural language interfaces.
Join us for a thought-provoking conversation about the future of data, analytics, and the role of AI in shaping the way we work with data. Whether you're a data professional or simply interested in the data-driven future, this episode offers valuable insights into what lies ahead. Don't miss it!
I'd rather read the transcript of this conversation please!
Intro - 00:00:02:
Data Masters is the go-to place for data enthusiasts. We speak with data leaders from around the world about data, analytics and the emerging technologies and techniques data-savvy organizations are tapping into to gain a competitive advantage. Our experts also share their opinions and perspectives about the hyped and overhyped industry trends we may all be geeking out over. Join the Data Masters podcast with your host Anthony Deighton, Data Products General Manager at Tamr.
Anthony Deighton - 00:00:39:
Welcome to another episode of Data Masters. Today I'm joined by David Jayatillake, co-founder and CEO of Delphi Labs. Delphi Labs is revolutionizing how users access and analyze data with its self-service analytics platform powered by OpenAI's GPT-4. David himself brings a wealth of experience and really is a visionary in data and analysis. He's held leadership roles, including the head of data at Metaplane, chief product and strategy officer at Avora, and VP of Data and Analytics at Ruby Labs. David's also an investor in Metaplane, Lightdash, and Gravity. We're happy to have you here with us today, David. Welcome to the show.
David Jayatillake - 00:01:23:
Thanks so much for having me. It's great to be here.
Anthony Deighton - 00:01:25:
So maybe we could start. I mean, you've had a fairly storied history in the space of Data and Analytics and how users access and use information, make decisions. Feels like you've both used every tool under the planet. Maybe you could share a little bit about your journey, how you come to be here, your career path.
David Jayatillake - 00:01:45:
We could just sort of start there. So I think as a kid I was always like quantitatively minded. I'd always be asking how much or questions like that. And I did those kinds of like STEM subjects at school, did mathematics with like a bit of Econ and Finance. At University. Um, ended up in big four accounting through like graduate schemes and wasn't really for me, but the part that I really liked was the analysis part and having never really touched a database or SQL or anything like that, I thought I decided let's go and be an analyst somewhere. And so I started applying for analyst jobs when I realized I didn't want to be in big four accounting anymore. I ended up with a company called Ocado, which is the UK's and possibly the world's first online grocer. And Ocado is like a bit of a data mecca actually, because even back in 2010, when I was there, They had probably still the best data I've ever seen. And you can see why they have advanced robotics inside their warehouse. Everything runs on data from a production point of view. And really, the data that we were using for analytics was very much a replication of the data they used for production. And so that's why it worked very, very well. And that's kind of like where I learned my SQL, where I learned my advanced Excel skills for like dashboarding and doing analysis on the data after the fact. Learn how to do some interesting stuff in DBA and things like that for scripting, essentially using it like Airflow and few other tools as well. That's really where I start my journey in data. And then I spent a long time in payments and doing different data roles. They had different names, but essentially doing some analytics engineering, data engineering. And then running a commercial analytics team that looked at pricing towards the end of that time, then moved back into mainstream data roles. So I was head of data at a company called Elevate Credit. And then before becoming like taking a few different data roles at a tech company called LIS. Based in London, which is like a fashion search engine with some really interesting data problems around. Recommender systems and a very large product catalog. Does that interface with quite a complex web and app experience for users as well. And that's pretty much where I jump off into my journey. As a founder, I spent a brief amount of time at Ruby Labs after leaving LEST and then joined Devora to help them try and spin out a new company out of an existing startup. That didn't work out, but then ended up at Metaplane, had a really good time working there, again another Modern Data Stack company, and before founding Delphi with Michael Irvine, my friend. Co-founder and that's kind of like where we are today.
