For Health Data Interoperability, Healthcare Organizations Need Machine Learning
Health data interoperability has been a goal in the US for decades. While the CMS ruling on data interoperability is definitely the right driver for industry-wide data standardization, data governance in healthcare will always face a variety of difficulties for reasons including:
- Data standards are always changing
- Different data vendors and systems conform to different data standards
- Varying and sometimes conflicting perspectives on how data should look like
- Data management and usage is siloed across departments and users
- Lack of data documentation
- Lack of data consistency
- And more…
However, traditional approaches to solving data management problems often rely on the efforts of a large team of data stewards who meticulously process; or aggressively prioritize and downsize data assets under management. With the explosion of tools now available to collect and organize data, being able to actually rationalize, analyze, and actively manage the organization’s data assets around enterprise standards is still very manual.
With pressure from regulations such as CMS interoperability in 2021, and the increase in data collection due to the global pandemic accelerating, there is a need for healthcare organizations to quickly and sustainably apply ongoing data standardization and mapping at scale. Taking traditional manual and rule-based methods to data curation will simply not meet the challenges of data variety, volume, and velocity.
Instead, a machine learning approach allows for the development of a customizable and evolving engine to accelerate and, in some cases, automate health data needs such as:
- Classification of metadata against Protected Health Information (PHI) and security policies
- Generation of cross-standard mappings for varying data element and value terminologies used by different users and systems
- Standardization of data terminologies used across the enterprise
Configure Machine Learning to Master Data Against PHI and Security Classifications
The most obvious data classification problem in healthcare may be the identification of Protected Health Information (PHI) information. PHI is defined under the Health Insurance Portability and Accountability Act (HIPPA) as any health information that can be tied to an individual, and includes over 18 identifying data elements. Many existing data governance softwares which purport to identify PHI “by machine learning” actually rely on a variety of rules and known key-terms in order to identify these sensitive data elements. Not only is this misleading, this type of automation is limiting; what if you have data in multiple languages, or need to expand the scope of what PHI means to your organization? What if there are PHI indicators and variations that have not been captured by the machine’s pre-configured learning? What if you need a completely new data security category for your organization?
With Tamr’s human-guided machine learning, customizable machine-learning classification engines can be generated to interrogate your data for PHI, PII, or any other security categorizations specific to your organization. This is thanks to Tamr’s award-winning interface to collect active feedback from subject matter experts on what “the experts” believe the data should look like, not some preconceived configuration.
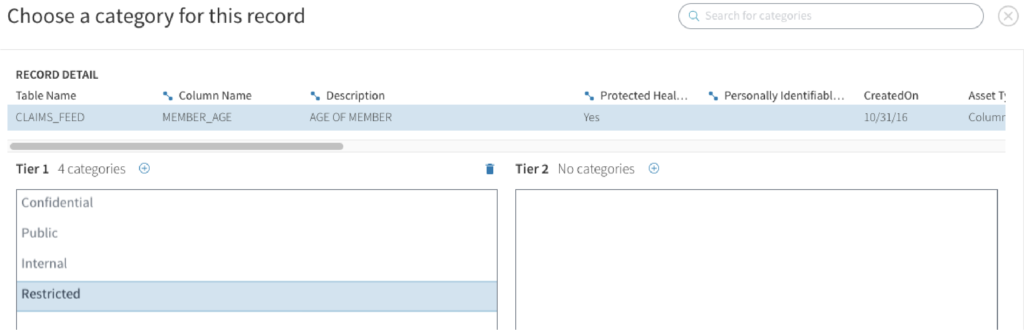
Tamr’s data classification engine allows you to build custom machine learning models against any taxonomy you need. Whether it’s grouping together metadata as PHI / non-PHI, or applying more nuanced security policies, Tamr’s UI/UX allows subject matter experts to easily build their knowledge into an ML model.
With this approach, the machine may start with basic knowledge of PII and PHI, but will “become experts” over time as the organization’s experts train it to their view of the world in data governance.
Configure Machine Learning to Master Data Against FHIR Mappings
Training a machine to automate the identification and mapping of data for governing data security also applies to governing data standards more broadly. In particular, as health data is moving towards the HL7 FHIR standards, the application of Tamr’s machine learning to quickly identify linkages across different data standard versions, discrepancies, and similarities in order to generate cross-walks for both data schema and elements, as well as data dictionaries and value sets.
The capability to initiate and maintain mappings across standards places machine learning as a critical piece of any future terminology service as FHIR helps the healthcare industry accelerate its data interoperability capabilities.
