Google Reiterates Just How Critical Data Is For High-Stakes AI
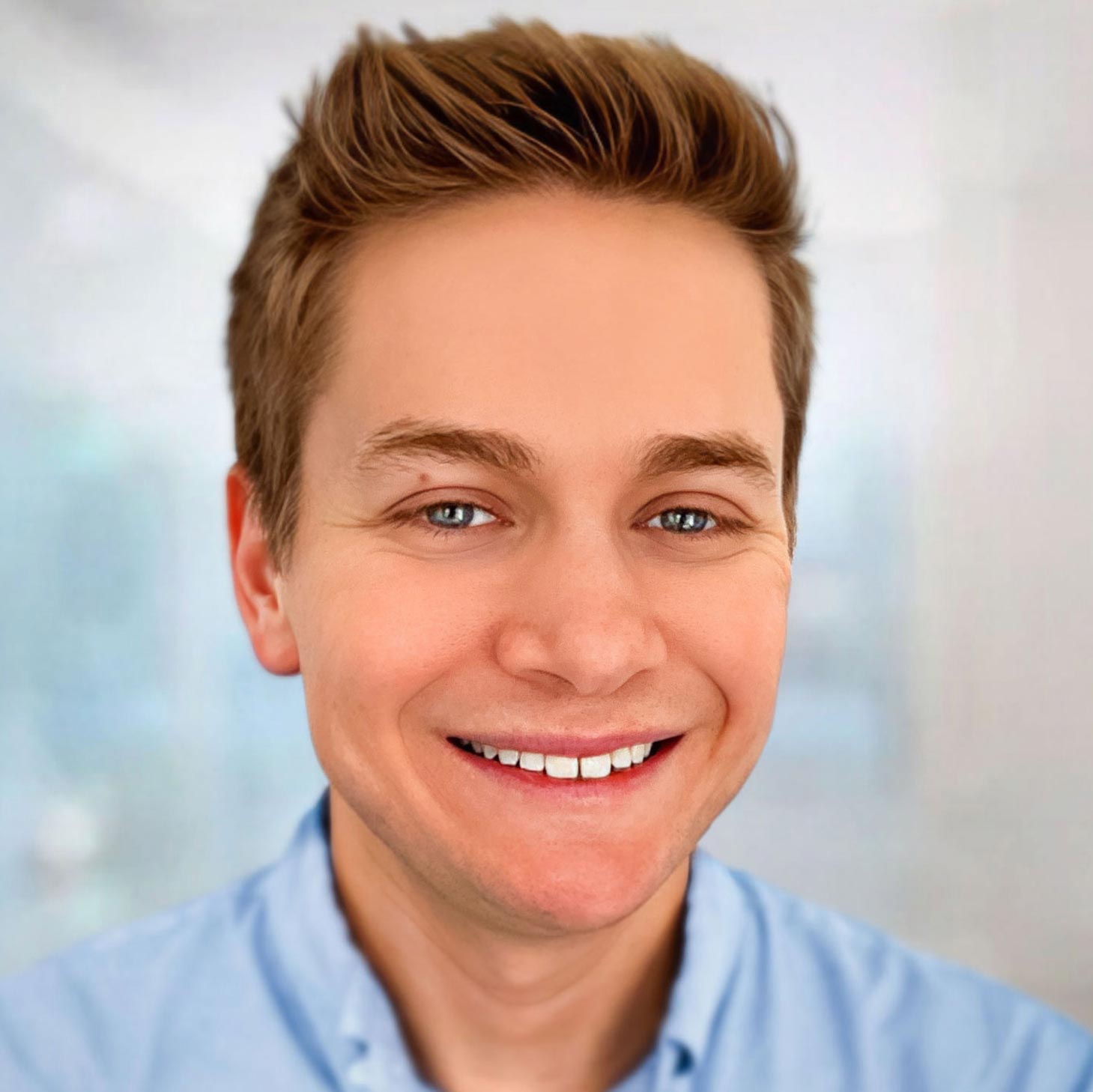
Summary
- Data Cascades—compounding events causing negative, downstream effects from data issues—triggered by conventional AI/ML practices that undervalue data quality.
- High-stakes applications with downstream human costs are most vulnerable to data cascades and face the most dire consequences from poor data quality
- Conventional AI/ML practices that undervalue data quality are at fault
- Secular and operational changes required
- Modern data mastering can help improve data quality and fix persistent data problems
Cascades can be a good thing. Who wouldn’t want a cascade of money or bitcoin? But the data that’s powering your AI models? Not so much–particularly when that data is being applied to high-stakes problems in areas like health care and conservation.
That was the conclusion of a recent, aptly named paper from Google Research, “Everyone wants to do the model work, not the data work”: Data Cascades in High-Stakes AI.”
The researchers document the very real problem of what can happen when data for AI models is ill-prepared or guided, along with the lack of a viable, real-world process for generating good data for AI. The researchers warn: “Data quality carries an elevated significance in high-stakes AI due to its heightened downstream impact.”
Data cascades are “compounding events that create negative, downstream effects from data issues triggered by conventional AI/ML practices that undervalue data quality. Such data cascades can result in technical debt over time and potentially high human costs.” Poor data practices, for example, reduced accuracy in IBM’s cancer treatment AI and led to Google Flu Trends missing the flu peak by 140%.