Anthony Deighton - 00:04:53:
So in a way, you really sort of grew up with the Modern Data Stack. Like you started by thinking deeply about data problems, working at companies which were by their nature very modern in their consumption and use of data. And then my assumption is able to see the Modern Data Stack develop. I think as a result, and having read some of your writing on subspace, you've clearly thought really deeply about the Modern Data Stack. So maybe to ground this conversation, because I think in a way it provides a foundation for what you're doing at Delphi, but also sort of maybe the way you think about Data and Analytics. Maybe you could share a little bit about what is the Modern Data Stack in your view? And then more importantly, your view on the problem that it was invented to solve. Since it's not as though we invented the Modern Data Stack to do Data and Analytics, we've been doing that for 25, 30 years, if not longer, going back to mainframes. So something changed with the Modern Data Stack. It's designed to solve a problem and do some really wonderful things, maybe share the positive side of the Modern Data Stack.
David Jayatillake - 00:06:09:
Yeah, and I think what I would say is I didn't start out on a one-day set. If you think about my first day to a card day. The Database I was using for analysis was an Oracle database. It was very well structured and organized and looked after by the DBAs there because they were the same ones who looked after the production database and they treated it the same. And that worked well at the time. It started to creak a bit as we grew in scale. At that point they were talking about moving us to something like Greenplum or even the technology like that is pre big data era technology. And you know. I was using Excel primarily as my analysis tool. And this was even like Excel 2003 before we had the million row limits, like 30,000 row limit. So that's like a very much a not a Modern Data Stack. That's like old data stack, probably one of the first data stacks that people have used, right? Which is Excel plus an SQL Database. After leaving Ocado, I spent a lot of time using tools like Microsoft SQL Server, again, with Excel. Learning how to use stored procedures to do like more longer tasks that couldn't be done in a few operations or one script. And that's where I really began to feel the pain because often with Microsoft SQL Server, part of its appeal to organizations was that it could be run on a relatively small piece of infrastructure. So I'd be trying to serve and run analysis and data transformation on quite large dates. So if you think about a company of well-paced scale and the number of even just transactions that they've processed in a given year, like trying to do that on Microsoft SQL Server where it's running on one server rack, we'd hit problems all the time. We'd run out of space. We'd run out of memory. And we'd be struggling with that the whole time. It would be a constraint on everything that we do. We wouldn't do some of the more complicated things that we could imagine because of the constraints of the infrastructure and the constraints of the technology which was designed to run on that infrastructure. And so I stayed pretty much in that Microsoft SQL Server world until I first encountered Snowflake, really. And that's where, because I had some ex-colleagues, so Chris Tabb of LEIT DATA. He had worked with me at Worldpay and I could remember him talking about Snowflake a lot when it came out and he was talking about it being the future. And I remember moving directly off SQL Server to Snowflake and just seeing this amazing lift in what I could do. And we used to have something that would take two or three hours to run, like a query that it was like this was it like inside a credit risk context at Elevate. And I remember working with my counterpart in the credit risk team and him running the same thing that he took two or three hours to run on the previous stack and running it on Snowflake. And even on the small cluster on Snowflake, it would take minutes. And then if we use the large cluster, it would take like 20 seconds. And he was just absolutely blown away that we could just iterate that quickly, which we could never do before. And therefore, we'd always constrain ourselves to do to work within those limits. And that's where I kind of leap off and I stay. I saw those, I experienced firsthand the benefits of what you'd now call modern dataset with a tool like Snowflake. And I've worked. I've tried very hard as I've gone through my career after that point to bring tools like that into my stack. So when I then moved from there to list. They had Redshift, which is still, I guess, called Modern Data Stack, but it was the very start of the Modern Data Stack and had a lot of problems in terms of. Some features not being there like to deal with concurrency and multiple workloads. And then bringing in Snowflake, but they had things like Looker there. And then I brought in tools like dbt. And that's when we probably had what you'd call the first Modern Data Stack that was typical of the time.
Anthony Deighton - 00:10:32:
So at the same... It feels like your experience with the Modern Data Stack is largely around letting the infrastructure get out of the way and get you to a place where the speed of asking questions and answering them isn't mediated by the infrastructure. That's wonderful and freeing, but you've also written that the Modern Data Stack is dead. And this is a sentiment that I can certainly empathize with as much as we talk about the Modern Data Stack as being a wonderful set of technology that gives you a lot of tooling to do a lot of things. I think equally we're seeing customers struggling with having to knit together three, four, five, ten, fifteen different pieces of technology, each of which does a small piece, many of which overlap with each other, that do some part well and another part poorly. And they essentially end up becoming an IT software development integrator to try to get the stuff to work together. And all of a sudden we move away from single vendor's decisions mediating what questions we can answer to a place where having to be our own systems integrator mediates what we can answer. Would you agree with that sentiment? Is the Modern Data Stack dead or is it just having a moment?