Below are examples of just some of the many ways machine learning can accelerate data discovery, standardization, and mappings.
Discover the appropriate elements and values to map into – Machine learning can support the linkage of similar data schema elements and data values. Linkages across these metadata elements allow for the analysis and understanding of what and where data exists. Not only can Tamr link similar data elements across disparate datasets, but when matched against data standard specifications such as FHIR, machine learning greatly accelerates health data interoperability initiatives. Identified by Tamr, these linkages can further be managed within the workflows of existing governance tools such as Collibra or Alation, further boosting the value and coverage of such tools.
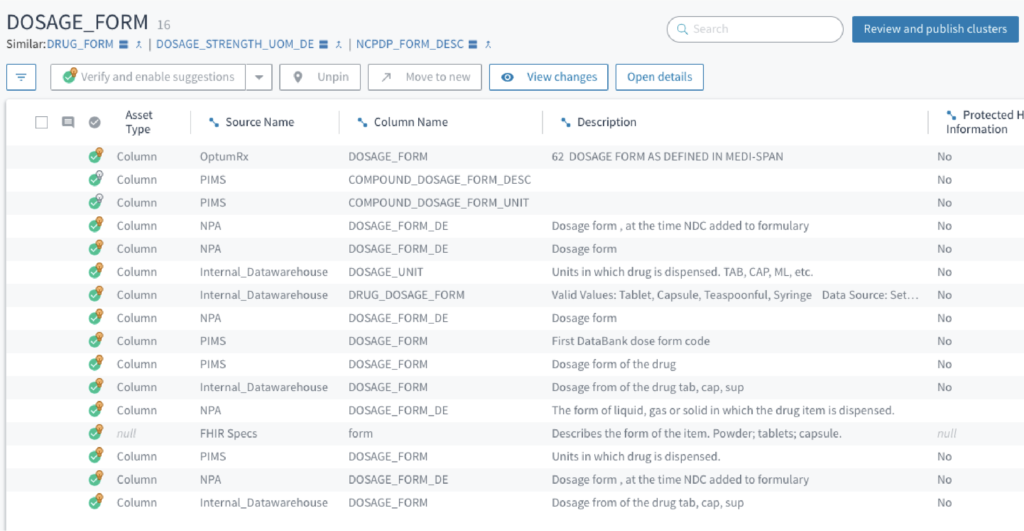
Tamr machine learning links together similar terminologies used across different data sources and standards. Within Tamr’s UI/UX, subject matter experts most familiar with the data can supply their knowledge of mapping relationships into a machine learning model to accelerate the generation and maintenance of terminology mappings across the enterprise. Once mappings are available, enforcing standardized terminology becomes much easier.
Standardization of the elements and values for ongoing enterprise use – Once mappings for data elements and values across disparate datasets (and/or standards) have been identified with the help of machine learning, the implementation of logic to determine preferred terminologies becomes much easier. Moreover, the generation of terminology crosswalks enables individual users to seamlessly view their data within their standard context whether there is a preferred data source (such as FHIR standard specifications), or a particular rule logic for that business unit.
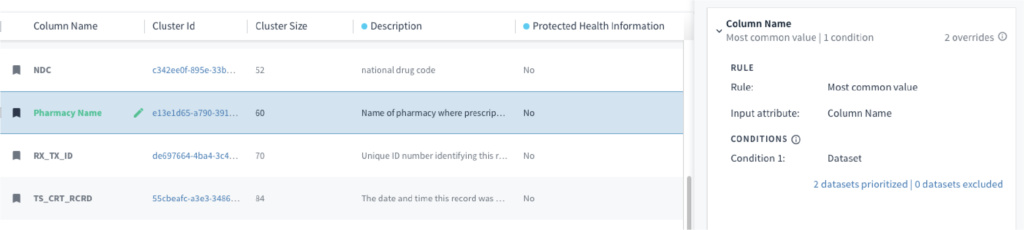
Conclusion: Machine learning is necessary for the future of managing health data
Tamr’s machine learning approach to mastering and classification significantly accelerates healthcare organization initiatives to better manage their data standards on an ongoing basis. The traditional approach of manually mapping data elements and terminologies across different data sources and standards over time is not cost-efficient nor scalable – leading ongoing struggle for the industry to achieve health data interoperability.Tamr has been successfully implemented across the world’s top life science and managed health organizations. We have seen a large variety of digital transformation initiatives and ecosystems. We’d love to offer you a workshop to explore how to modernize your approach to health data interoperability with machine learning.
Get a free, no-obligation 30-minute demo of Tamr.
Discover how our AI-native MDM solution can help you master your data with ease!