How to Bring Intelligence to Taxonomies
Why are organic foods given their own aisles of the grocery store? Unlike other sections, “organic food” isn’t very descriptive; the classification can apply to anything from a banana to baby food. But customers have shown with their wallets that this distinction is meaningful, and the market has responded.
This type of response to market demand is rarely seen in how enterprises classify their data. The needs of data consumers are constantly evolving, but companies are slow to adapt, as they rarely have tools or processes for monitoring these changes and taking action on them. If a company transitions from manufacturing engines to selling software, it is instantly more interested in the types of servers it’s buying than pistons. In turn, the internal taxonomies need to evolve to provide this level of granularity — rarely a quick or easy task.
The primary challenge with building or evolving a taxonomy is getting alignment without having data. One person may want servers to be classified based on their physical dimensions, while another may want the classification to reflect the performance of the server. Each person can have a great argument, but it’s hard to prove whose way is better. We at Tamr believe the only way to break this cycle is by introducing data into the equation.
One of our customers, a $21bn+ industrial firm with over 12 business units, recently kicked off a project to classify all of its purchase data into a global taxonomy. But first, our customer leads wanted to answer two questions:
How do we update our taxonomy so that it reflects how we do business today?
If a transaction doesn’t fit into a category, should we create a new category or label it as “other”?
Rather than put the project on hold until these questions could be resolved, we decided to turn our platform on its head. Typically, Tamr is learning from user feedback to make inferences about how data should be classified. Experts provide examples of classified records, and Tamr identifies the keywords that are most relevant to data in that category.
Developing or modifying a taxonomy requires the opposite approach: understand the keywords within the data and then build categories aligned with what’s actually in the data. We think of this as creating an “intelligent taxonomy” — a taxonomy that is aware of the context under which it is being applied.
For our customer, this meant adding ten new categories to the “electrical components” category to align with product categories, such as Schottky diodes, that had become more significant portions of their spend. Since these recommendations were data driven, it only took one meeting to gain support from business stakeholders.
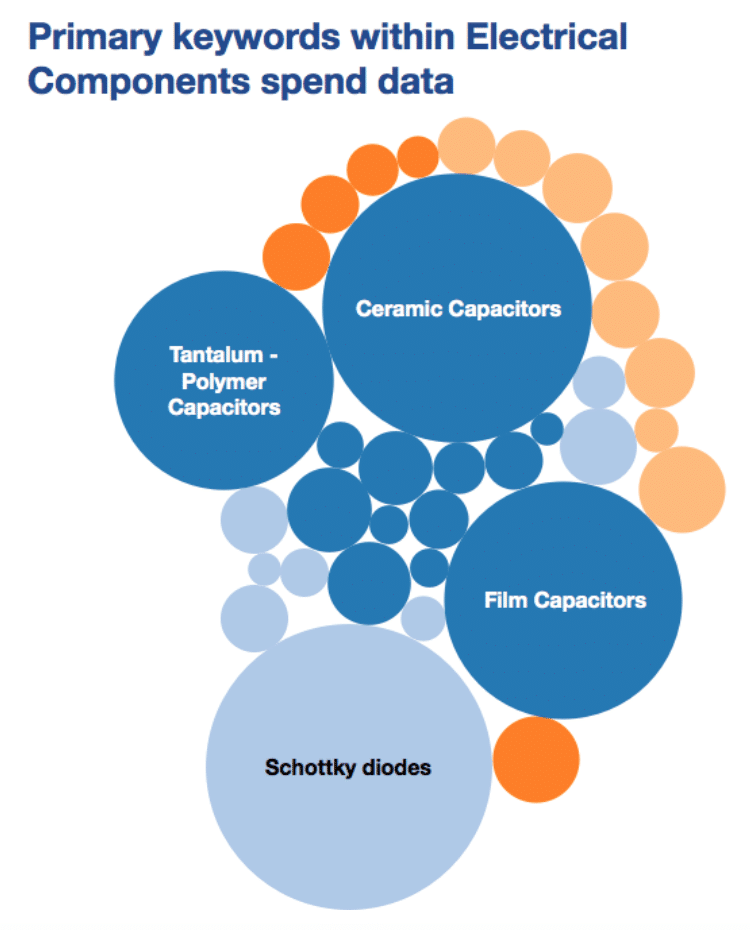
Clustering reveals component types that are actually relevant to the business
Our customer was also able to use this approach to understand the keywords most commonly classified as “other” within their legacy taxonomies. This revealed that items were typically classified as “other” because the taxonomy did not have a good category for the data, rather than the assumption that employees were using the category in haste. Identifying new categories to add to the taxonomy — and eliminating “other” — means that procurement can start to negotiate better pricing on this spend, which is worth $10M+ in cost savings for this company.