David Jayatillake - 00:11:57:
If you think about the title of that post, it was the title I gave was the Modern Data Stack is dead, long live the Modern Data Stack. And my point was that yes, we need to, if you think about first one data stack I had, which was snowflake dbt looker, it was, I did have to do some platform work to get that functional. I went to terraform things, we had to deal with like how to stream data into snowflake. So we did a lot of platform and infrastructure, but we also were able to do a lot of analytics work as well. And over time, what we've seen is that original kind of smallish 5 tool stack has become 10 to 15 tool stack and it's becoming unwieldy. You've seen like companies have data platform teams and all they do is look after how things inside this data stack integrate. Now, people say that this is terrible, but actually every other engineering team that looks after a production use case also has a platform team. That's long been the case that they've had those teams and needed those teams. So is it the case that it's why is it that data being becoming much bigger than it has been in the past? Is that unreasonable to expect? The thing about it that I think makes it potentially unreasonable is if there is just unnecessary complexity in the stack. And that's where you refer to map text diagram. Some of those tools in that stack are covering a tiny surface area, and they're just too small. And they should be part of some bigger tool. And like you mentioned, I've worked at Metaplane. I've seen Metaplane kind of grow its coverage to like, oh, they're going to look after Column-level lineage and lineage throughout the stack, as well as observability, and potentially doing some maybe even going broaden that with metadata. And you can see metadata tools becoming broadly covering all everything that you could do with metadata. You see companies like Alvin.ai becoming catalogs, as well as lineage tools. And you can see those sorts of tools will all become very, very similar in the future. And that's a good thing, because then you don't have to have three. You can just have one. And that's good for the customer, providing you can still choose best and breed. Yeah, I think does that cover your question?
Anthony Deighton - 00:14:13:
Yeah, again, I think it's that trade-off between a range of different tools that you're responsible for knitting together. And maybe the other extreme is that you're buying everything from Oracle or some vendor.
David Jayatillake - 00:14:24:
And we're seeing like integrators like 5X and Y42 and Mozart offer a stat. They've done the stitching together for you of those 15 tools. They choose the best in breed. They choose the things that they know work together. Here you go. Pay for one thing and you get everything together. Single like sign-on and access control and it's done well. So you can see it's possible, but they're doing it for you. I think that's actually like a good alternative as well.
Anthony Deighton - 00:14:54:
So one of the important ideas behind Delphi Labs is this idea of using OpenAI's GPT-4 and large language models. In a moment, I want to get to what you're doing with Delphi. But before we get into that, I'd love to hear your take on AI and large language models. And as context for the question, and I have been asking this a lot on this podcast, people's sort of hot take on AI and large language models, I've sort of heard a range of different perspectives and I'll give you the range and then look at your view on it. So the one extreme is large language models are just a statistical oddity. They're simply there to complete the next most logical or most statistically relevant word in a stream. The best version of this, by the way, that I heard, the funniest version was someone called it mansplaining as a service. That was absolutely brilliant. So putting together things which seem like they might make sense, but in fact are just givers. Maybe one step to the right of that would be large language models are really about language, about semantic understanding and have built real intelligence or understanding of human language and that's their sort of function. And then maybe the extreme other perspective would be that large language models and things like GPT-4 really are synthesizing knowledge, are really doing the hard work that humans we used to think are uniquely capable of, which is to say, gathering a set of information, synthesizing it into knowledge. So from statistical oddity to knowledge, what's your view of where GPT-4 ends?
