AI Failure: 7 Blunders to Avoid in 2025
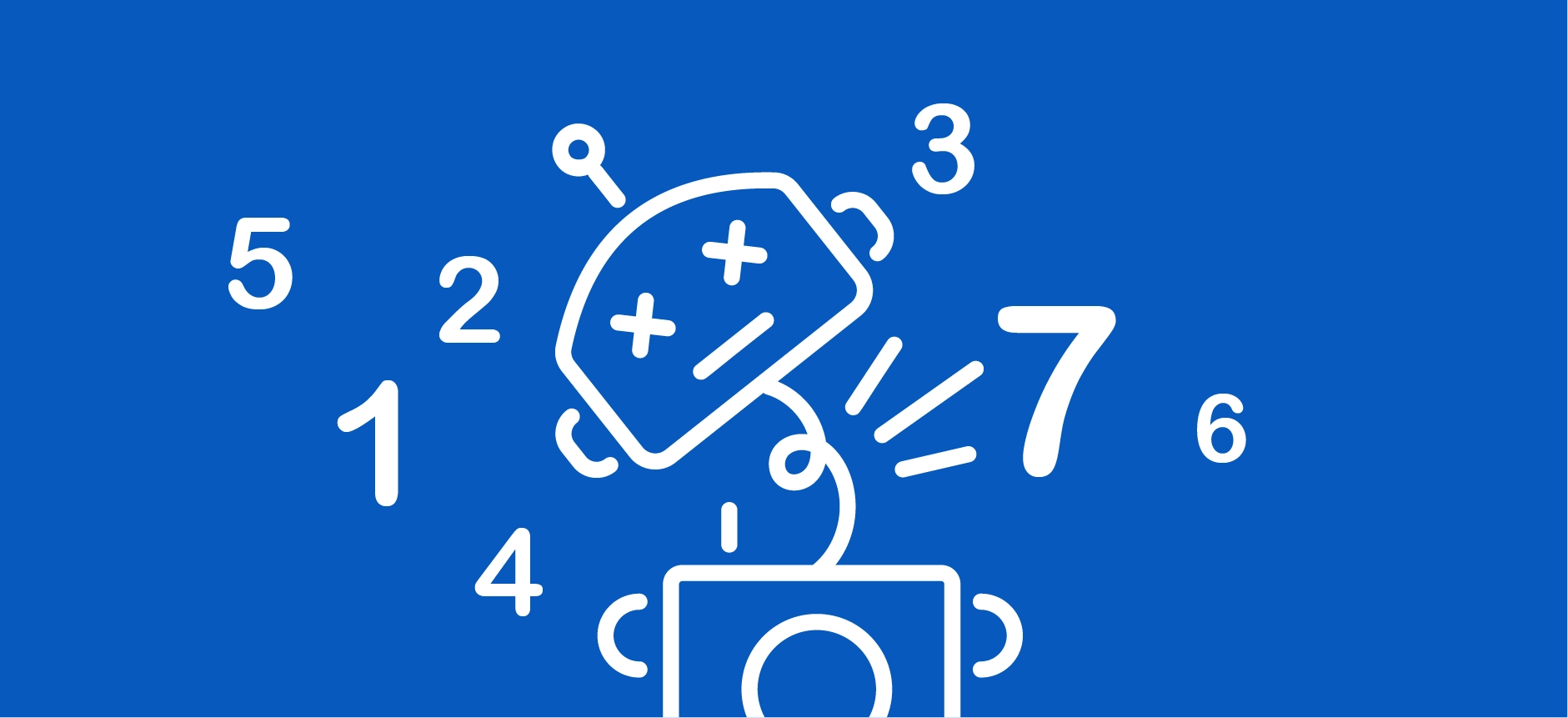
We’ve all heard the adage “disrupt or be disrupted.” And when it comes to artificial intelligence (AI), nothing rings more true. However, as the pressure to embrace AI increases, many companies are falling prey to common pitfalls on the road to AI nirvana. From underestimating the quality of data they need to power AI applications to costly mistakes that erode trust or worse, tarnish brand reputation, these missteps can be both a drain on resources and a setback to achieving strategic goals.
