Customer Data Integration: Since When Is “Fuzzy” Better Than Exact?
Since companies started drowning in more data than they could reasonably manage through tidy, top-down schemas and custom cleaning code. That’s when.
Data source proliferation — a natural result of business growth (especially M&A), idiosyncratic software systems and exploding big data investment – continues to amplify the problems of data volume, variety and cleanliness in an enterprise. There is simply too much dirty data, too many types of data, and too many data sources. Traditional management and cleaning methods can’t handle them quickly enough to matter. The cost of data variety is only fully appreciated when companies attempt to ask simple questions across many business silos – be they divisions, geographies, applications or functions.
Take the case of a global financial services company – a current Tamr customer. One of its divisions serving high-net-worth individuals has 400 relationship managers who enter data into a legacy client on-boarding application. With so many relationship managers entering client information over time, the data has become increasingly dirty, beset with duplicate records, missing fields and erroneous values.
The company was looking to remedy these problems and unify client records in order to proceed with a migration to a new on-boarding application. They simply didn’t want to put dirty, fragmented data into a powerful new application.
So they did what a lot of companies naturally do: get an internal IT team to develop code customized to their record structure, with rules identifying and de-duping exact matches on customer name, address, date of birth, etc. Problem is, exact, rule-based matching can’t fully account for the messy reality of disparate, dirty data — and can’t keep up with future format variations such as naming conventions. Doubting that custom coding was the most efficient solution, the company invited Tamr to go “head to head” with its internal approach.
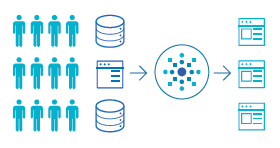
Read this case study for a full accounting of what and how Tamr did in this evaluation. But given a sample of the raw data set, Tamr’s “fuzzy,” probabilistic matching system (with only 2-3 hours of training) accurately matched 16 records to a particular customer versus the 2 records surfaced by the company’s rule-based approach.
Based on these results, the company quickly adopted Tamr for a data enrichment and unification project across all 400 relationship managers that prepared clean, unified records for the new on-boarding application (and other downstream systems).
The implication for this and other similar enrichment and unification use cases? Well, “clean fuel” for the expensive analytics engines that enterprises are investing in, for sure. But at a higher level, Tamr elevates the collective trust that people in the enterprise have for the data, the applications using it, and ultimately, the decisions they’re making as a truly data-driven organization.
Get a free, no-obligation 30-minute demo of Tamr.
Discover how our AI-native MDM solution can help you master your data with ease!