David Jayatillake - 00:16:45:
So it's funny, I actually don't think the two ends of the spectrum are mutually exclusive. So I do think, you know, fundamentally large language models are like a form of like matrix multiplication, right, which is a bit of the joke that is just squad. It is that. But it's so big and so deep and unfathomable that it's also doing the semantics and knowledge part because of like how complex they can be. And that's like where I believe both of those viewpoints are actually true. If you think about how that relates to the work that. Do it's really interesting because we've seen like previous hot technologies like Web3 and crypto being the previous one where people have described it as a technology in search of a use case And for me, large language models, like they are the, it was there was a use case in search of a technology, right? And the large language model is the technology. So it was always how to use case for this technology, right? We've always needed to interact with machines and software and struggled. And if you think about from the very first computers, you know, you had command line interfaces, we had graphical user interfaces, we've always needed an interface with the computer or with the software. And it's always been a struggle. So we've always needed this and this is better than what we've had before. I think about it.
Anthony Deighton - 00:18:09:
So your analogy would be something akin to leap from the command line to the mouse and graphical display. And this is the next logical leap from that.
David Jayatillake - 00:18:22:
100%. This is just the next. Sometimes I think about how technology is like. Forward over time like the wheel, the printing press, the combustion engine. And if you think in more recent times, there was the computer with the graphical, with the command line user interface. And then we had the computer with the graphical interface. Then we had databases, we had cloud. And this is like the next thing after cloud is this, that technology.
Anthony Deighton - 00:18:46:
So I hadn't heard this idea before and I like it. And if we believe that one of Apple's superpowers is being ahead in terms of human interfaces to computers. And Apple was one of the first adopters of the graphical user interface. And again, to be clear, didn't invent it, but certainly commercialized it and similar for Multi-touch on mobile devices. I think you could make an interesting argument that they missed the thread on this one and have jumped to VRAR as the interface and actually missed this may be Apple's undoing. I hadn't thought about that until you said that.
David Jayatillake - 00:19:21:
Well, actually, if you think that they tried this with Siri and they never gave up on Siri, Siri is still there, right? That's their version and they can actually do, they could actually improve it massively with large language model technology. And I think yesterday they announced Apple GPT, I think maybe that's what the colloquial name for it is, but they announced they were doing it and their stock like went up by 72 billion. Apple talk, I don't know, something. And so they're going to do it. I think they probably have been researching it for some time, just without saying so. And so I think they will move on to this. I actually am excited about their AR-VR interface because I feel like... That's almost like a physical interface, like how do we touch our computer? Like, it's like, and if you think about even how we use it, a large language model, just say we type something into a keyboard. Maybe speak into a microphone at best. And could AR just replace the hardware part of how we interface with the computer rather than the software part?
Anthony Deighton - 00:20:23:
Yeah, I mean, certainly an interesting theory. And often we see art providing a sort of vision for the future and blanking on the name of the movie where the person was sort of swimming through the data The idea that as people we want to be able to interact with data in these very physical ways, manipulate it, does seem like a vision which may actually be manifest through Apple Vision as opposed to.
David Jayatillake - 00:20:51:
I feel like the way if I'm traveling, for example, on a plane or a train and like the best I can have is my laptop monitor and even at my desk I've got a monitor and my laptop monitor. So is that the best you can have really? I think we're probably due some kind of upgrade there.
Anthony Deighton - 00:21:09:
Minority Report, that's the movie I lost the name of. So let's shift a little bit to Delphi Labs and what you're working on, because I put words in your mouth, but it feels like it's the intersection of our two prior conversations. This question of human interfaces for data, how we make things accessible, built on a Modern Data Stack, but thinking about how we can take advantage of natural language interfaces, GPT, large language models. So maybe share what you're working on and how you see these worlds colliding.
