Is Your Data AI Ready?
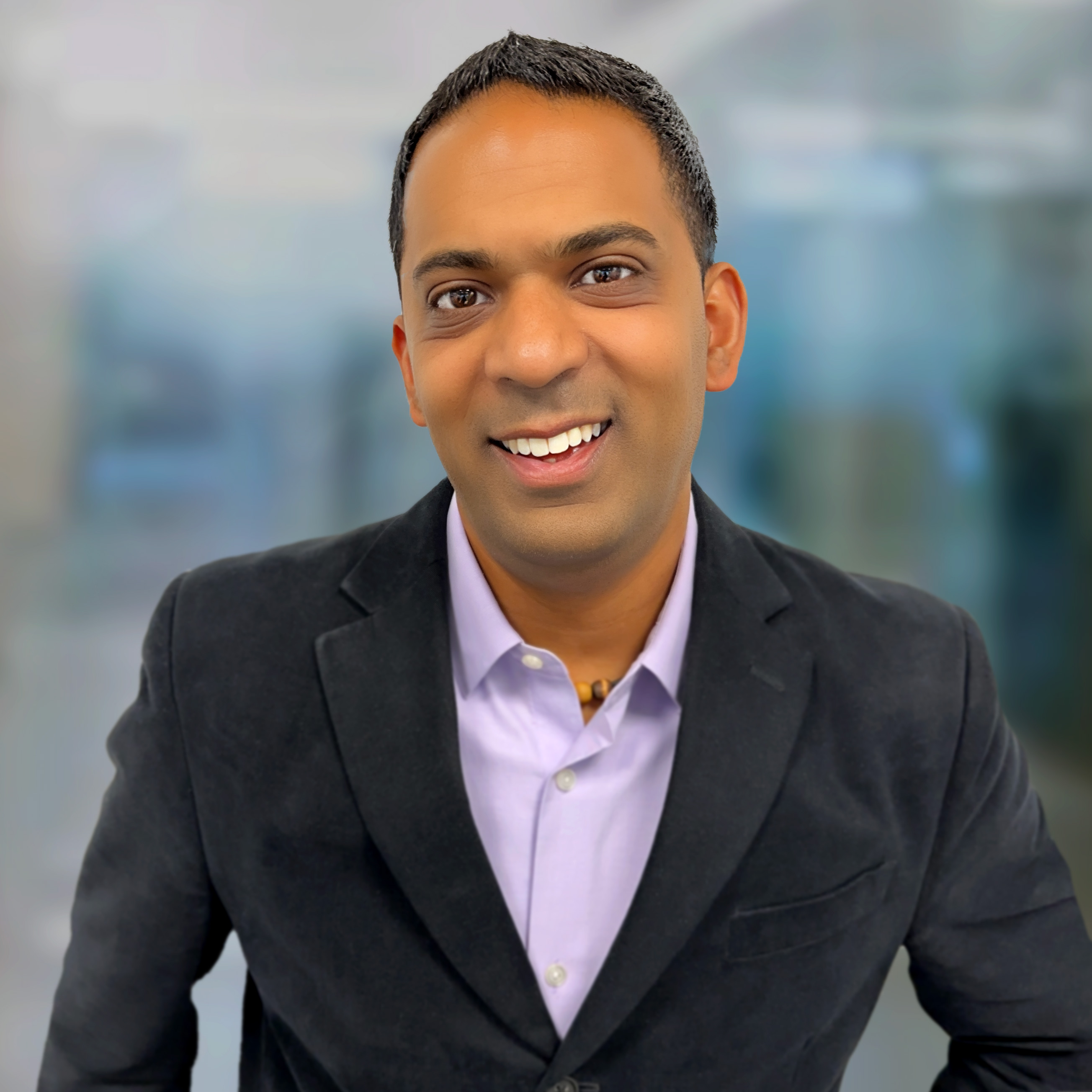

Summary:
- Organizations worldwide are grappling with what it means to fully embrace AI for decision making and are realizing they need to get their data AI-ready first.
- To make data AI-ready, organizations should focus on improving data quality, increasing data volume and variety, structuring data properly, and adding metadata and documentation.
- In turn, applying AI to data management can help organizations tackle the scale problem.
- AI has the potential to radically transform how CDOs, data teams, and analysts work in the future by enabling users to interact with data in real time and prioritize collaboration for delivering high-quality insights.
- Before jumping on the AI bandwagon, ensure your data is AI-ready by focusing on governance efforts and incorporating human feedback.