As we head into 2025, AI’s influence is only going to increase, putting pressure on businesses to implement these technologies quickly. But hasty adoption could lead to costly mistakes, especially if the initiative isn’t aligned with the company’s strategic goals. That’s why it’s crucial to recognize common blunders and understand how to avoid them before they cause disruption to your business.
Taking some inspiration from Tamr's former Chief Product Officer’s presentation at the 2024 Big Data London conference (available here), here are the 7 AI Blunders to Avoid in 2025:
1. Doing “AI” initiatives, not “business” initiatives
AI is a powerful tool. But many people become too enamored with the tool itself, rather than what it can enable for their business. That’s why organizations who embark on AI initiatives often fail to realize the full potential and value that AI has to offer. Because their focus is on implementing the latest whiz-bang technologies, their efforts become siloed, lacking the context and internal support needed to drive measurable impact.
Instead, organizations who succeed with AI align it within strategic business priorities. By integrating AI into these initiatives, AI has direction and purpose, elevating its role from a powerful tool to a critical piece of the business strategy. Further, when AI aligns with business objectives, it promotes greater adoption which, in turn, maximizes the overall value of AI-driven insights.
2. Believing a foundation model will solve everything
Foundation models (i.e., LLMs) offer numerous benefits when it comes to building Generative AI (GenAI) solutions. Not only are they efficient and versatile, but they also are adaptable and accelerate the development of AI-powered solutions.
However, if your organization believes that a foundation model will solve all of your business problems, you’re wrong. Too often, data teams are blinded by the promise of foundation models and move full steam ahead with the building of a GenAI solution. However, in doing so, they often fail to consider key questions such as:
- Does the data you need to train the foundation model live within your four walls?
- Are LLMs the best solution for all your challenges?
- Do we have the right resources to help with this effort?
- Can a new team maintain what gets built?
To avoid this AI error, don’t place all responsibility with the AI engineering heroes. And don’t disregard other approaches, such as classic ML techniques. Instead, assemble a cross-functional team with representatives from the data team and the business who can help answer the questions above. And let these answers guide your strategy, especially when it comes to decisions related to build vs. buy. Not only will this collaborative approach help your organization ensure it’s taking everyone’s needs into consideration, but it will also help you to be smart about how you approach GenAI solutions.
3. Forgetting that machines need love (feedback), too
It’s a well-known fact that AI/ML models need data to train them. But in addition to high-quality data, it’s also important to incorporate human feedback. Human expertise provides a layer of oversight and refinement that complements the AI’s capabilities, ensuring that the data is accurate and trustworthy. Without it, you run the risk of introducing inaccuracies, misrepresentations, or bias.
Too often, however, companies view human feedback as a “one and done” activity. This is a mistake. To ensure AI models improve over time, it’s critical to embed human feedback into your everyday workflows. Using consumption as your guide, make it easy for users to give AI results a thumbs-up or thumbs-down to indicate the quality of the output. Using this critical input, organizations can then determine which results require further refinement and training.
4. Neglecting the end-user experience
End users are a critical piece of the development process, providing input into the context, needs, and real-world challenges that guide the user experience. This is especially important with new technologies like AI where UX best practices are changing quickly. When organizations fail to involve users, the design process results in disconnected, frustrating user experiences that are difficult to adopt. As a result, adoption suffers because users are not bought-in to the value the solution provides.
A simple way to avoid these issues is to involve end users right from the start. By engaging with end users early and often, your organization can gain valuable feedback on the features and capabilities that matter – as well as the ones that don’t. Incorporating this input into the development process increases the likelihood that the end result is both intuitive and functional for the task at hand. Further, involving users builds trust and promotes a sense of ownership, both of which are critical when it comes to the successful introduction and adoption of new technologies such as AI.