David Jayatillake - 00:21:41:
So what we're working on with Delphi is, it's a natural language interface for analytics. Using large language models and semantic layers. So what we want to happen is we want to allow anyone in an organization to be able to ask easy questions that they don't have to know a special syntax, they don't necessarily have to know what the metrics and the dimensions are called, they can just ask a question that they want to know and then Delphi will answer with the appropriate and safe response for them. And the way that works today is that we use semantic layers. So semantic layers are mapping between real world things like customers, orders, revenue and like data structures, whether that's tables and columns in a database or files in the data lake or even an event stream. It just abstracts away the complexity of how data is stored to allow an interface to it, which just allows you to know about business semantics. That's the joy of the semantic layer. And you see the likes of dbt, Cube, AtScale , Looker, like Dash Metabase, who all have semantic layers or metric layers that we can use in this way. So Delphi does not generate SQL. There's a lot of tools that have come out that have offered what looks like a very similar interface to us. And a similar experience, but the issue is they write SQLs. They get exposed to a database schema, and then they generate a SQL query, run it, and give you the answer as a result that you are asking for. And the problem that I see with this is, sure, large language models are great at writing SQL. They've read a lot of SQL from Stack Overflow and whatever else they've been fed. That's fine. The problem is that they don't have very good context into your organization. And the best context you're giving them is a database schema and maybe some additional information maybe from a data catalog. And it's not great. And what we've seen is that GAS is hallucinates a lot. As soon as it's on a very complex or unclean dataset , it really struggles to give you a good answer. And what Michael, my co-founder, and I have known from working in data teams for as long as we have, if your tool is wrong a lot and guesses a lot, people will just stop using it and not trust it. With data trust is everything, that's what we know. It's so easy to lose as a data person, so it's true for a tool as well. And so that's why our goal has always been with Delphi is to provide people with safe answers. And if we can't give an answer with any confidence, we'd rather not give one at all and tell you to go and speak to a data team. So some of these other competitors who are doing Text2SQL, they're saying things like, oh, you don't need a data team, you can just use us. We fundamentally disagree that we need that data team. We need those engineers setting up that semantic layer and doing the good work of. Getting data there cleanly and completely with accurately, which we can then leverage to offer like in a very high bandwidth and quick interface to save answers for people. So we do help those data teams become more efficient. Like, so there are a lot of Junior analysts out there and I've been one of these who are just essentially a semantic layer themselves. They just get asked a question and then they translate that to SQL because they know how the data fits together. And then they run the SQL and give the answer back to the person, except this can take hours at best, weeks at worst, and sometimes not at all, depending on the priorities of the team. These analysts, they need to have lunch, they work eight hours a day, they sleep. And Elvie doesn't do any of those things. You can ask and answer anytime you want and get a response back in seconds or minutes at worst. So that's kind of like what we're trying to solve. It's like the two-sided problem of you have these data teams that are having to be bloated and having to be disrupted because they keep getting hit with these small questions. But then you also have the other side, which is the stakeholders who need these answers quickly and they just often left waiting and frustrated and both sides need a solution and that's what we're trying to solve.
Anthony Deighton - 00:25:51:
Yeah, so the disruption here is really the junior analyst. Maybe that's the person who being the hope is the junior analyst becomes the senior data scientist.
David Jayatillake - 00:26:01:
Yeah, exactly. And I think it does allow for that because to be honest, you don't need to spend very long. There are simple questions before you want to upgrade yourself and start doing deeper pieces of work. And really, I think in the future, when you think about how you want to use humans in the workplace, you don't want them to do repetitive or rote work. You want them to be being human and being creative and doing things that a machine struggles to do.
Anthony Deighton - 00:26:25:
So you made this, I think, an important point there I just want to highlight for a second, this idea that at the core of even what you're doing, semantic layers, is clean, curated, high quality, recently updated data. And your point, I think, is an excellent one that the data teams responsible for that aren't going away. And the idea that we're going to disrupt data teams is probably false. One of the interesting new concepts that I hear coming up and that you haven't spoken about, so I thought I would throw it out there and ask you about our data products. The idea of a data product and then also treating data as a product, this idea of data product managers, something Tamrs spend some time both thinking about and writing about. But I'm curious on your hot take on data products, if they make any sense, you see any role for them.