Based on an analysis of real-life data practices in high-stakes AI, the researchers showed data cascades as pervasive (92% prevalence), invisible, delayed, but often avoidable. Their prescription? Designing and incentivizing data excellence as a first-class citizen of AI, resulting in safer and more robust systems for all.
Despite these problems, we have readily available solutions – both in terms of data management strategies and modern technologies – that can solve these problems at scale.
Implications for Mainstream-Business AI Models
AI is fast-becoming table stakes for businesses, governments, nonprofits, and NGOs. For CDOs, this makes lowering the risk of ill-informed, dysfunctional and/or unscalable AI models a priority. However, the fix isn’t better models or hiring more PhDs; rather it’s the less glamourous work improving the underlying data that needs their attention.
An analogy can be made to what’s happened in the weighting of importance between advancement in software vs. hardware. For a long time, the conventional wisdom reasoned that technology could only advance as quickly as the hardware would allow. But with Moore’s Law slowing (and the emergence of cloud computing), it’s now the software governing the pace of change.
This is similar in AI. Historically, AI was limited by the models underpinning it; in turn, leading to greater investment in the technology and people (data scientists) who solved this. The data feeding AI models were largely an afterthought given the models could only consume so much data. But as Google highlights it’s now data — specifically the quality of the data — that limits AI’s effectiveness.
With AI initiatives being a focus, here are three steps you should be taking today to mitigate data cascades.
Reward Human Involvement in Data Quality
AI model development is the new “It” tech profession. Meanwhile, data management and preparation is considered grunt work and a thankless task. This thinking is rooted in the problems with today’s AI. As the Google researchers noted: “Data largely determines performance, fairness, robustness, safety, and scalability of AI systems…[yet] In practice, most organizations fail to create or meet any data quality standards, from under-valuing data work vis-a-vis model development.”
They remind us that beyond data scientists, there are many crucial roles in the process of preparing, curating, and nurturing data, which are often under-paid and over-utilized. And the costs of poor on inefficient data practices can add up: for example, the researchers estimate that data labeling can account for an estimated 25%-60% of the costs of ML.
AI model developers lack the skills and context for ensuring quality data. Because they depend critically on good data, effective AI models require more human involvement–from both official data workers (database engineers, data curators) and from people for whom data collection and expertise isn’t their day jobs but a chore (nurses, forest rangers, oil field workers and business experts intimately familiar with their data).
This is no different than the consumer web. Why are the store hours on your favorite bagel shop accurate on Google? Because the shop owner cares a lot about the quality of that data, and curates it, just as Google intends.
As Google has shown, magic happens when you can automate challenging, but also mundane, tasks core to improving data quality, such as data mastering, while making it easier for humans to contribute their expertise by engaging them elegantly in data-quality decisions (which, as domain experts, they need to do anyway.)
For example, in enterprise data mastering, you can use ML to automate routine data-quality decisions (~90%), letting the ML do the obvious record-matching or involving humans only when necessary to answer the gnarly exceptions (~10%). Reward their participation by making it painless and quick for them to answer data-quality questions (e.g., via a simple yes-no question delivered via email) and by distributing questions correctly and equitably. In other words: value and optimize their time and effort. We’ve seen many Tamr customers use rewards programs on top of these automation initiatives to encourage contributions to their enterprise’s data asset, not dissimilar from the type of rewards that sites like Yelp give to encourage contributions to the commons.
The Effects of Real Data vs. Prototype Data
In the interest of time and lack of readily identifiable data, AI-model creators too often don’t use real data, settling for “good enough” data. Without real data, however, even the most beautiful AI model risks falling apart under real-world working conditions. Prototype conditions almost never match real-world conditions, resulting in AI models that just can’t scale. Further, how data work is often invisibilized through a focus on rules, arguing that empirical challenges render invisible the efforts to make algorithms work on data, the researchers noted. “This makes it difficult to account for the situated and creative decisions made by data analysts and leave a stripped-down notion of ‘data analytics’” they said.
When you solve the data-quality-flow problem by involving the right humans in the right amount at the right time (see above) to create repeatable master enterprise data models, you’ll begin to solve this problem. However, for the best results, you really need to do this systemically..(Read on.)
Embrace DataOps
Google’s research results indicated “the sobering prevalence of messy, protracted, and opaque data cascades even in domains where practitioners were attuned to the importance of data quality.”
By embracing DataOps principles, you’ll shift your MO from data exhaustion (reactive, repetitive, labor-intensive data handling) to data excellence (proactive, human-guided data automation, repeatable and updatable mastered data models), creating responsive data pipelines to feed your AI models. As the Google researchers suggest, consider proactively training the model data instead of letting it train itself unattended. By using your new data-quality engine and human-informed process, you can let a human intervene and correct data quality problems without disrupting your AI model timetable, for example.
Next Steps
Large organizations like the U.S. Air Force, are pushing the envelope on AI/ML applications for mission-critical activities, and are using these techniques successfully today in getting high-stakes, high-profile projects to work.

To hear from them directly, check out the DataMasters podcast or the on-demand version of our inaugural DataMasters Summit.
The Google paper is an excellent, must-read for CDOs, CTOs and others responsible for data assetization and AI model deployment. (You can download it here.)
Get a free, no-obligation 30-minute demo of Tamr.
Discover how our AI-native MDM solution can help you master your data with ease!