How to Master Reference Data at Scale in Financial Services
I recently read an article by David Howes, the Global Co-Head of Financial Crime Compliance at Standard Chartered, where he aptly captures our mindset about data with the following statement: “Banks are data companies. The data helps you swim, but the wrong types can make you sink.”
This idea that data is a core asset for financial service companies is important when trying to understand reference data.
A decade or two ago, when we heard the term reference data, it simply meant data that provided additional descriptive content to our existing market data. Today, what we define as reference data is something much more. Reference data now encompasses an array of external data sources such as Refinitiv, Thomson Reuters, and LexisNexis. The sheer number of external reference data sources has grown exponentially.
Due to the rapid advancements in technology coupled with tighter regulations, we have access to a wealth of information right at our fingertips. Even typical market reference data offer an unprecedented level of detail from analyst opinions to news from third party sources.
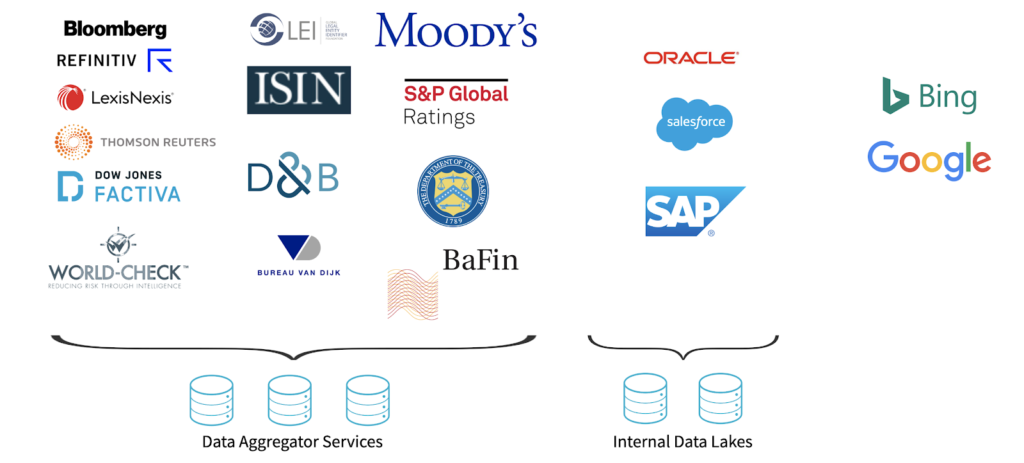
The evolution of reference data has made it an essential component in accelerating the digitization of your business and boosting operations cross-functionally. The Office of Foreign Assets Control (OFAC) publishes sanctions lists of individuals and companies acting on behalf of targeted countries. Financial institutions are under immense pressure to leverage their Customer Due Diligence (CDD) or Know Your Customer (KYC) program to identify customers sanctioned by these global governing bodies.
This is why financial organizations must make mastering customer reference data a priority in order to ensure the lines of defense for KYC and and Anti-Money Laundering (AML) can tackle manual processes and an abundance of dirty and duplicate data. With the intention of getting a handle on compliance and auditing issues, firms must adopt AI/ML solutions to significantly reduce the likelihood of having multi-billion-dollar fines being imposed against them.

From counterparty risks and vendor intelligence to customer 360 and sales analytics, reference data stitches together the fabric of your business comprised of every entity and boosts operational excellence. Conversely, common challenges around managing reference data include data silos across business units, resource-intensive onboarding process, and scaling up with reference data built around a specific MDM.
Today most enterprises still have dedicated teams working on this problem despite being originally designed years ago with a specific, rules-based, MDM in place. Consequently, the volume and variety of data result in an addition of thousands of rules that require teams of developers to deploy making scaling up a monumental effort. Therefore, teams tend to evaluate whether it is really worth it.
This dilemma creates a strong business case for a machine-augmented approach to managing reference data. Instead of juggling thousands of rules, human experts can train the model to pick up the grunt work while simply defining a handful of rules. The business efficiencies achieved from a human-guided machine learning approach can reduce the manual effort required from 200 full-time employees to less than 125 in preparing the data. As a result, this has the potential to generate more than $5 million in annual savings*.
Tamr offers a faster, more flexible, and better-optimized solution to master reference data at scale for financial institutions. Watch this on-demand webinar to learn how to maintain a 360-degree view of their customers, leverage machine learning, and implement a reference data management program built for scale.
* Assumes $75,000 FTE annual salary
Get a free, no-obligation 30-minute demo of Tamr.
Discover how our AI-native MDM solution can help you master your data with ease!