Power to the People: The Role of Humans in AI
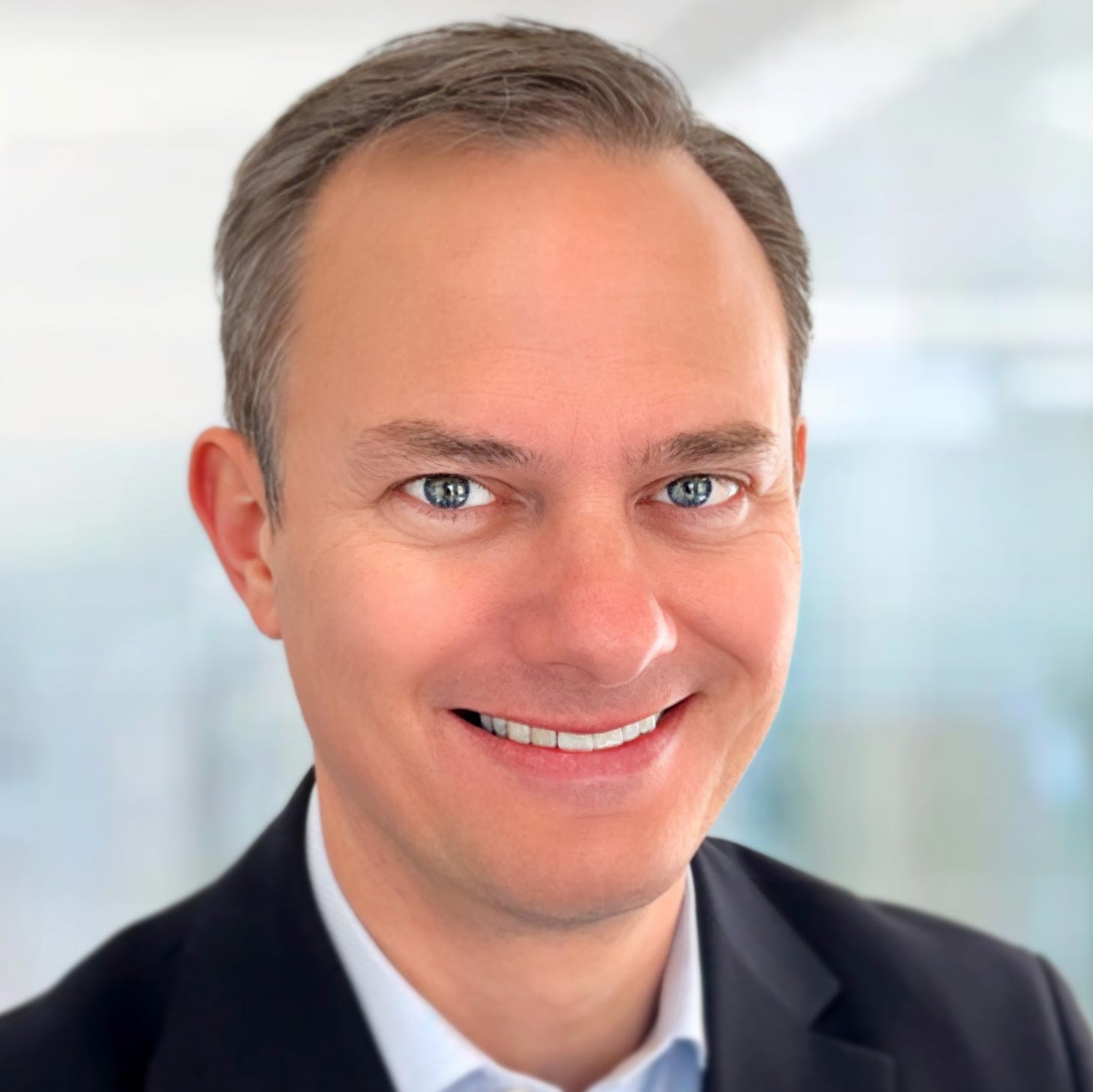

- AI is transforming modern business, but humans play a crucial role in safeguarding the integrity and ethical use of AI.
- Humans can refine AI by identifying potential bias, introducing new scenarios, and providing contextual relevance to AI-driven results.
- When humans and AI collaborate, organizations can drive better outcomes for their business.
Artificial intelligence (AI) is transforming modern business in ways we never thought possible just a few short years ago. But as new, AI-powered technologies make big promises, touting benefits such as increasing operational efficiencies, improving competitiveness, personalizing customer experiences, and boosting revenues, many businesses are realizing that technology alone is not enough to succeed in this AI-driven era. Organizations must also consider the role of humans in AI, including the valuable role that human expertise plays in safeguarding the integrity and reliability of AI and the ethical use of it to drive business outcomes.
The Role of Humans in AI
As AI tools and technologies integrate into modern business, it's important to understand the distinct role AI technology plays versus the role only humans can fill. While some doomsayers claim that AI can (and will) take over any task a human can perform, the reality is not quite that bleak.