5. Making data quality a project, not a process
Poor data quality is a pervasive problem for businesses worldwide. And as we head into 2025, it’s likely this problem will persist.
Duplicate, inaccurate, incomplete, and outdated data accumulates across systems and silos, making it difficult to identify and correct. Left unchecked, dirty data multiplies over time, causing a ripple effect within organizations that disrupts business processes and leads to misguided, ill-informed, or faulty decisions. Many organizations initiate projects aimed at cleaning up their bad data, and while well-intentioned, these one-time efforts don’t provide a long-term fix for the problem. And as companies continue to accelerate their adoption of AI, the importance of high-quality data becomes even more acute.
To overcome the dirty data challenge once and for all, it’s imperative that organizations establish strong practices and procedures that avoid the continuous cycle of correcting and re-correcting inaccurate data. By implementing real-time data mastering using AI-native MDM, organizations can validate newly-created data and identify potential duplicates while the data is still in motion, preventing erroneous, poor-quality data from entering systems in the first place. As a result, businesses can deliver the clean, accurate, continuously updated golden records needed to drive business outcomes.
6. Succumbing to the “Innovator’s Dilemma”
In his well-known book The Innovator’s Dilemma, Harvard Business School professor Clayton Christensen introduces a challenge that successful companies face when disruptive innovations emerge: prioritizing the refinement and advancement of current products over experimenting with or adopting newer, more disruptive innovations. Oftentimes, well-established businesses opt for the former, causing them to fall behind their forward-thinking competitors.
Christensen’s concept translates to data management. For years, many companies have invested in traditional, rules-based master data management (MDM) solutions that aim to improve data quality and deliver golden records. However, as data has grown in both size and complexity, they’ve failed to adopt newer, more advanced ways of mastering data, such as AI-native MDM. Their failure to embrace a more modern way of mastering data and delivering golden records has held them back, making it difficult to master their data at scale.
As we head into 2025, experimenting with and adopting new, AI-powered technologies is more important than ever before. AI offers incredible potential to personalize customer experiences, improve operational efficiencies, and uncover new opportunities to advance the business. By taking an experimental approach, organizations can begin to test how their organization can harness the potential of AI to drive business outcomes while also minimizing risk. They can gain insight into ethical considerations, address data privacy concerns, and understand the nuances of using AI to drive decisions across the business. By giving these experiments room to breathe, the business can test-drive AI-powered applications and experience, first-hand, how AI can help them reach - or exceed! - their business goals.
7. Waiting for someone else to take action
When it comes to adopting new technology such as AI, taking action early can be the difference between remaining a leader in your industry and falling behind. But many times, companies fail to take decisive steps or prioritize the enhancement of existing systems (see Blunder #6!), leaving them struggling to catch up as others in their industry move full steam ahead.
Technology, especially AI, is evolving rapidly. And assuming that someone else in the organization will act first could be a costly mistake. Instead, take a bias toward action. Doing so will enable your company to foster a culture of innovation that incorporates AI technologies into existing business processes in a way that is safe and secure. By taking action, you’ll gain firsthand insights into how the technology works as well as its benefits and limitations in the context of a specific use case. This approach also enables your organization to identify potential issues and adjust prior to a large-scale rollout, which reduces the risk of costly mistakes.
As we head into 2025, it’s essential to approach the adoption of AI with clarity and a strategic plan, as well as an awareness of potential missteps you may encounter along the way. Avoiding common AI errors—such as failure to align AI initiatives with business strategies, overlooking end-user needs, or underestimating the importance of data quality—helps to ensure that AI will act as an enabler for your business, not a hindrance to its success. By taking a thoughtful approach to AI in the new year, your organization will build a resilient, scalable approach to AI adoption that sets the stage for long-term business success.
Get a free, no-obligation 30-minute demo of Tamr.
Discover how our AI-native MDM solution can help you master your data with ease!