What does it mean for data to be AI-ready? It's a question that organizations worldwide are grappling with as the adoption of AI continues to surge. According to Wavestone's 2024 Data and AI Leadership Executive Survey, 64% believe Generative AI (GenAI) holds the potential to be one of the most transformative technologies in a generation, with 90% of organizations expecting to increase their investments in Generative AI.
To take full advantage of AI’s potential, businesses need more than just an abundance of data. They need data to be clean, organized, and accessible. That's why getting your data AI already involves more than just gathering information—it requires thoughtful data management, robust data governance, and alignment with AI technologies. Let's take a closer look.
4 Data Management Practices to Make Your Data AI-Ready
Making data AI-ready requires organizations to think about strong data management practices, implemented with intent. Below are four actions all organizations should take if they want to move towards AI-ready data.
- Improve data quality: While it may seem obvious, improving data quality is priority number one when it comes to preparing your data for use in AI. Failing to do so can lead to faulty insights and hallucinations resulting from inaccurate, incomplete, biased, or non-compliant data. By eliminating duplicates, removing inaccuracies, and filling in missing values, organizations can improve the integrity of their data and, in turn, the quality of the results AI produces.
- Embrace data volume and variety: Data is prolific. But the good news is that AI can handle very large volumes of data at scale - so let it do so! By feeding your AI models new, complex (high quality!) data, you can continue to train the models and help them to become more accurate.
- Creating data frameworks: Organizing both structured and unstructured data properly ensures that AI systems can use the data as intended. By applying consistent frameworks and organizing data, businesses can unlock deeper insights, enhance AI capabilities, and make more informed decisions.
- Add metadata and documentation: Defining metadata and maintaining thorough documentation improves data discoverability and usability. Together, they provide essential context, ensuring everyone can interpret the data’s quality, origin, and provenance. As well, metadata and documentation enable the AI models to process and generate reliable insights with minimal errors.
These practices should sound familiar. And the good news is, you may already have some of these in practice at your organization. So what's next?
Applying AI to Improve Data Management
Truth be told, all master data management styles benefit from the application of AI because the core challenge with mastering data is scale. Consider this scenario:
A business has 525,600 customer records, which equals the potential for 138 billion comparisons. This is known in computer science as the N-squared problem. Even if you have a fast computer and a good set of rules to bruteforce the comparison for 100 comparisons per second, it's going to take you 44 years.
This is where AI steps in to reframe the problem. Instead of brute-forcing all comparisons, AI uses techniques like bulking, clustering and pre-grouping to focus on the most relevant matches, significantly reducing the number of necessary evaluations. This way, regardless of which data mastering style you use, AI makes it easier for organizations to resolve entities across disparate systems and silos and create trustworthy golden records that everyone across the business can use to drive better decisions. It helps you tackle all of your data in a manageable way, focusing first on internal structured data and then moving on to unstructured data.
Additionally AI enhances data quality and coverage by distinguishing high-value information from irrelevant data — similar to how spam filters sift out unwanted emails. And, a robust AI solution will apply human feedback to continuously refine its understanding of what data matters most, improving overall data quality and reducing the noise that plagues many organizations.
However, when implementing AI, it's important to shift the mindset on data governance from source-based to consumption-based. Consumption-oriented data governance structures and manages data with the end-user in mind, ensuring it is easily accessible, understandable, and actionable. By aligning governance policies with how users actually consume data, organizations can improve data usability, drive better decision-making, and enhance overall data quality for use in AI applications.
The Impact of AI on CDOs of the Future
AI holds the potential to radically transform how Chief Data Officers (CDOs), data teams, and analysts work in the future. New, GenAI capabilities make it easy for all data users to interact with the data, ask questions about it, and quickly find answers in real time. It's like ChatGPT, but for your data.
Using intuitive, chat features, like those provided in Tamr's Virtual CDO, users can ask questions like "Who is the parent company for this customer - and how do you know?" In response, the system not only provides who the parent company is, but also the justification for how it knows this information to be true and the ground truth data it used.
With AI handling routine inquiries and automating data curation, CDOs and data teams can shift their focus from manual data cleansing to strategic collaboration with their business counterparts to deliver high-quality insights that move the business forward.
Clearly, AI is a game changer when it comes to realizing greater value from your data. But before you jump on the AI bandwagon, it's crucial to ensure that your data is AI-ready. By focusing governance efforts on consumption and providing human feedback, you will position your organization to take full advantage of the power AI has to offer.
To learn more about how Tamr uses AI to solve the scalability issue, please request a demo.
Get a free, no-obligation 30-minute demo of Tamr.
Discover how our AI-native MDM solution can help you master your data with ease!