Tamr Co-Founder and CTO Michael Stonebraker Wins ACM 2014 A.M. Turing Award
“Nobel Prize of Computing” Recognizes His Pioneering and Extensive Contributions to Modern Database Systems
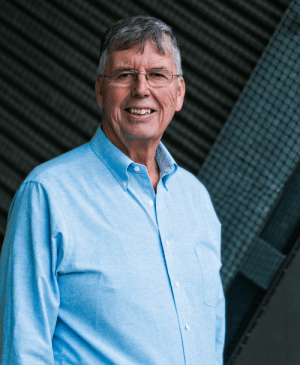
CAMBRIDGE, Mass., March 25, 2015 - Michael Stonebraker, Ph.D., co-founder and CTO of Tamr, Inc., has been awarded the 2014 A.M. Turing Award by the Association for Computing Machinery (ACM), the educational and scientific society, it was announced today by the ACM. The award, which carries a $1 million prize with financial support provided by Google, Inc., recognizes Dr. Stonebraker’s fundamental contributions to the concepts and practices underlying modern database systems as well as their practical application through nine start-up companies that he has founded.
The Turing Award is often referred to as the “Nobel Prize of computing.” It is named for Alan M. Turing, the British mathematician who articulated the mathematical foundation and limits of computing.
Dr. Stonebraker, 71, is an adjunct professor at MIT CSAIL and co-director of the Intel Science and Technology Center (ISTC) for Big Data. The ISTC is an Intel-funded initiative that brings together some of top academic researchers in the US involved in database systems, to create new technologies for dealing with big data.
He has been a pioneer of database research and technology for more than 40 years, and is the author of scores of papers in this area. He was the main architect of the INGRES relational DBMS; the object-relational DBMS POSTGRES; and the federated data system Mariposa. He created start-up companies to commercialize these database technologies and, more recently, big data technologies (Vertica, VoltDB, Paradigm4, Tamr). He is also a member of the National Academy of Engineering and the American Academy of Arts and Sciences.
“Mike has made incredible contributions that span both industry and academia,” said Andy Palmer, co-founder and CEO of Tamr. “Much like Alan Turing used codebreaking as the compelling reason to advance the development of the first computer, Mike has used commercial applications to compel the advancement of new ideas in database systems at a miraculous pace. It’s been a privilege to have him as a friend and my co-founder on Tamr, Vertica, Paradigm4 and many other commercial and academic projects.”
Many of Dr. Stonebraker’s students have gone on to be some of the top innovators in both industry and academic.
About Tamr, Inc.
Tamr, Inc., catalogs, connects and curates the vast reserves of underutilized internal and external data using a combination of machine learning with human guidance so enterprises can use all their data for analytics. Tamr was founded in 2013 by big-data serial entrepreneurs Andy Palmer and Michael Stonebraker, who previously co-founded Vertica Systems (acquired by HP); Ihab Ilyas of the University of Waterloo; George Beskales; Daniel Bruckner; and Alex Pagan.