This effort has given our customer a taxonomy that represents what it buys globally today, but what it buys will never stop changing. We have embraced this problem by introducing full taxonomy management into our product. We’re giving customers the ability to add, eliminate, and modify categories within their taxonomy so their data is consistently organized in a way that’s optimized for gaining visibility into their business and finding new opportunities.
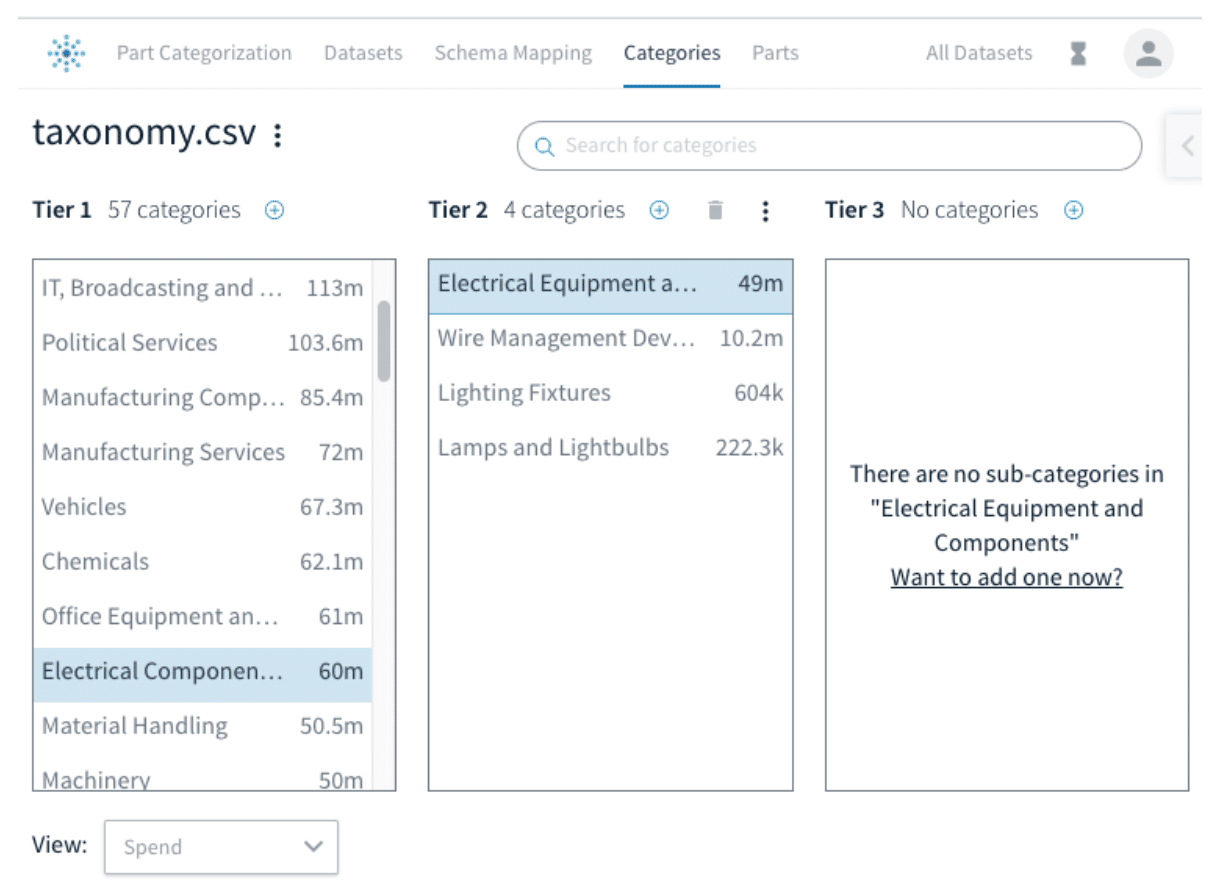
Additional granularity can be added as spend gets managed at lower levels of detail
By eliminating the hurdles associated with developing or modifying a taxonomy, we want to enable more organizations to organize their data in a way that aligns with the actual contents of the data and how end users consume the data. If grocery stores are constantly reorganizing their aisles to simplify how customers find what they want, why shouldn’t the enterprise do that with data?