David Jayatillake - 00:27:18:
And I'll just touch on the point about clean data quickly, that like Meta just open-sourced Llama 2, I think over the last couple of days, and their model code for generating it is only about 1,500 lines of Python. It's really simple. The what's actually important for that model is the quality of that dataset that's fed it. That's the complexity. So even generating large language models is about doing data engineering well, moving on to data products. Yeah, I absolutely value the concept of thinking about data as a product. And I've spent a long time thinking about that perspective. I'm not so zealous with it that I would say to everyone, you must always think about data as a product because there are times where it doesn't fit as well as it does with software engineering. And often that can be because what you're trying to actually get to with data isn't as clear. Because when you set out to build a piece of software, you have clear requirements, what you're setting out to build and what it's supposed to do. With data, and I've been asked this before, it's like sometimes you're just trying to find out what's out there, and you're trying to find out how something works. So you don't know what you're going to find until you start looking. And that's partly where the data as a product mindset starts to break apart. But I think generally, a lot of the time it does work well because many times in data you do have a good understanding of how deterministic is it that what we're trying to build is possible and what we need to do to get there. A lot of the time it is true, you can know those things, but there are times when it's not true.
Anthony Deighton - 00:28:59:
So last question, and arguably an unfair one, but cast your eye forward five, 10 years into the future in the Data and Analytics space. What are some unexpected predictions that you might make about how organizations and people work with data that you see as coming to fruition that maybe others do not?
David Jayatillake - 00:29:23:
Yeah, that's very interesting. I think that infrastructure will be hidden even more. From not only the user who currently has to concern themselves a bit with infrastructure, I think in the future they'll just be here's a interface whether that's a text box or whether that's some AR feature or whatever it might be. This is all they need to care about. They go there, ask for what they want, and whether that's gonna be delivered by AI or whether that's gonna be delivered in conjunction with some very highly skilled human, that's all they need to care about. And they don't need to worry about 10 different applications. They'll have a clean way of accessing what they need and getting to value quicker. That's definitely one thing I believe the future holds. I think it's just the stakeholders are demanding it and the stakeholders who understand what they should expect from data are demanding it even more loudly. It's interesting, I spoke to Bob Mugler recently, and I also heard him on the analytics engineering podcast with Tristan. And he said on that, he asked me two years ago when I thought AI was coming out, I'd have said 2100 and not cared very much about it because I wouldn't, I'm not going to be here. But he said, now, if you ask me, I'd say 2030, and I'm excited because I think I might be here. And I was like, that really changes things. You know, 2030 is not very far away. And if someone like Bob is saying he thinks it's 2030, I'd say it's probably definitely going to be here by 2040. And that's really going to change everything. If you think about all of that data platform work that we talked about earlier around the complexity of it, a lot of that is interfaces. It's interfaces between different systems that make it hard to stick those things together and compose them into something good. If those go away because a system is capable of doing it without you having to think about it, or the system is capable of just building one of those components on the fly without you having to think about it, then it starts to feel a bit like a Gene Roddenberry view of how you interact with computers. It's like that and it's much less painful for everyone. That's my hope. I think we're going to see a leap in the way people interface with computers that we haven't seen for a really long time, not since the 90s.
Anthony Deighton - 00:31:48:
Yeah, I think what's interesting about that is you're putting together this idea of interfacing with computers and interfacing with data. And so the idea that business decision makers are simply interacting with the data that drives their organization. Interface is very similar to the way they interface with the computer, you know, to the other ways that they work with a computer. I think that's a really interesting convergence, like again, to map back to something we were talking about earlier, this idea that there's a strong divergence between how we interact with data, i.e. SQL and Excel and these sorts of things, and how we interact with computers where we click and drag and visual. That I think is a great vision for the future.
David Jayatillake - 00:32:30:
And I think we've seen this sort of thing actually happen before. So when we started. Becoming a Google-dependent civilization, which we are. Before that, you'd have to look in the yellow pages, or you'd have to go to a library or look in career or something to find information. It was so slow and you'd do things much less. And now today, anytime you think about anything that you want the slightest answer to, you Google it. And I think we'll see that shift in the business context as well. And that's, I guess, what I mean, like that convergence of data with the LLMs as well.
Anthony Deighton - 00:33:10:
Well, fantastic. David, thank you so much for the thoughts and for joining us on Data Masters.
David Jayatillake - 00:33:16:
Thanks for having me. It's been great fun.
Outro - 00:33:19:
Data Masters is brought to you by Tamr, the leader in data products. Visit tamr.com to learn how Tamr helps data teams quickly improve the quality and accuracy of their customer and company data. Be sure to click subscribe so you don't miss any future episodes. On behalf of the team here at Tamr, thanks for listening.