Arguably, artificial intelligence is assuming responsibility for some of the work previously owned by people. But similar to how machines replaced manual labor during the Industrial Revolution, AI is replacing humans in areas where speed and efficiency matter. AI has the capacity to work faster than a human. And it doesn't tire because, well, it's a machine. That's why we're seeing AI take over straightforward, repetitive tasks that are time-consuming for humans. A perfect example is entity resolution.
Entity resolution is the process businesses use to integrate and match data across disparate systems and silos in order to create a "golden record" that represents the best version of a critical business entity such as a customer, supplier, product, or patient. Resolving entities across a company's ever-growing, ever-evolving data set would take a person, a team of people, or even traditional technology such as rules-based master data management (MDM) an inordinate amount of time. And every time the data changed or new data entered the systems, the process would reboot. But when AI is put to the task, entity resolution becomes exponentially more efficient. Using AI, organizations can employ advanced AI and machine learning-driven matching models to quickly and easily resolve the entities and create golden records that represent the best version of the data. And when data changes or new data is added to the system, the models can quickly and easily adapt, without the need to rewrite or redefine the rules. As a result, companies see results in weeks, not months or years.
In this example, AI assumes the heavy lift required to compare and resolve data sets. And as new data enters the system, either organically or through data enrichment, it can quickly add it to the mix and resolve it against existing entities as well. It replaces a human or outdated technology, but in the best possible way, making a repetitive, time-consuming task more efficient. But because AI only operates based on the data that trains it, AI alone simply isn't enough.
While AI can match and resolve entities based on what it's learned from the data that trained it, humans still have a role to play: fixing errors, making judgment calls on ambiguous cases, or providing additional context that the AI might not have considered. Involving humans in the process is crucial to ensure the highest level of accuracy and reliability, not only in the AI models but also in the golden records it produces. When paired with AI, humans spend less time on the tedious, rote tasks associated with entity resolution and more time adding value, context, and perspective through feedback, which, in turn, improves the integrity and trustworthiness of the golden records. Together, AI and human refinement combine the best of both worlds: AI's efficiency and scalability with human expertise, emotion, and empathy.
Why Collaboration Between Humans and AI is Critical
While artificial intelligence can and will transform business, the importance of human oversight and collaboration cannot be understated. Where AI's strengths lie in processing speeds and advanced algorithms, humans shine in the application of empathy, creativity, and contextual relevance.
Taking accountability for ethical decision-making and responsible use of AI is critical - and it's something businesses must prioritize. They must ask themselves: are we doing the right things as we adopt and use AI – and are we doing them in the right way? Promoting collaboration between humans and AI is a good place to start.
When humans are in the loop, they can help to expose potential biases, add context, and introduce new considerations that not only strengthen the integrity of the AI, but also hold organizations accountable for its ethical use. Without this human refinement, organizations run the risk of poor decisions or reputational harm.
Below are three risks organizations face when they fail to include human oversight as part of their AI process.
Exposing Potential Bias
Data powers AI. And when the data is bad, so, too, are the AI results. But bad data can lead to other consequences as well. When training data is incomplete, incorrect, out-of-date, or skewed, the results can be biased, leading users to make incorrect assumptions or biased decisions. Humans can help reduce the risk of bias by challenging the models when results appear incorrect or distorted and adding insight and context that the AI may not have considered.
Failing to consider new scenarios
Humans have the unique ability to imagine possibilities and create new scenarios based on their own distinct experiences. And because each human's experiences and make up are different, the scenarios they envision are distinct. AI, on the other hand, does not yet have the ability to consider situations and scenarios beyond what it is taught. Therefore, it may inadvertently exclude potential use cases that only a human could conceive or fail to consider new or different scenarios that exist outside the realm of its training data.
Ignoring contextual relevance
At face value, it's possible for AI to misinterpret data and return an invalid, inaccurate, or biased result. That's why it is critical for humans to take accountability and refine the results by adding context based on their experiences and their history. Without this valuable insight, data can be misconstrued and misrepresented, leading to faulty decisions or flawed outcomes. But when humans are involved, they can apply judgment and domain expertise to review and refine the AI by making judgment calls on ambiguous cases or providing additional, relevant context that the AI might not have considered.
Clearly, humans play a critical role in the refinement of AI-driven results. Without their feedback, AI runs the risk of overlooking relevant context, excluding critical scenarios, or worse, exposing bias. However, when humans and AI collaborate, organizations can increase the integrity of the results and drive better outcomes for their business.
To uncover more insights about innovations in data and AI, download our e-book entitled Data Master Perspectives: Innovations in Data and AI in 2024 and Beyond. In it, you'll find perspectives from leading thinkers on the future of AI as well as their views on topics such as ethics, transparency, and bias, the critical role of humans in AI, and much more.
Get a free, no-obligation 30-minute demo of Tamr.
Discover how our AI-native MDM solution can help you master your data with ease!